Causal Classification: Treatment Effect Estimation vs. Outcome Prediction
J. Mach. Learn. Res.(2022)
摘要
The goal of causal classification is to identify individuals whose outcome would be posi-tively changed by a treatment. Examples include targeting advertisements and targeting retention incentives to reduce churn. Causal classification is challenging because we ob-serve individuals under only one condition (treated or untreated), so we do not know who was influenced by the treatment, but we may estimate the potential outcomes under each condition to decide whom to treat by estimating treatment effects. Curiously, we often see practitioners using simple outcome prediction instead, for example, predicting if someone will purchase if shown the ad. Rather than disregarding this as naive behavior, we present a theoretical analysis comparing treatment effect estimation and outcome prediction when addressing causal classification. We focus on the key question: "When (if ever) is simple outcome prediction preferable to treatment effect estimation for causal classification?" The analysis reveals a causal bias-variance tradeoff. First, when the treatment effect estimation depends on two outcome predictions, larger sampling variance may lead to more errors than the (biased) outcome prediction approach. Second, a stronger signal-to-noise ratio in out-come prediction implies that the bias can help with intervention decisions when outcomes are informative of effects. The theoretical results, as well as simulations, illustrate settings where outcome prediction should actually be better, including cases where (1) the bias may be partially corrected by choosing a different threshold, (2) outcomes and treatment effects are correlated, and (3) data to estimate counterfactuals are limited. A major practical implication is that, for some applications, it might be feasible to make good intervention decisions without any data on how individuals actually behave when intervened. Finally, we show that for a real online advertising application, outcome prediction models indeed excel at causal classification.
更多查看译文
关键词
Bias-variance tradeoff, causal inference, treatment assignment
AI 理解论文
溯源树
样例
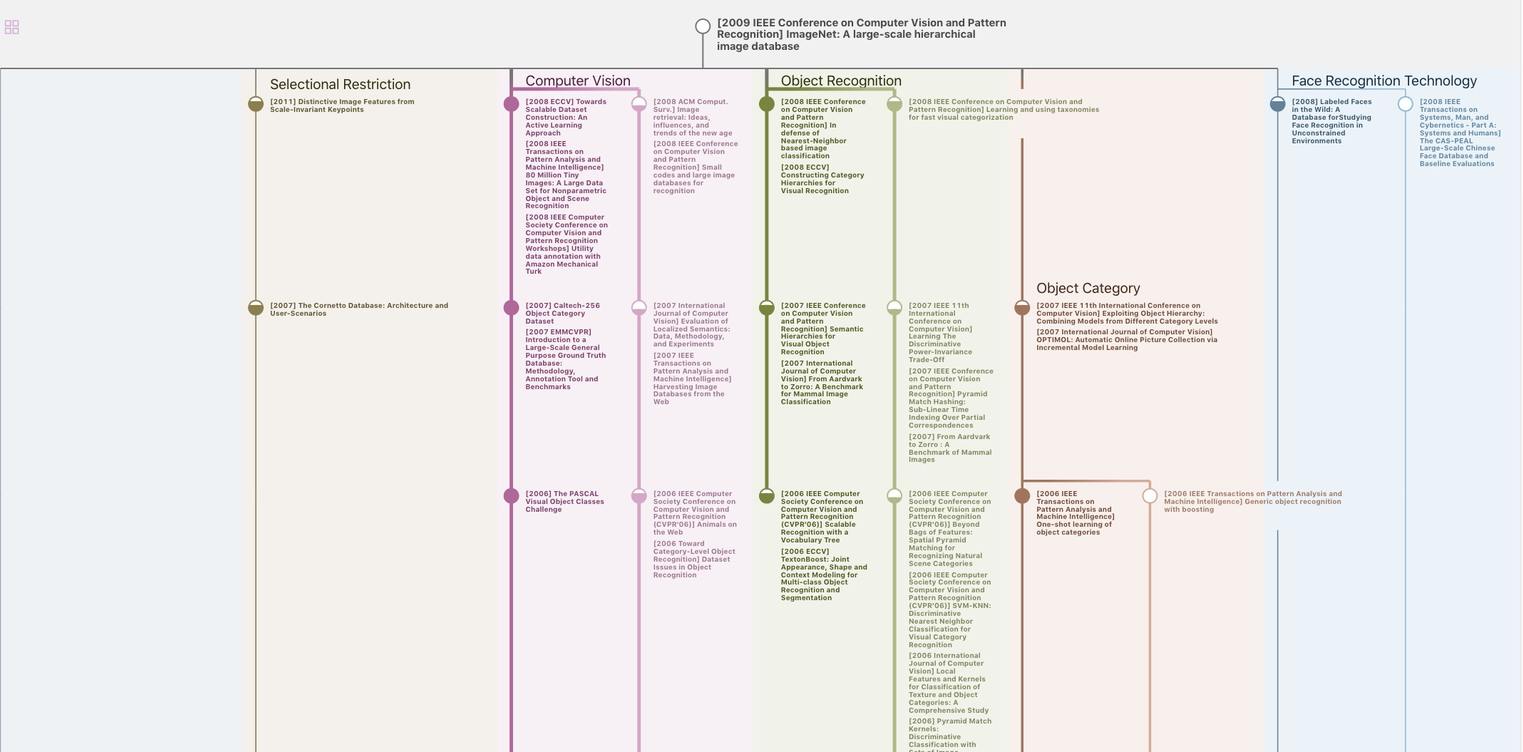
生成溯源树,研究论文发展脉络
Chat Paper
正在生成论文摘要