CREAM: Computing in ReRAM-Assisted Energy- and Area-Efficient SRAM for Reliable Neural Network Acceleration
IEEE TRANSACTIONS ON CIRCUITS AND SYSTEMS I-REGULAR PAPERS(2023)
摘要
SRAM-based computing-in-memory (CIM) has been widely explored to accelerate neural networks (NNs). However, it is challenging to store all weights of many modern NNs due to limited on-chip SRAM capacity. This bottleneck induces a large amount of off-chip DRAM accesses and impedes the improvement of performance and energy efficiency. This paper proposes a new approach of computing in resistive random-access memory (ReRAM)-assisted energy- and area-efficient SRAM (CREAM) for accelerating large-scale NNs while eliminating the DRAM access. The NN weights are all stored in high-density on-chip ReRAMs and restored to the proposed non-volatile SRAM (nvSRAM) CIM cells with array-level parallelism. Furthermore, to deal with the influence of ReRAM and CMOS variations, a novel layer-wise and bit-wise weight-configuration search algorithm is proposed by leveraging different sensitivity of each layer in NN models. A data-aware weight-mapping method is also presented to efficiently map NN models to ReRAMs in CREAM for high computation parallelism. The experiment results show 10.3x weight storage density over the standard 6T SRAM array. Evaluations of ResNet-18 and VGG-9 on CIFAR-10/CIFAR-100 datasets show up to 3.47x and 1.70x energy efficiency over two baseline designs of SRAM-CIM and ReRAM-CIM, respectively, in addition to 15.6% higher accuracy than ReRAM-CIM under device variations.
更多查看译文
关键词
ReRAM-assisted SRAM,compute-in-memory,neural network,high density,restore yield,weight mapping
AI 理解论文
溯源树
样例
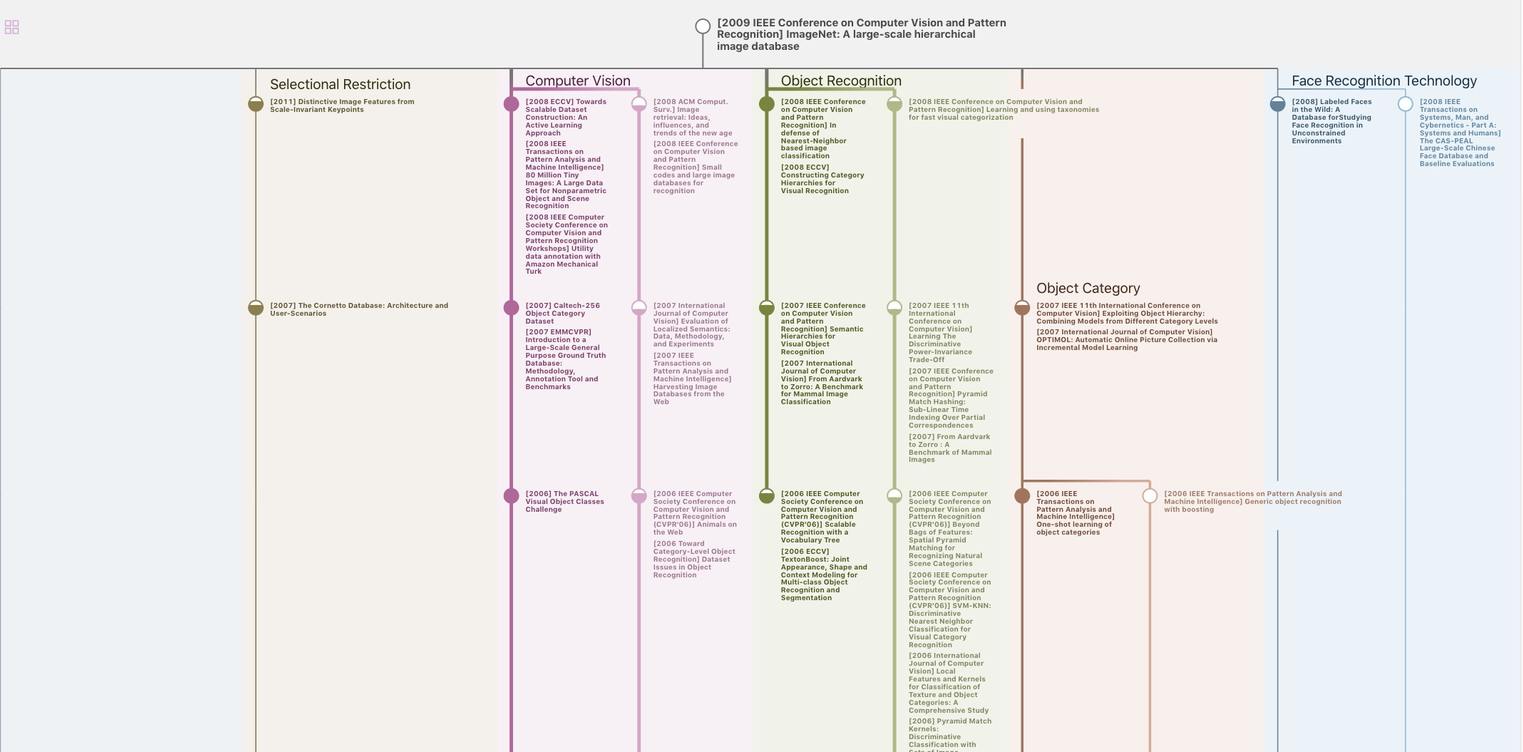
生成溯源树,研究论文发展脉络
Chat Paper
正在生成论文摘要