Large Language Model Augmented Narrative Driven Recommendations
PROCEEDINGS OF THE 17TH ACM CONFERENCE ON RECOMMENDER SYSTEMS, RECSYS 2023(2023)
摘要
Narrative-driven recommendation (NDR) presents an information access problem where users solicit recommendations with verbose descriptions of their preferences and context, for example, travelers soliciting recommendations for points of interest while describing their likes/dislikes and travel circumstances. These requests are increasingly important with the rise of natural language-based conversational interfaces for search and recommendation systems. However, NDR lacks abundant training data for models, and current platforms commonly do not support these requests. Fortunately, classical user-item interaction datasets contain rich textual data, e.g., reviews, which often describe user preferences and context - this may be used to bootstrap training for NDR models. In this work, we explore using large language models (LLMs) for data augmentation to train NDR models. We use LLMs for authoring synthetic narrative queries from user-item interactions with fewshot prompting and train retrieval models for NDR on synthetic queries and user-item interaction data. Our experiments demonstrate that this is an effective strategy for training small-parameter retrieval models that outperform other retrieval and LLM baselines for narrative-driven recommendation.
更多查看译文
关键词
recommendations,language
AI 理解论文
溯源树
样例
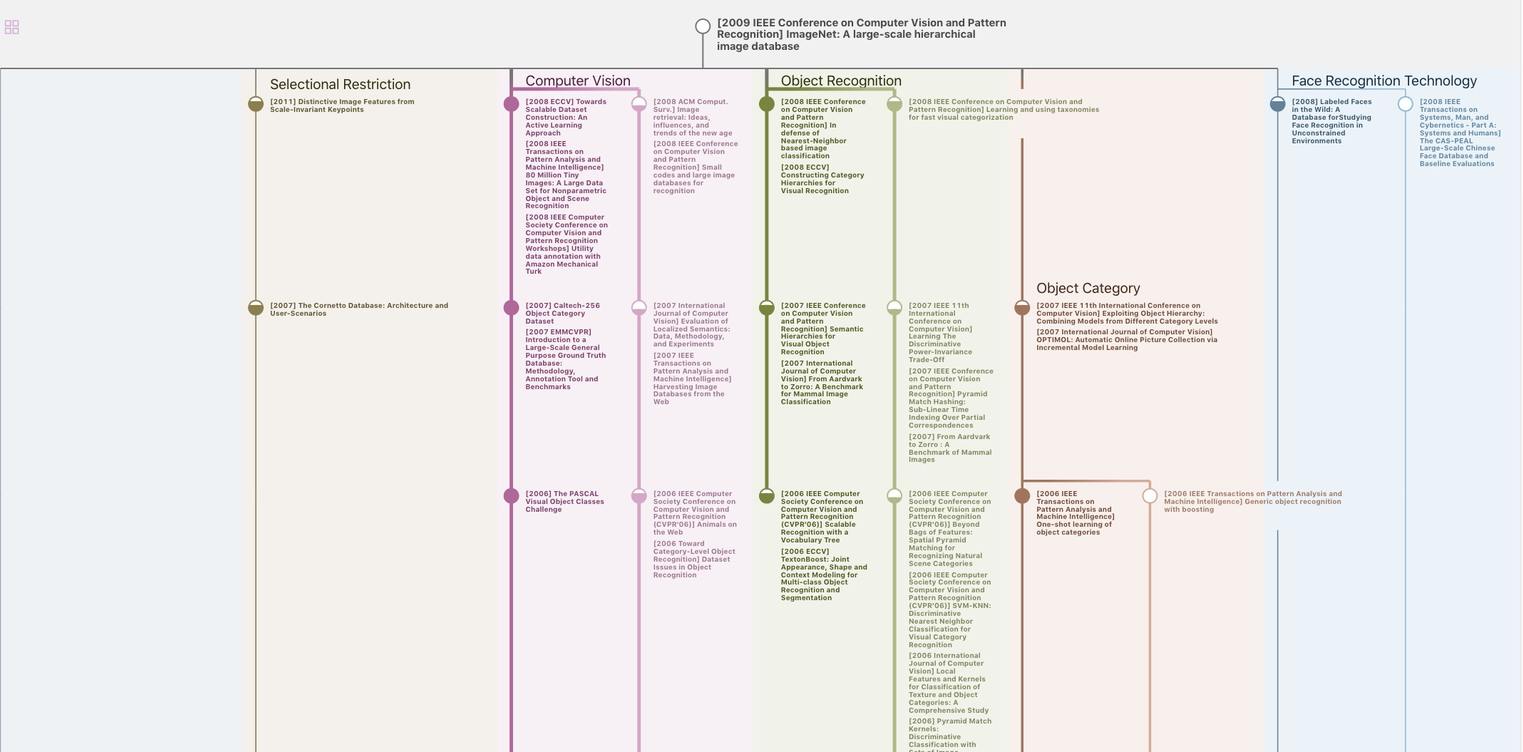
生成溯源树,研究论文发展脉络
Chat Paper
正在生成论文摘要