Combining Digital Covariates and Machine Learning Models to Predict the Spatial Variation of Soil Cation Exchange Capacity
LAND(2023)
摘要
Cation exchange capacity (CEC) is a soil property that significantly determines nutrient availability and effectiveness of fertilizer applied in lands under different managements. CEC's accurate and high-resolution spatial information is needed for the sustainability of agricultural management on farms in the Nagaland state (northeast India) which are fragmented and intertwined with the forest ecosystem. The current study applied the digital soil mapping (DSM) methodology, based on the CEC values determined in soil samples obtained from 305 points in the region, which is mountainous and difficult to access. Firstly, digital auxiliary data were obtained from three open-access sources, including indices generated from the time series Landsat 8 OLI satellite, topographic variables derived from a digital elevation model (DEM), and the WorldClim dataset. Furthermore, the CEC values and the auxiliary were used data to model Lasso regression (LR), stochastic gradient boosting (GBM), support vector regression (SVR), random forest (RF), and K-nearest neighbors (KNN) machine learning (ML) algorithms were systematically compared in the R-Core Environment Program. Model performance were evaluated with the square root mean error (RMSE), determination coefficient (R-2), and mean absolute error (MAE) of 10-fold cross-validation (CV). The lowest RMSE was obtained by the RF algorithm with 4.12 cmol(c) kg(-1), while the others were in the following order: SVR (4.27 cmol(c) kg(-1)) 更多
查看译文
关键词
soil,digital covariates,machine learning models,spatial variation,machine learning
AI 理解论文
溯源树
样例
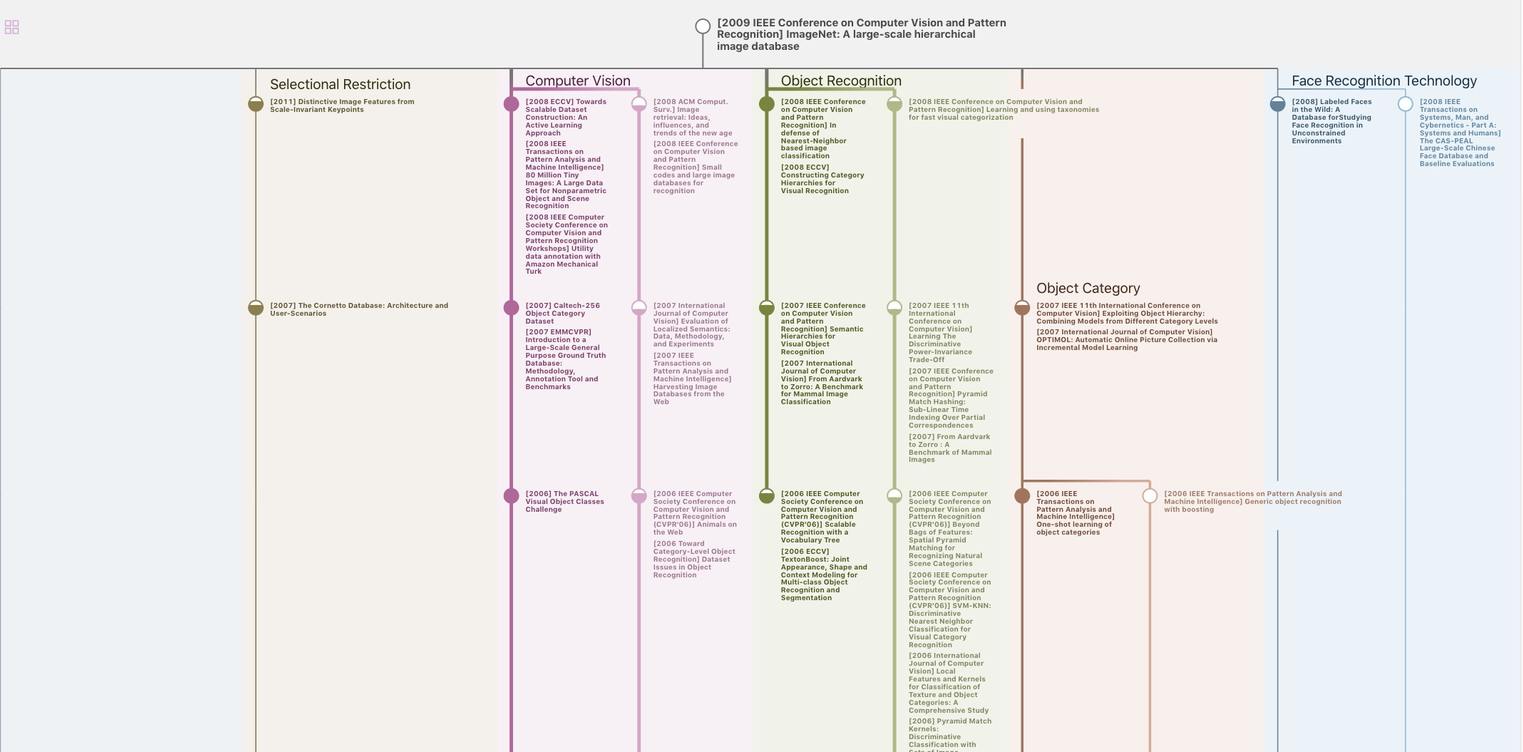
生成溯源树,研究论文发展脉络
Chat Paper
正在生成论文摘要