Federated Learning with Heterogeneous Models for On-device Malware Detection in IoT Networks
2023 DESIGN, AUTOMATION & TEST IN EUROPE CONFERENCE & EXHIBITION, DATE(2023)
摘要
IoT devices have been widely deployed in many applications to facilitate smart technology, increased portability, and seamless connectivity. Despite being widely adopted, security in IoT devices is often considered an afterthought due to resource and cost constraints. Among multiple security threats, malware attacks are observed to be a pivotal threat to IoT devices. Considering the spread of IoT devices and the threats they experience over time, deploying a static malware detector trained offline seems ineffective. On the other hand, on-device learning is an expensive or infeasible option due to the limited available resources on IoT devices. To overcome these challenges, this work employs 'Federated Learning' (FL) which enables timely updates to the malware detection models for increased security while mitigating the high communication or data storage overhead of centralized cloud approaches. Federated learning allows training machine learning models with decentralized data while preserving its privacy by design. However, one of the challenges with the FL is that the on-device models are required to be homogeneous, which may not be true in the case of networked IoT systems. As a panacea, we introduce a methodology to unify the models in the cloud with minimal overheads and an impact on on-device malware detection. We evaluate the proposed technique against homogeneous models in networked IoT systems encompassing Raspberry Pi devices. The experimental results and system efficiency analysis indicate that end-to-end training time is just 1.12x higher than traditional FL, testing latency is 1.63x faster, and malware detection performance is improved by 7% to 13% for resource-constrained IoT devices.
更多查看译文
关键词
security,federated learning,machine learning
AI 理解论文
溯源树
样例
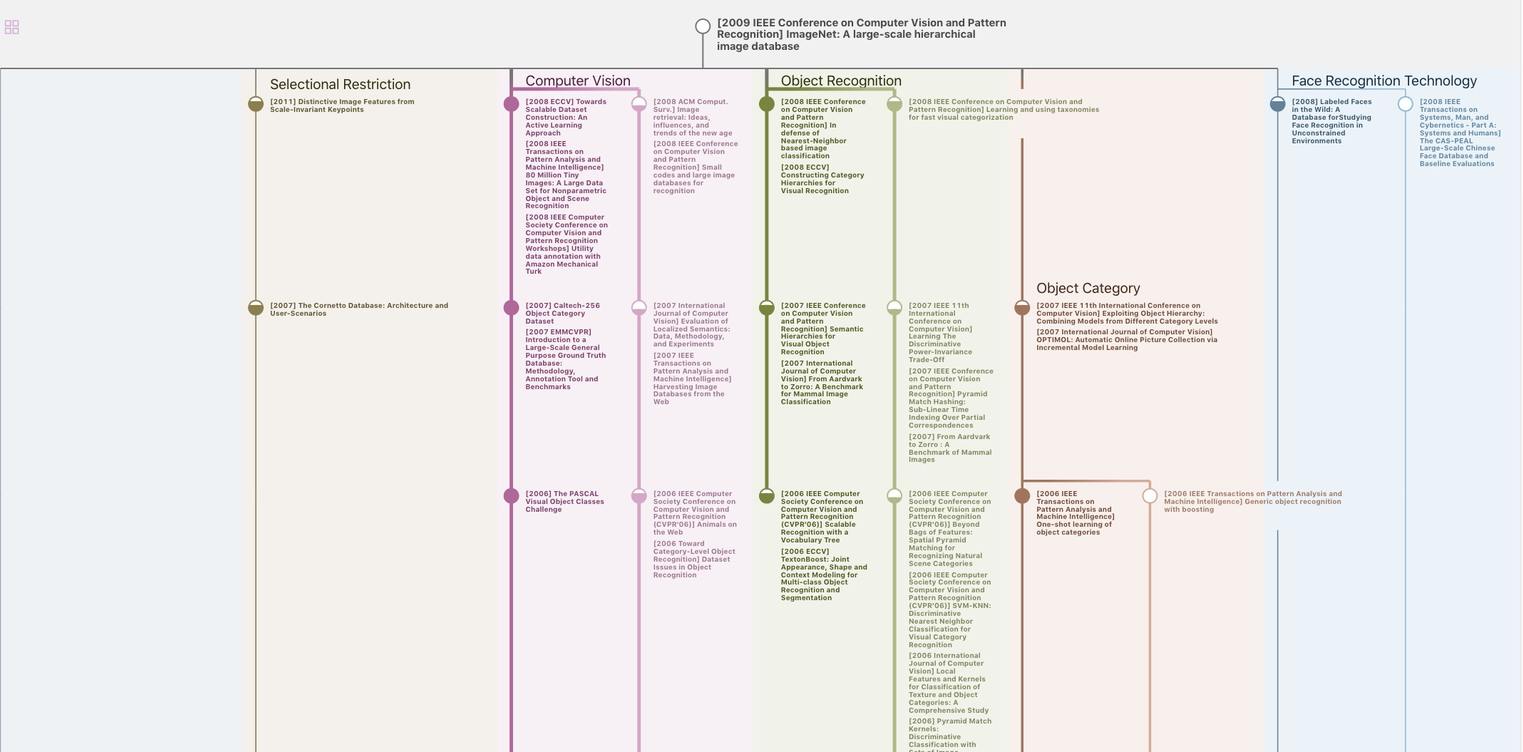
生成溯源树,研究论文发展脉络
Chat Paper
正在生成论文摘要