Full Waveform Inversion-Based Ultrasound Computed Tomography Acceleration Using Two-Dimensional Convolutional Neural Networks
Journal of Nondestructive Evaluation, Diagnostics and Prognostics of Engineering Systems(2023)
摘要
Ultrasound computed tomography (USCT) shows great promise in nondestructive evaluation and medical imaging due to its ability to quickly scan and collect data from a region of interest. However, existing approaches are a tradeoff between the accuracy of the prediction and the speed at which the data can be analyzed, and processing the collected data into a meaningful image requires both time and computational resources. We propose to develop convolutional neural networks (CNNs) to accelerate and enhance the inversion results to reveal underlying structures or abnormalities that may be located within the region of interest. For training, the ultrasonic signals were first processed using the full waveform inversion (FWI) technique for only a single iteration; the resulting image and the corresponding true model were used as the input and output, respectively. The proposed machine learning approach is based on implementing two-dimensional CNNs to find an approximate solution to the inverse problem of a partial differential equation-based model reconstruction. To alleviate the time-consuming and computationally intensive data generation process, a high-performance computing-based framework has been developed to generate the training data in parallel. At the inference stage, the acquired signals will be first processed by FWI for a single iteration; then the resulting image will be processed by a pre-trained CNN to instantaneously generate the final output image. The results showed that once trained, the CNNs can quickly generate the predicted wave speed distributions with significantly enhanced speed and accuracy.
更多查看译文
关键词
ultrasonic imaging,full waveform inversion,convolutional neural networks,ultrasound computed tomography,deep learning
AI 理解论文
溯源树
样例
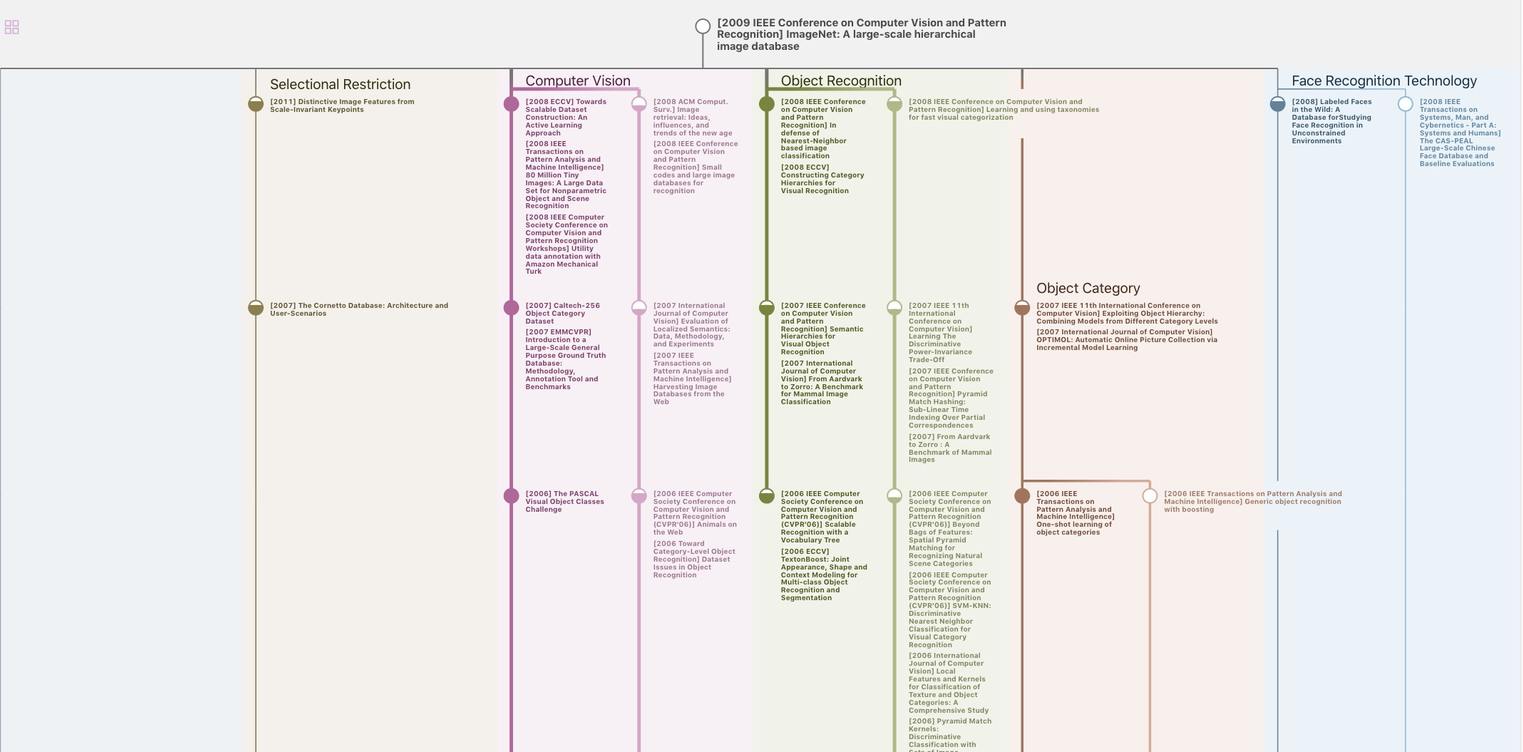
生成溯源树,研究论文发展脉络
Chat Paper
正在生成论文摘要