If only we had more data!: Sensor-Based Human Activity Recognition in Challenging Scenarios.
PerCom Workshops(2023)
摘要
With the proliferation of miniaturized movement sensing capabilities, human activity recognition using wearables and other forms of pervasive sensing (HAR) has seen an enormous boost in both research and practical deployments. Prominent application domains are health assessments, wellbeing monitoring, and novel interaction paradigms. Arguably, and especially when compared to video based activity recognition, the use of movement sensors such as inertial measurement units has many practical advantages including mobility, more direct movement capturing, and less privacy invasive sensing. However, those advantages come with a cost with regards to the design of machine learning based pipelines for the analysis of the sensor data streams, i.e., multi-variate time series data. Probably the biggest challenge lies in the absence of (very) large, labeled datasets that can readily be used for developing and training complex analysis models. In this paper I explore the challenges for deriving machine learning based systems for sensor-based human activity recognition. My focus is on: i) the role of representation learning in modern HAR systems that overcome the need for explicit feature engineering; ii) self-supervised methods for most effective use of small, labeled datasets; and iii) cross-modality transfer. I conclude with an outlook of next steps in the field that promise to lead to increased robustness and more flexible applicability of contemporary, machine-learnlng based HAR systems.
更多查看译文
关键词
human activity recognition,wearables,pervasive and ubiquitous computing,machine learning
AI 理解论文
溯源树
样例
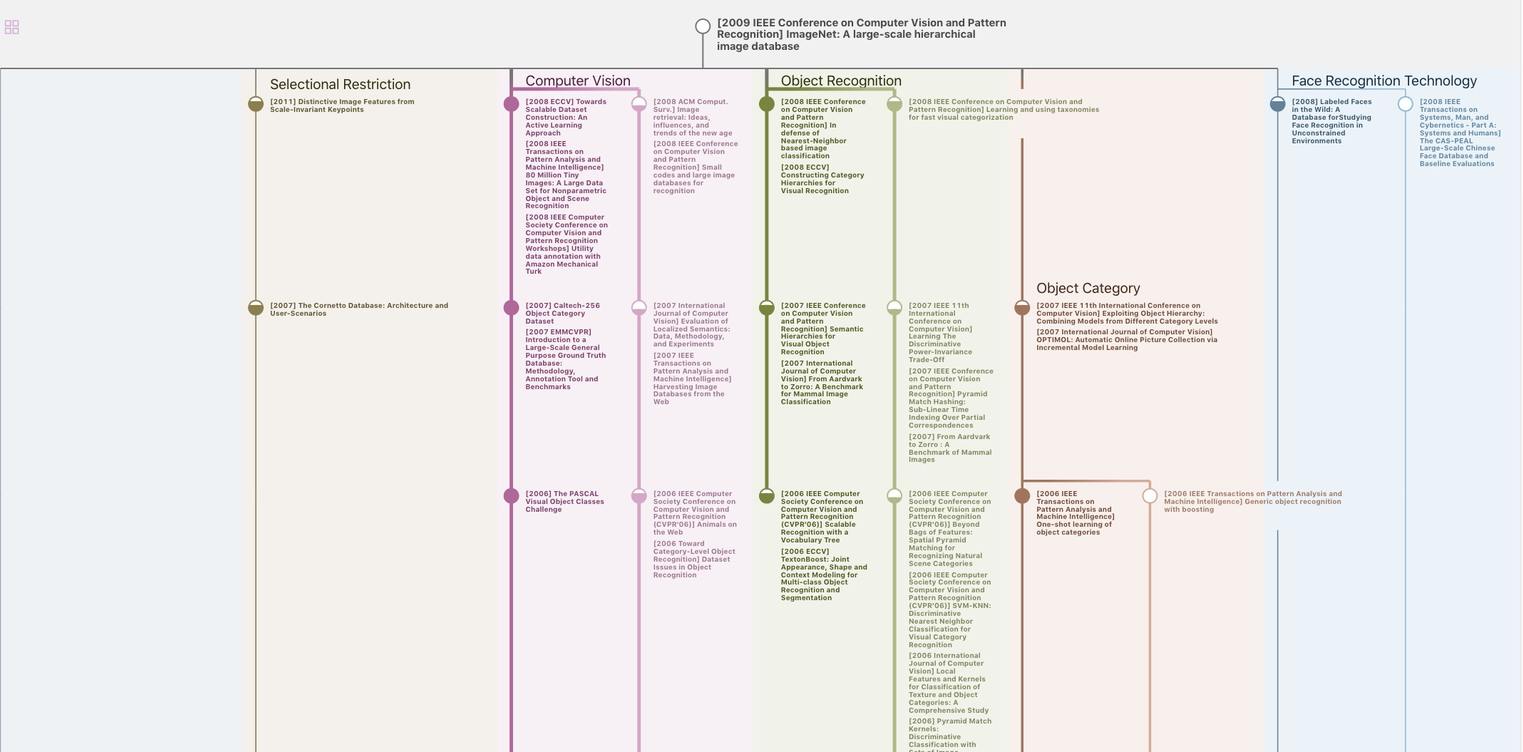
生成溯源树,研究论文发展脉络
Chat Paper
正在生成论文摘要