An Effective Meaningful Way to Evaluate Survival Models
CoRR(2023)
摘要
One straightforward metric to evaluate a survival prediction model is based on the Mean Absolute Error (MAE) -- the average of the absolute difference between the time predicted by the model and the true event time, over all subjects. Unfortunately, this is challenging because, in practice, the test set includes (right) censored individuals, meaning we do not know when a censored individual actually experienced the event. In this paper, we explore various metrics to estimate MAE for survival datasets that include (many) censored individuals. Moreover, we introduce a novel and effective approach for generating realistic semi-synthetic survival datasets to facilitate the evaluation of metrics. Our findings, based on the analysis of the semi-synthetic datasets, reveal that our proposed metric (MAE using pseudo-observations) is able to rank models accurately based on their performance, and often closely matches the true MAE -- in particular, is better than several alternative methods.
更多查看译文
关键词
survival,effective meaningful way,models
AI 理解论文
溯源树
样例
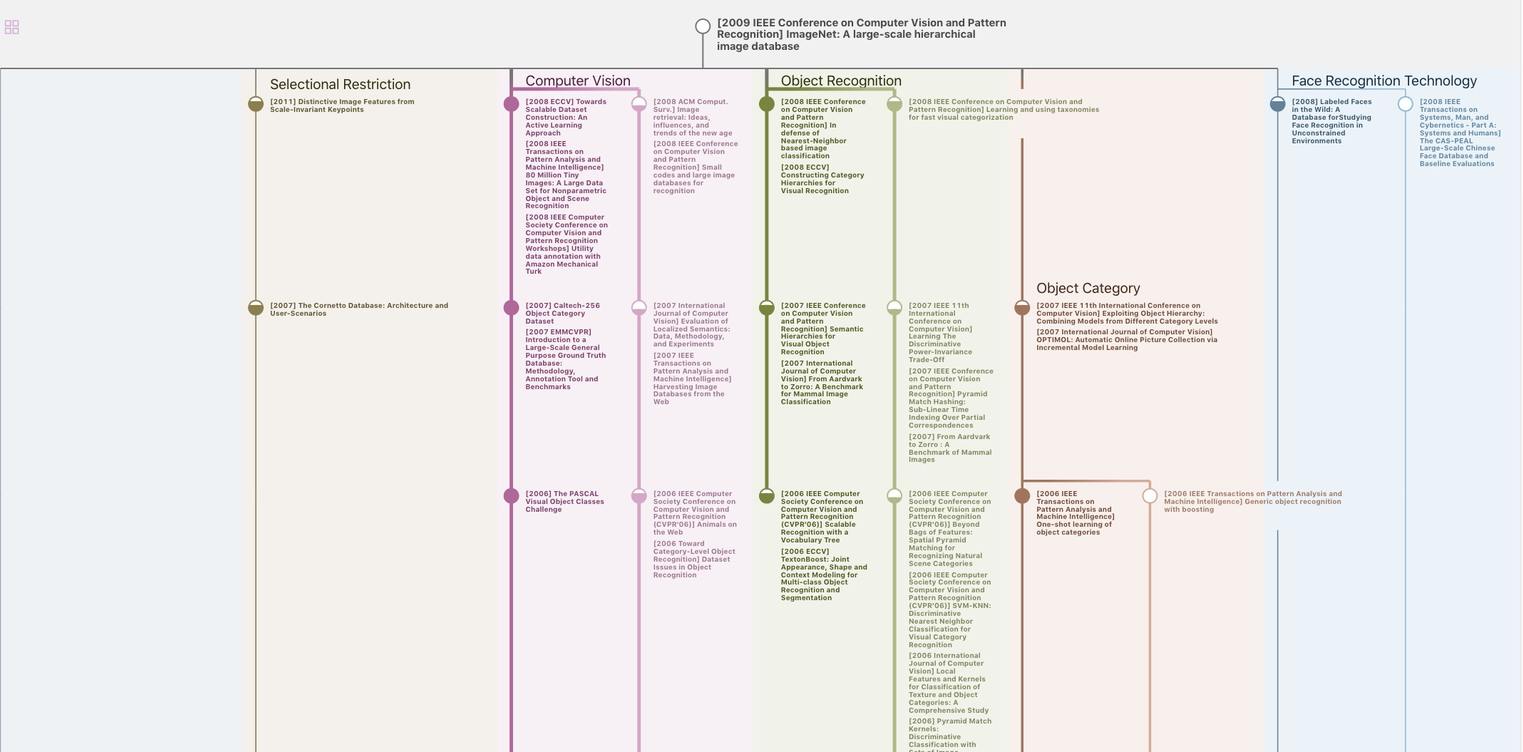
生成溯源树,研究论文发展脉络
Chat Paper
正在生成论文摘要