AutoFL: A Bayesian Game Approach for Autonomous Client Participation in Federated Edge Learning
IEEE TRANSACTIONS ON MOBILE COMPUTING(2024)
摘要
Given that devices (i.e., clients) participating in federated edge learning (FEL) are autonomous and resource-constrained in nature, it is critical to design effective incentive mechanisms to encourage client participation so as to improve the performance of FEL. In this article, we aim to boost the FEL training efficiency by answering how much compute resource should clients autonomously contribute to maximize their utilities. To this end, we develop AutoFL, an autonomous client participation decision framework for federated learning at the network edge without assuming that each client possesses complete information. We first model the problem of autonomous client participation as a Bayesian game with incomplete information, where each player in the game is associated with a set of types according to network conditions. We optimize an individual client's decision based on the dynamics of the population estimated following the Bayes rule. We prove that AutoFL can converge to a unique Bayesian Nash equilibrium point. Empirical results on three real datasets show that AutoFL achieves a higher model accuracy with only 15.5-24.5% model aggregation time per global training round, and its energy cost saving on mobile devices is 82.2-86.8% compared to the state-of-the-art algorithms. Moreover, we can achieve a 2.75-3.2x long-term fairness compared to classical solutions.
更多查看译文
关键词
Training,Games,Costs,Computational modeling,Servers,Bayes methods,Task analysis,Bayesian game,client participation,federated edge learning,incomplete information
AI 理解论文
溯源树
样例
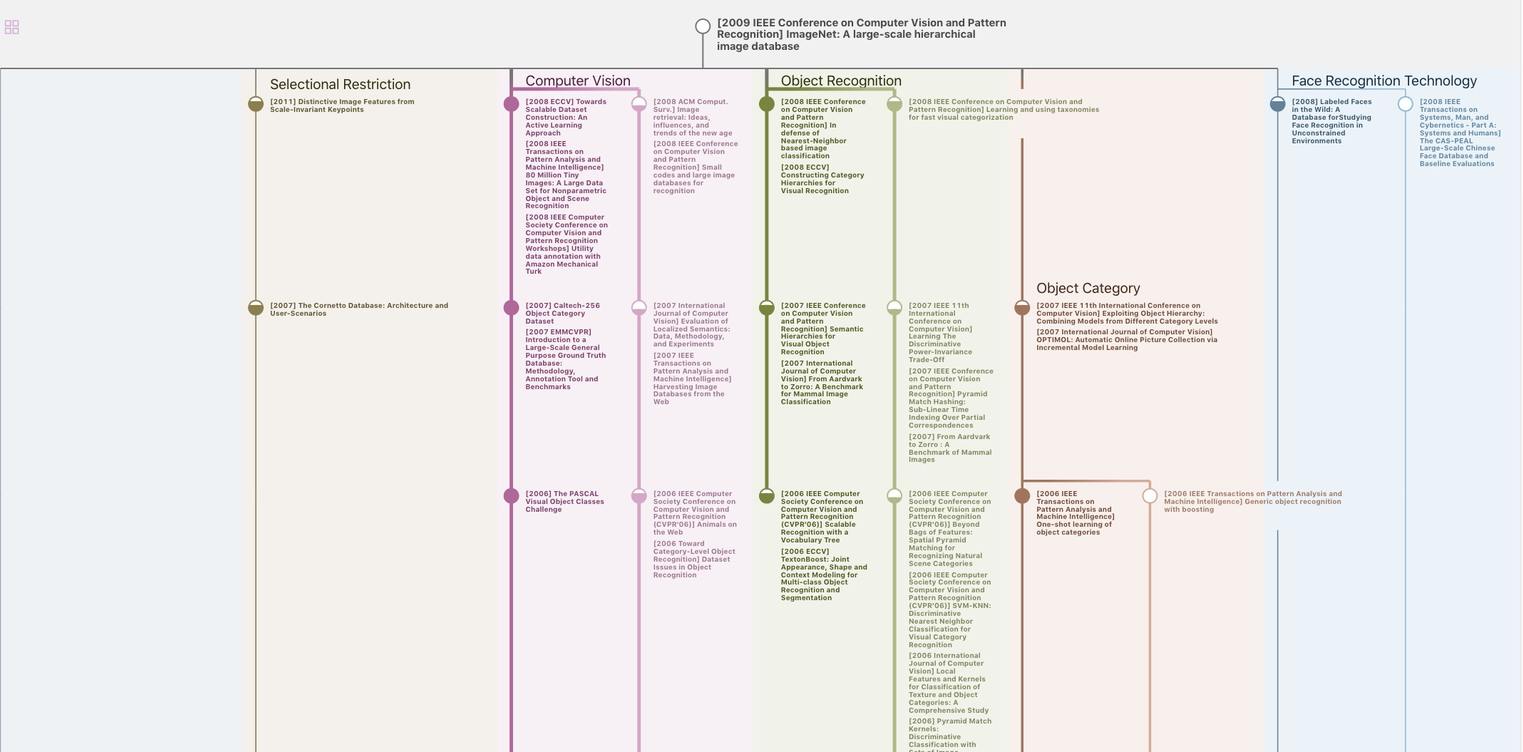
生成溯源树,研究论文发展脉络
Chat Paper
正在生成论文摘要