Self-Supervised Spontaneous Latent-Based Facial Expression Sequence Generation
IEEE Open Journal of Signal Processing(2023)
摘要
In this article, we investigate the spontaneity issue in facial expression sequence generation. Current leading methods in the field are commonly reliant on manually adjusted conditional variables to direct the model to generate a specific class of expression. We propose a neural network-based method which uses Gaussian noise to model spontaneity in the generation process, removing the need for manual control of conditional generation variables. Our model takes two sequential images as input, with additive noise, and produces the next image in the sequence. We trained two types of models: single-expression, and mixed-expression. With single-expression, unique facial movements of certain emotion class can be generated; with mixed expressions, fully spontaneous expression sequence generation can be achieved. We compared our method to current leading generation methods on a variety of publicly available datasets. Initial qualitative results show our method produces visually more realistic expressions and facial action unit (AU) trajectories; initial quantitative results using image quality metrics (SSIM and NIQE) show the quality of our generated images is higher. Our approach and results are novel in the field of facial expression generation, with potential wider applications to other sequence generation tasks.
更多查看译文
关键词
Faces,Mathematical models,Markov processes,Gold,Training,Task analysis,Image sequences,Affective computing,artificial neural networks,self-supervised learning
AI 理解论文
溯源树
样例
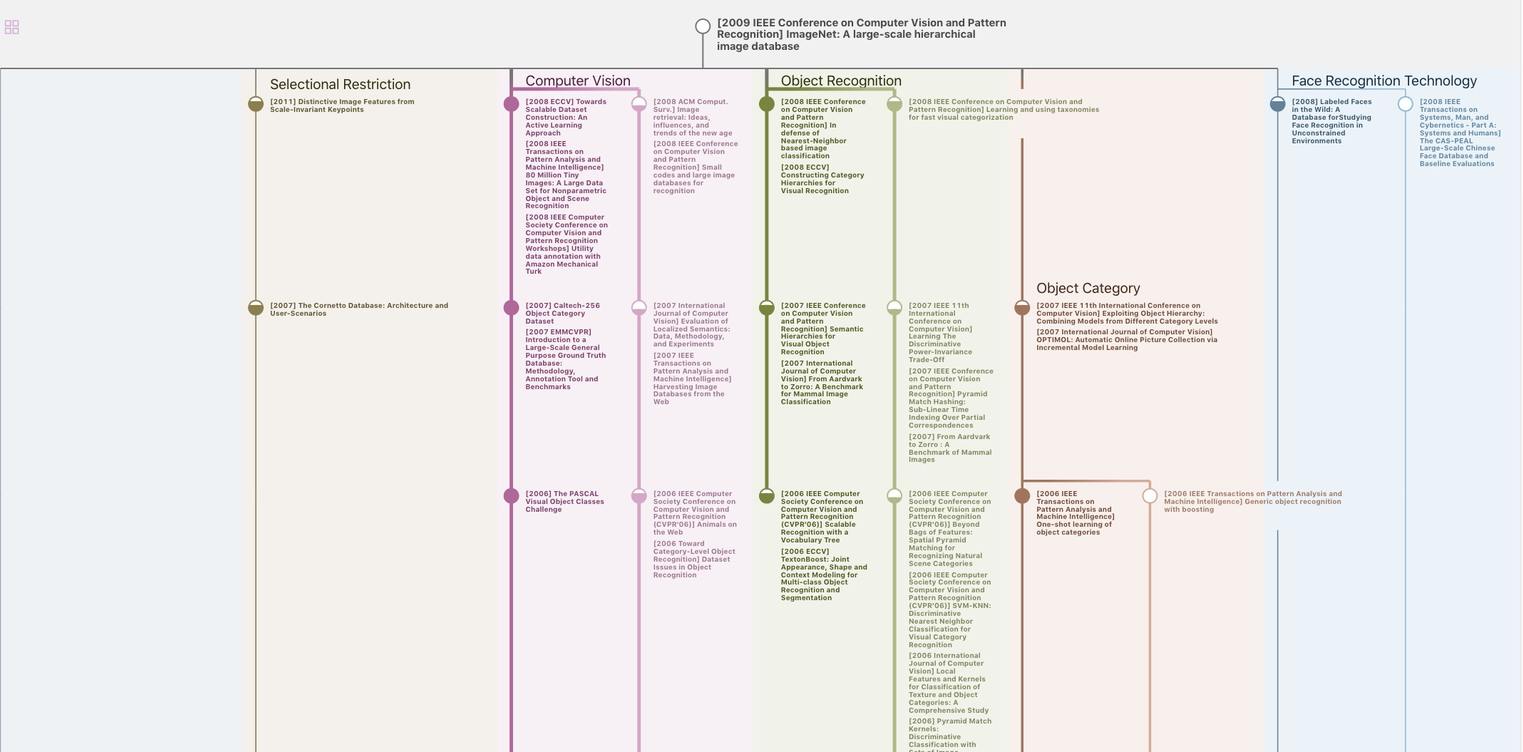
生成溯源树,研究论文发展脉络
Chat Paper
正在生成论文摘要