A Unified Model and Dimension for Interactive Estimation
arXiv (Cornell University)(2023)
摘要
We study an abstract framework for interactive learning called interactive estimation in which the goal is to estimate a target from its "similarity'' to points queried by the learner. We introduce a combinatorial measure called dissimilarity dimension which largely captures learnability in our model. We present a simple, general, and broadly-applicable algorithm, for which we obtain both regret and PAC generalization bounds that are polynomial in the new dimension. We show that our framework subsumes and thereby unifies two classic learning models: statistical-query learning and structured bandits. We also delineate how the dissimilarity dimension is related to well-known parameters for both frameworks, in some cases yielding significantly improved analyses.
更多查看译文
关键词
estimation,unified model,dimension
AI 理解论文
溯源树
样例
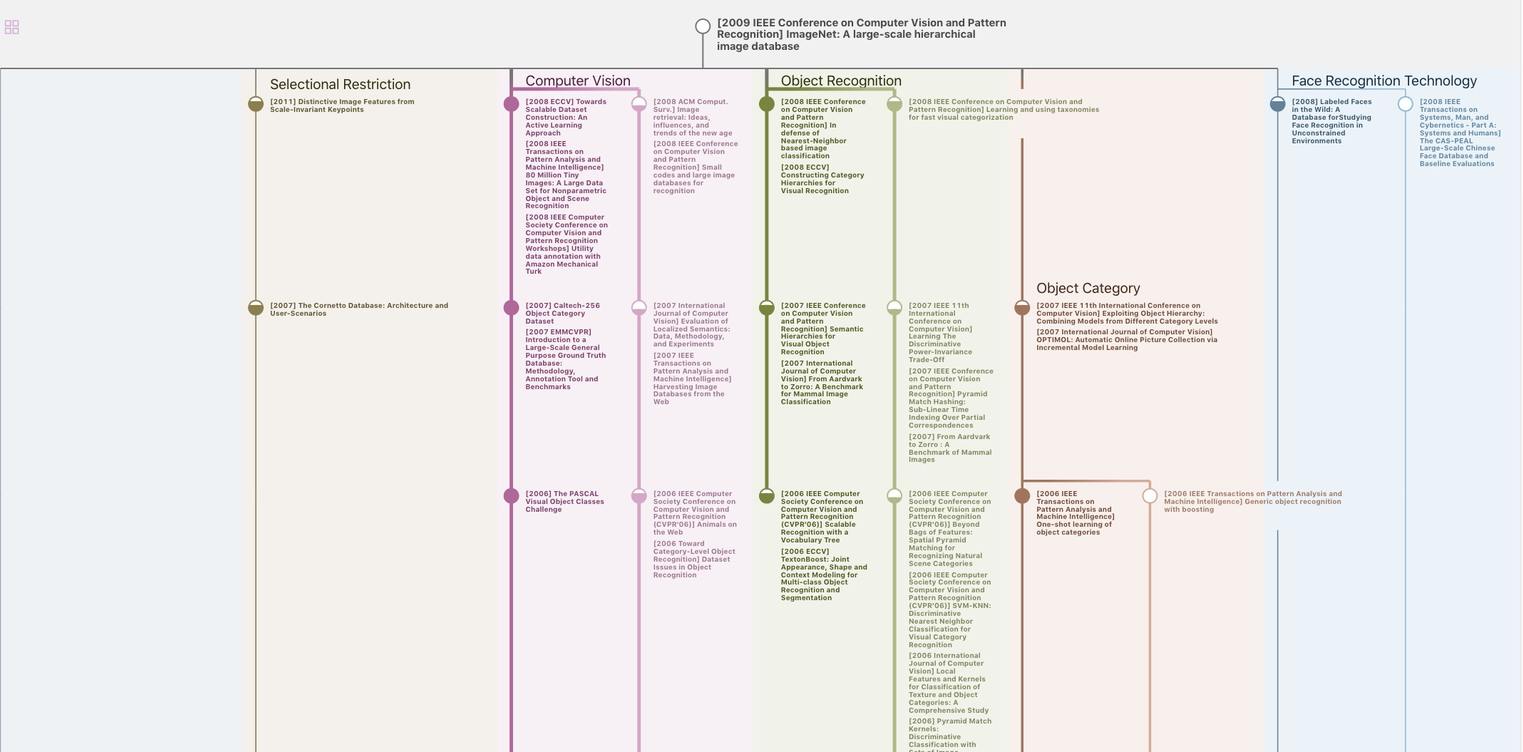
生成溯源树,研究论文发展脉络
Chat Paper
正在生成论文摘要