Octree-based hierarchical sampling optimization for the volumetric super-resolution of scientific data
JOURNAL OF COMPUTATIONAL PHYSICS(2024)
摘要
When introducing physics -constrained deep learning solutions to the volumetric super -resolution of scientific data, the training is challenging to converge and always time-consuming. We propose a new hierarchical sampling method based on octree to solve these difficulties. In our approach, scientific data is preprocessed before training, and a hierarchical octree-based data structure is built to guide sampling on the latent context grid. Each leaf node in the octree corresponds to an indivisible subblock of the volumetric data. The dimensions of the subblocks are different, making the number of sample points in each randomly cropped training data block to be adaptive. We reconstruct the octree at intervals according to loss distribution to perform the multi -stage training. With the Rayleigh-Benard convection problem, we deploy our method to state-of-theart models. We constructed adequate experiments to evaluate the training performance and model accuracy of our method. Experiments indicate that our sampling optimization improves the convergence performance of physics -constrained deep learning super -resolution solutions. Furthermore, the sample points and training time are significantly reduced with no drop in model accuracy. We also test our method in training tasks of other deep neural networks, and the results show our sampling optimization has extensive effectiveness and applicability. The code is publicly available at https://github .com /xinjiewang /octree -based _sampling.
更多查看译文
关键词
Scientific data,Volumetric super-resolution,Octree,Importance sampling,Physics-constrained deep learning
AI 理解论文
溯源树
样例
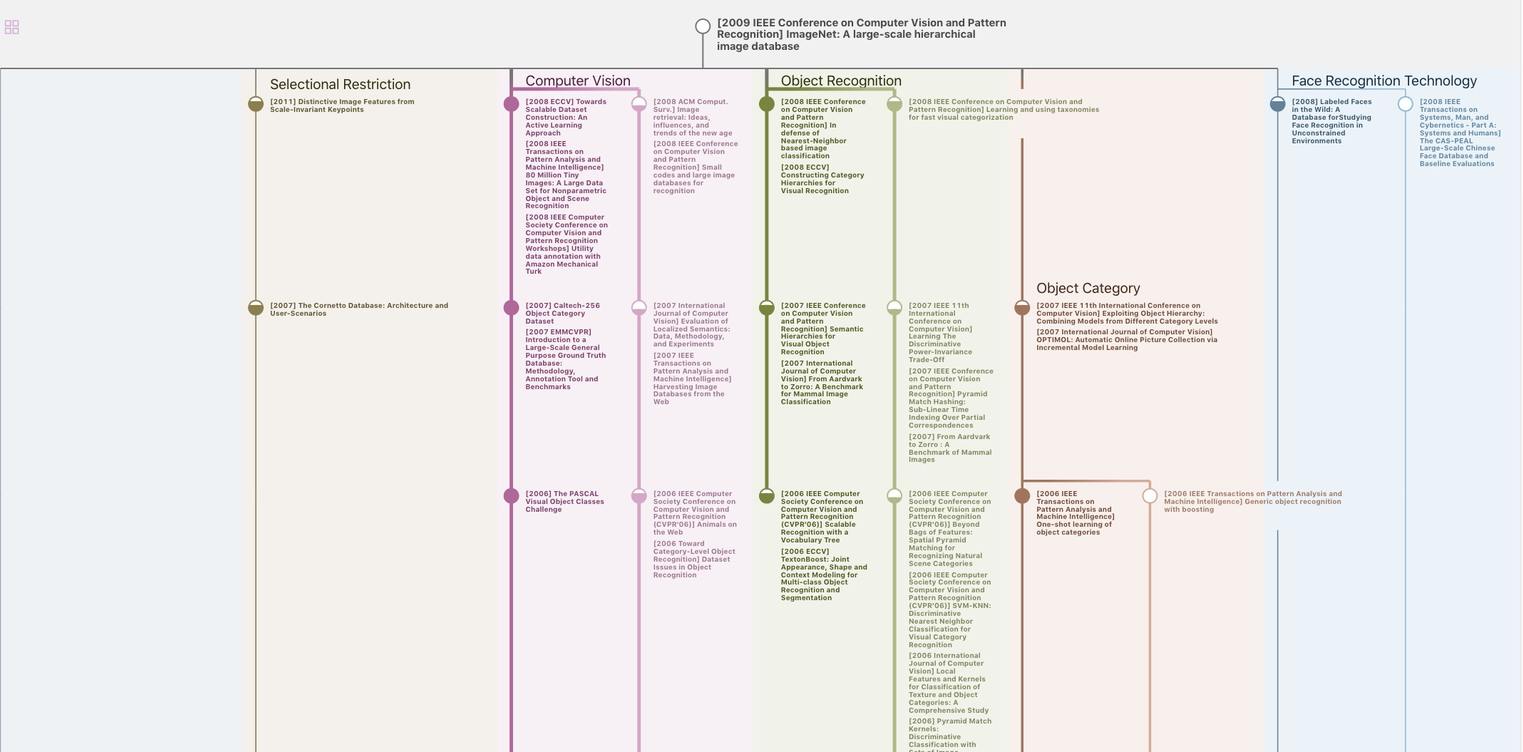
生成溯源树,研究论文发展脉络
Chat Paper
正在生成论文摘要