Black-Box Acceleration of Monotone Convex Program Solvers
OPERATIONS RESEARCH(2024)
摘要
This paper presents a black-box framework for accelerating packing optimization solvers. Our method applies to packing linear programming problems and a family of convex programming problems with linear constraints. The framework is designed for high-dimensional problems, for which the number of variables n is much larger than the number of measurements m. Given an (m x n) problem, we construct a smaller (m x epsilon n) problem, whose solution we use to find an approximation to the optimal solution. Our framework can accelerate both exact and approximate solvers. If the solver being accelerated produces an a-approximation, then we produce a (1 - epsilon)/alpha(2)-approximation of the optimal solution to the original problem. We present worst-case guarantees on run time and empirically demonstrate speedups of two orders of magnitude.
更多查看译文
关键词
linear programming,convex optimization,dimension reduction
AI 理解论文
溯源树
样例
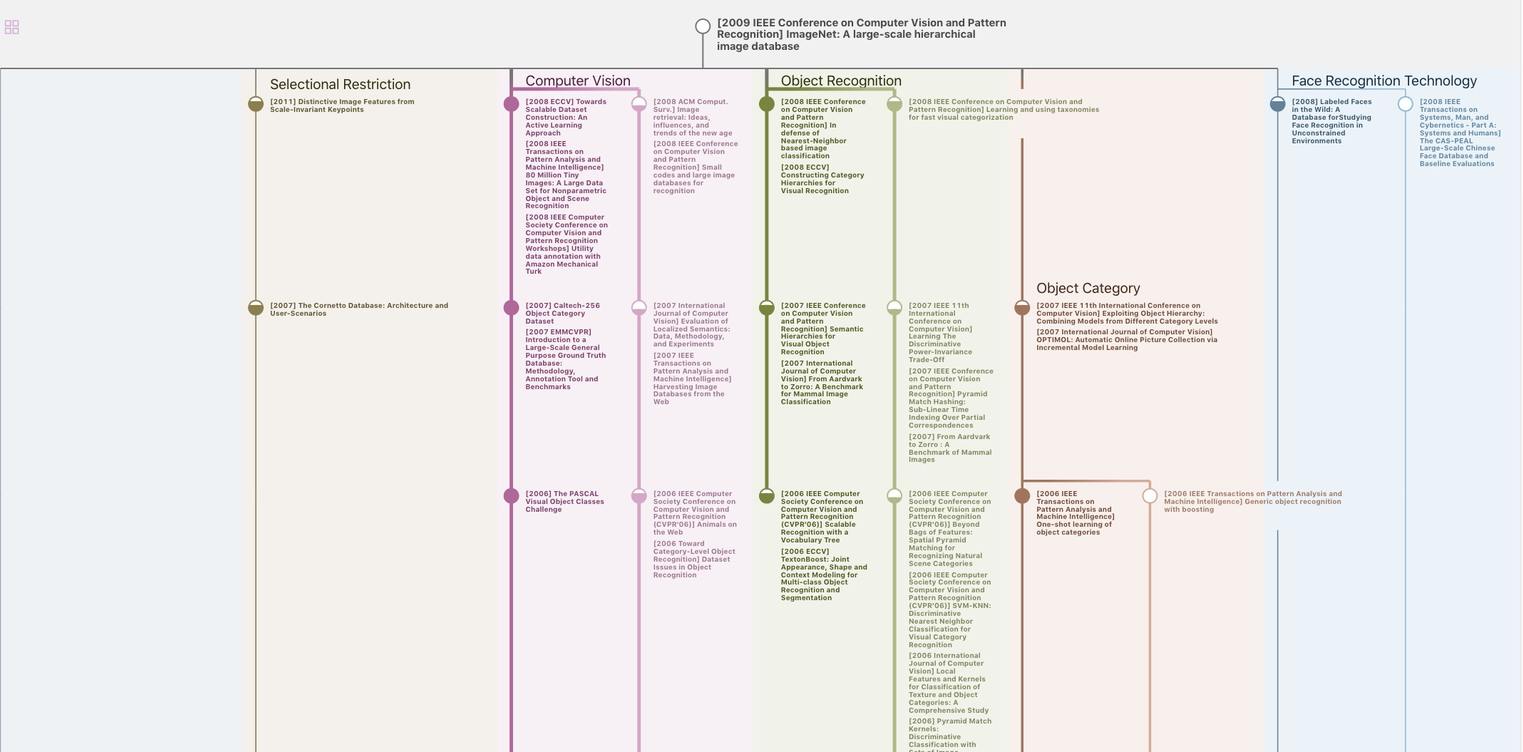
生成溯源树,研究论文发展脉络
Chat Paper
正在生成论文摘要