Uniform Convergence with Square-Root Lipschitz Loss
NeurIPS(2023)
摘要
We establish generic uniform convergence guarantees for Gaussian data in terms of the Rademacher complexity of the hypothesis class and the Lipschitz constant of the square root of the scalar loss function. We show how these guarantees substantially generalize previous results based on smoothness (Lipschitz constant of the derivative), and allow us to handle the broader class of square-root-Lipschitz losses, which includes also non-smooth loss functions appropriate for studying phase retrieval and ReLU regression, as well as rederive and better understand "optimistic rate" and interpolation learning guarantees.
更多查看译文
关键词
convergence,loss,square-root
AI 理解论文
溯源树
样例
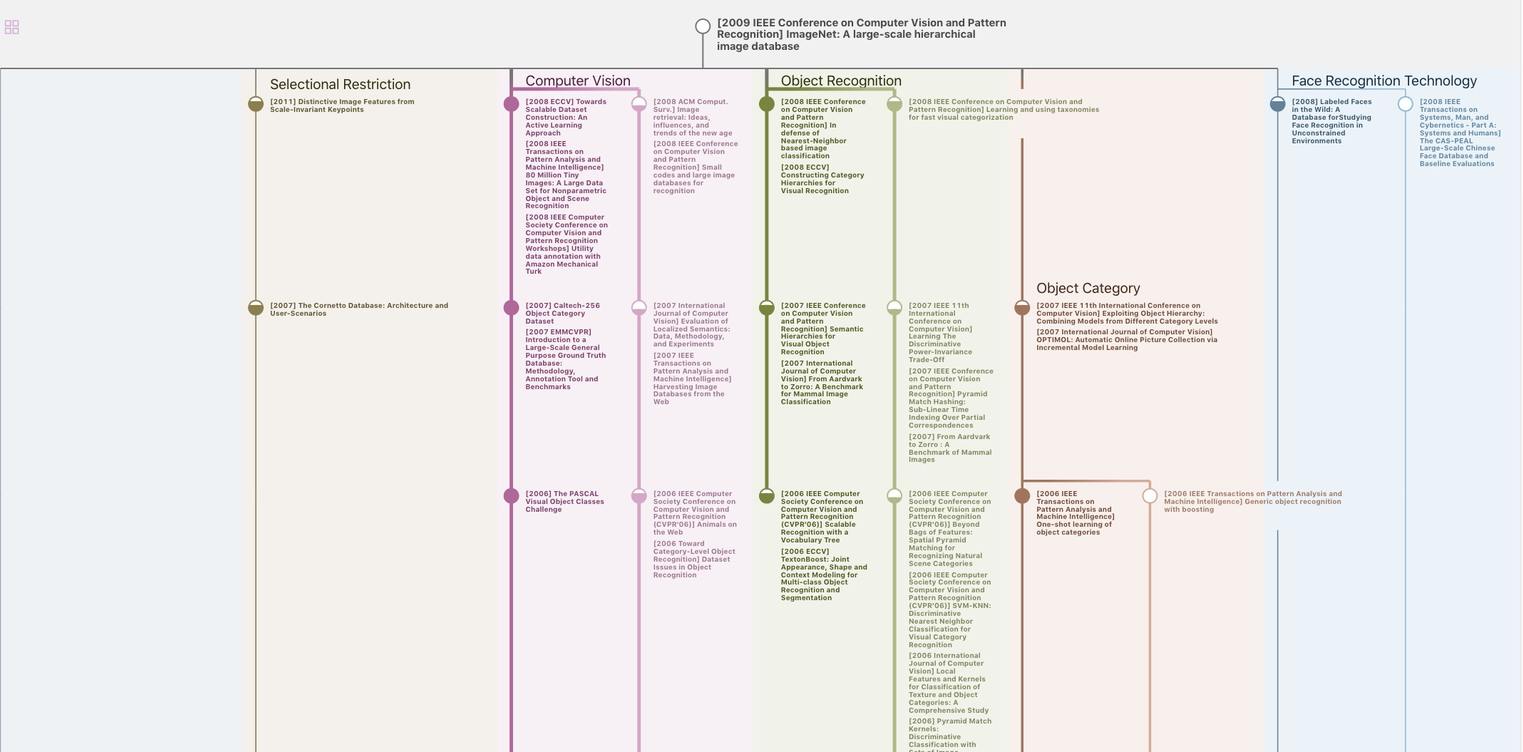
生成溯源树,研究论文发展脉络
Chat Paper
正在生成论文摘要