EMNAPE: Efficient Multi-Dimensional Neural Architecture Pruning for EdgeAI
2023 DESIGN, AUTOMATION & TEST IN EUROPE CONFERENCE & EXHIBITION, DATE(2023)
摘要
In this paper, we propose a multi-dimensional pruning framework, EMNAPE, to jointly prune the three dimensions (depth, width, and resolution) of convolutional neural networks (CNNs) for better execution efficiency on embedded hardware. In EMNAPE, we introduce a two-stage evaluation strategy to evaluate the importance of each pruning unit and identify the computational redundancy in the three dimensions. Based on the evaluation strategy, we further present a heuristic pruning algorithm to progressively prune redundant units from the three dimensions for better accuracy and efficiency. Experiments demonstrate the superiority of EMNAPE over existing methods.
更多查看译文
AI 理解论文
溯源树
样例
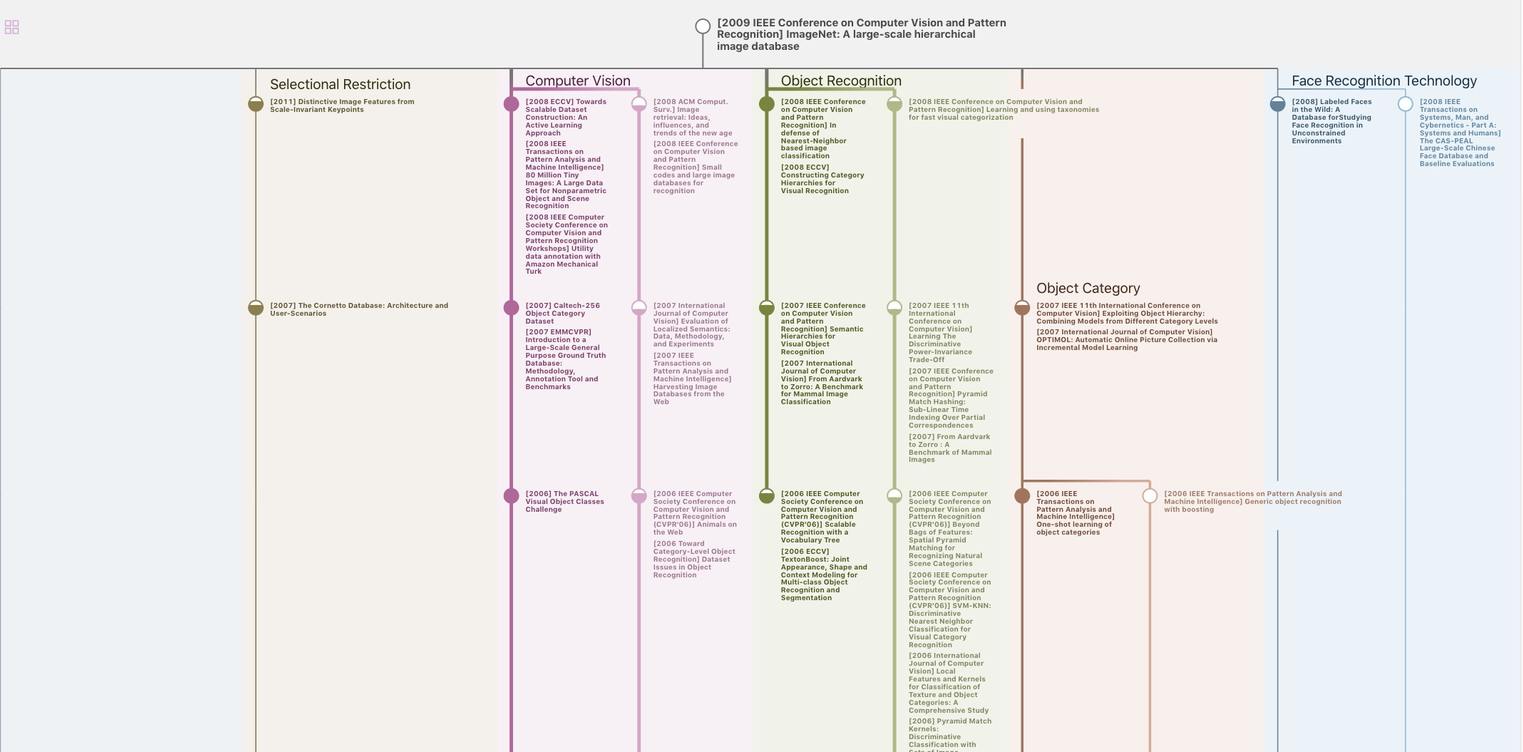
生成溯源树,研究论文发展脉络
Chat Paper
正在生成论文摘要