Learning Symbolic Timed Models from Concrete Timed Data.
NFM(2023)
摘要
We present a technique for learning explainable timed automata from passive observations of a black-box function, such as an artificial intelligence system. Our method accepts a single, long, timed word with mixed input and output actions and learns a Mealy machine with one timer. The primary advantage of our approach is that it constructs a symbolic observation tree from a concrete timed word. This symbolic tree is then transformed into a human comprehensible automaton. We provide a prototype implementation and evaluate it by learning the controllers of two systems: a brick-sorter conveyor belt trained with reinforcement learning and a real-world derived smart traffic light controller. We compare different model generators using our symbolic observation tree as their input and achieve the best results using k -tails. In our experiments, we learn smaller and simpler automata than existing passive timed learners while maintaining accuracy.
更多查看译文
关键词
symbolic timed models,concrete timed data,learning
AI 理解论文
溯源树
样例
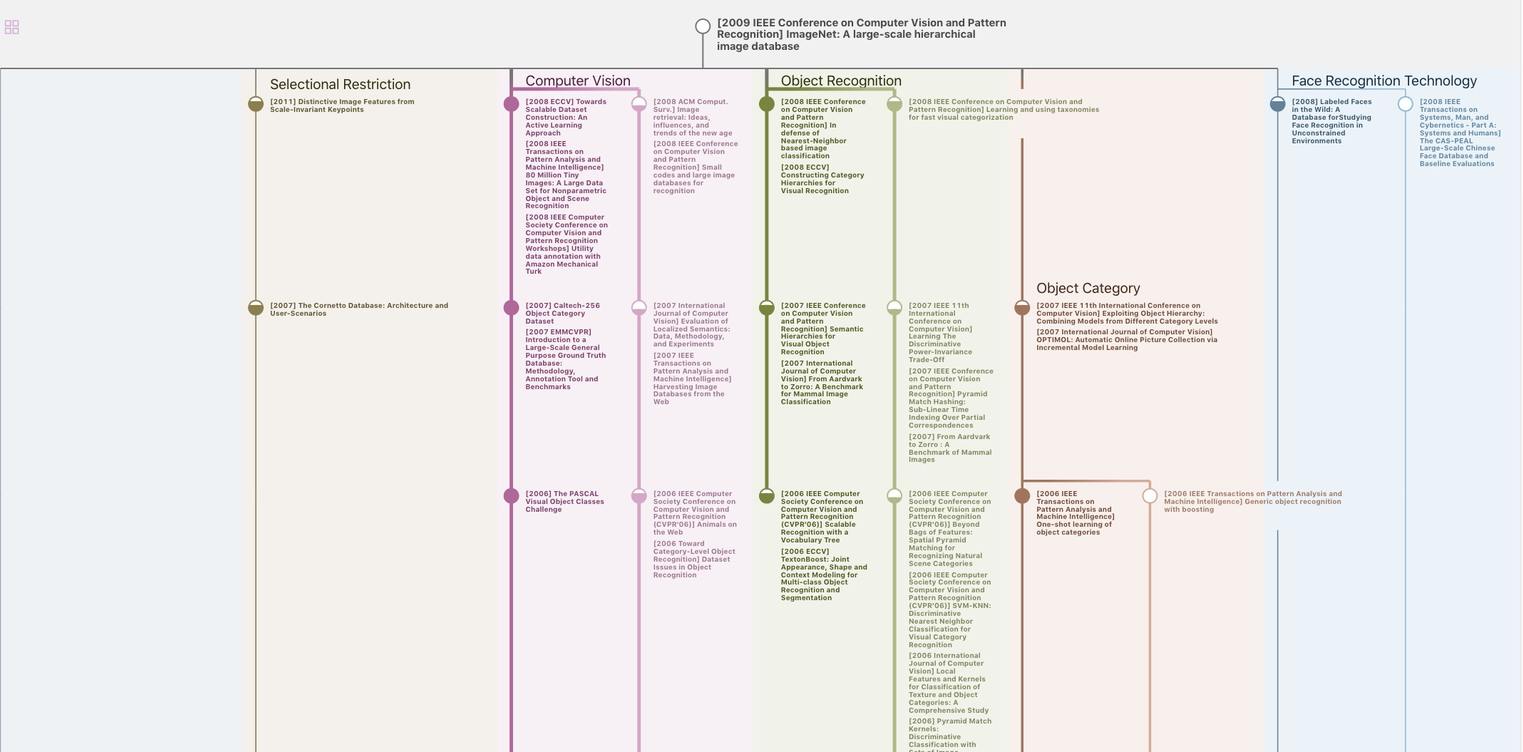
生成溯源树,研究论文发展脉络
Chat Paper
正在生成论文摘要