Fusion learning of preference and bias from ratings and reviews for item recommendation
DATA & KNOWLEDGE ENGINEERING(2024)
摘要
Recommendation methods improve rating prediction performance by learning selection bias phenomenon -users tend to rate items they like. These methods model selection bias by calculating the propensities of ratings, but inaccurate propensity could introduce more noise, fail to model selection bias, and reduce prediction performance. We argue that learning interaction features can effectively model selection bias and improve model performance, as interaction features explain the reason of the trend. Reviews can be used to model interaction features because they have a strong intrinsic correlation with user interests and item interactions. In this study, we propose a preference- and bias -oriented fusion learning model (PBFL) that models the interaction features based on reviews and user preferences to make rating predictions. Our proposal both embeds traditional user preferences in reviews, interactions, and ratings and considers word distribution bias and review quoting to model interaction features. Six realworld datasets are used to demonstrate effectiveness and performance. PBFL achieves an average improvement of 4.46% in root -mean -square error (RMSE) and 3.86% in mean absolute error (MAE) over the best baseline.
更多查看译文
关键词
Data mining,Text mining,Recommender systems,Selection bias,Interaction feature
AI 理解论文
溯源树
样例
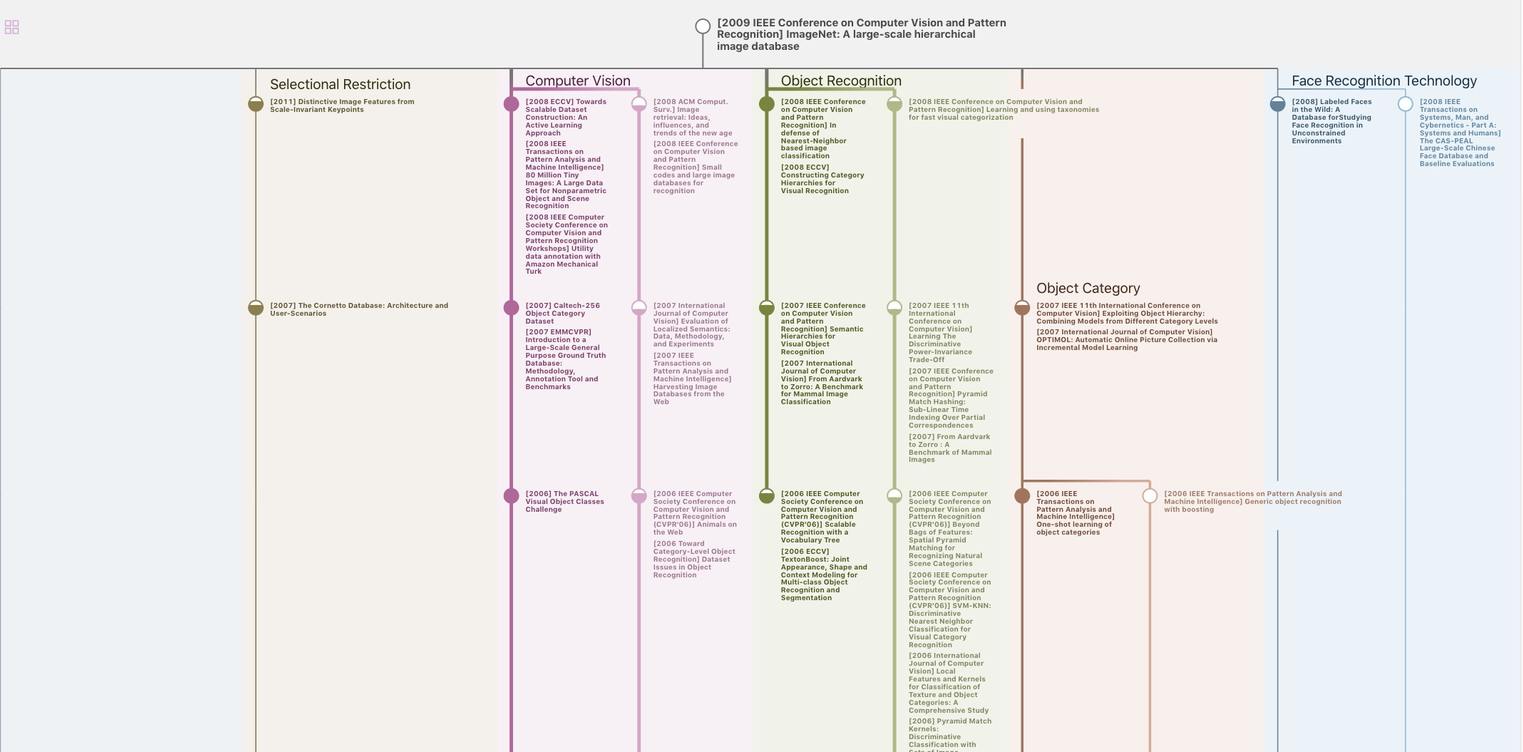
生成溯源树,研究论文发展脉络
Chat Paper
正在生成论文摘要