SketchNE: Embedding Billion-Scale Networks Accurately in One Hour
IEEE Transactions on Knowledge and Data Engineering(2023)
摘要
We study large-scale network embedding with the goal of generating high-quality embeddings for networks with more than 1 billion vertices and 100 billion edges. Recent attempts LightNE and NetSMF propose to sparsify and factorize the (dense) NetMF matrix for embedding large networks, where NetMF is a theoretically-grounded network embedding method. However, there is a trade-off between their embeddings’ quality and scalability due to their expensive memory requirements, making embeddings less effective under real-world memory constraints. Therefore, we present the SketchNE model, a scalable, effective, and memory-efficient network embedding solution developed for a single machine with CPU only. The main idea of SketchNE is to avoid the explicit construction and factorization of the NetMF matrix either sparsely or densely when producing the embeddings through the proposed sparse-sign randomized single-pass SVD algorithm. We conduct extensive experiments on nine datasets of various sizes for vertex classification and link prediction, demonstrating the consistent outperformance of SketchNE over state-of-the-art baselines in terms of both effectiveness and efficiency. SketchNE costs only
1.0 hours
to embed the Hyperlink2012 network with
3.5 billion
vertices and
225 billion
edges on a CPU-only single machine with embedding superiority (e.g., a
282%
relative HITS@10 gain over LightNE).
更多查看译文
关键词
networks,billion-scale
AI 理解论文
溯源树
样例
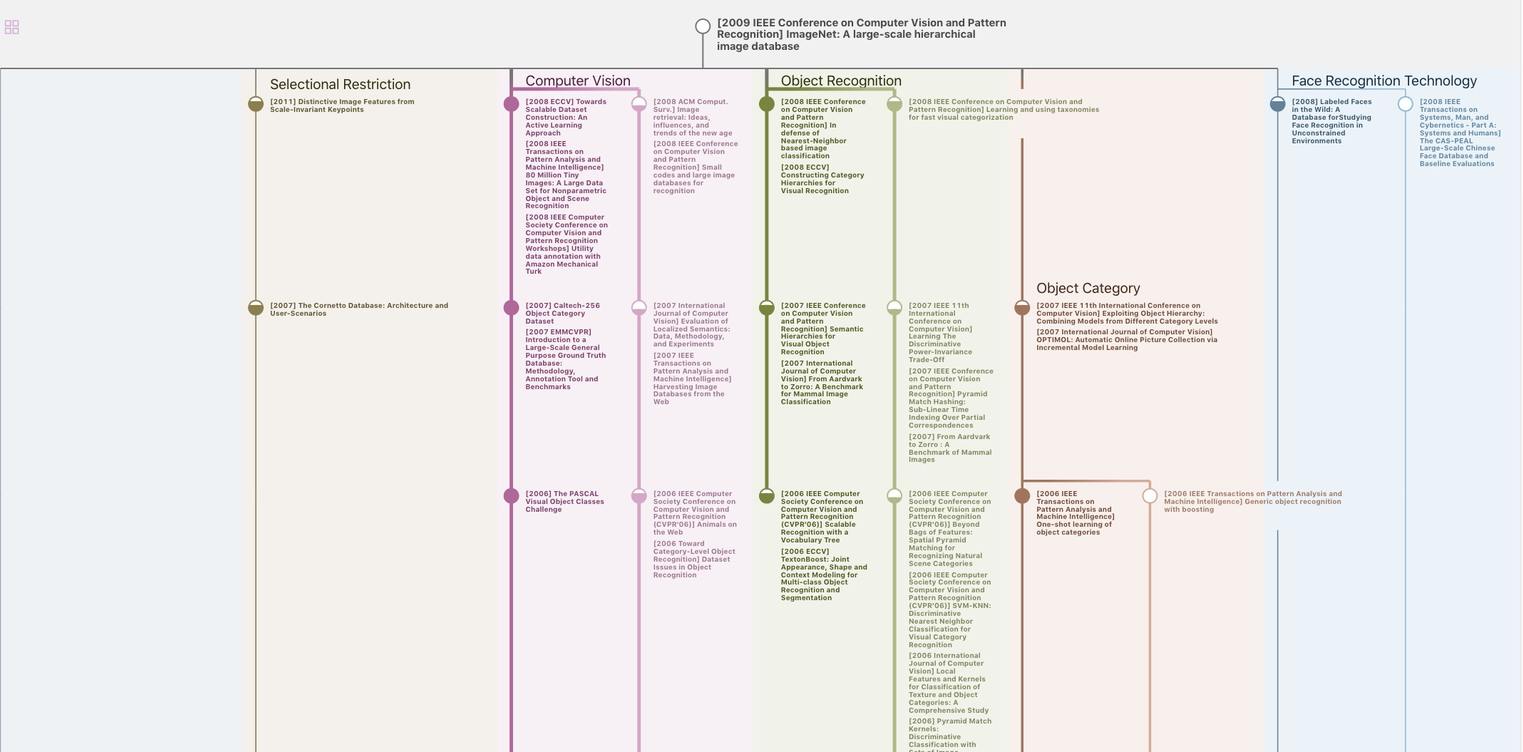
生成溯源树,研究论文发展脉络
Chat Paper
正在生成论文摘要