Explainable for Trustworthy AI.
ACAI(2021)
摘要
Black-box Artificial Intelligence (AI) systems for automated decision making are often based on over (big) human data, map a user’s features into a class or a score without exposing why. This is problematic for the lack of transparency and possible biases inherited by the algorithms from human prejudices and collection artefacts hidden in the training data, leading to unfair or wrong decisions. The future of AI lies in enabling people to collaborate with machines to solve complex problems. This requires good communication, trust, clarity, and understanding, like any efficient collaboration. Explainable AI (XAI) addresses such challenges, and for years different AI communities have studied such topics, leading to different definitions, evaluation protocols, motivations, and results. This chapter provides a reasoned introduction to the work of Explainable AI to date and surveys the literature focusing on symbolic AI-related approaches. We motivate the needs of XAI in real-world and large-scale applications while presenting state-of-the-art techniques and best practices and discussing the many open challenges.
更多查看译文
关键词
explainable
AI 理解论文
溯源树
样例
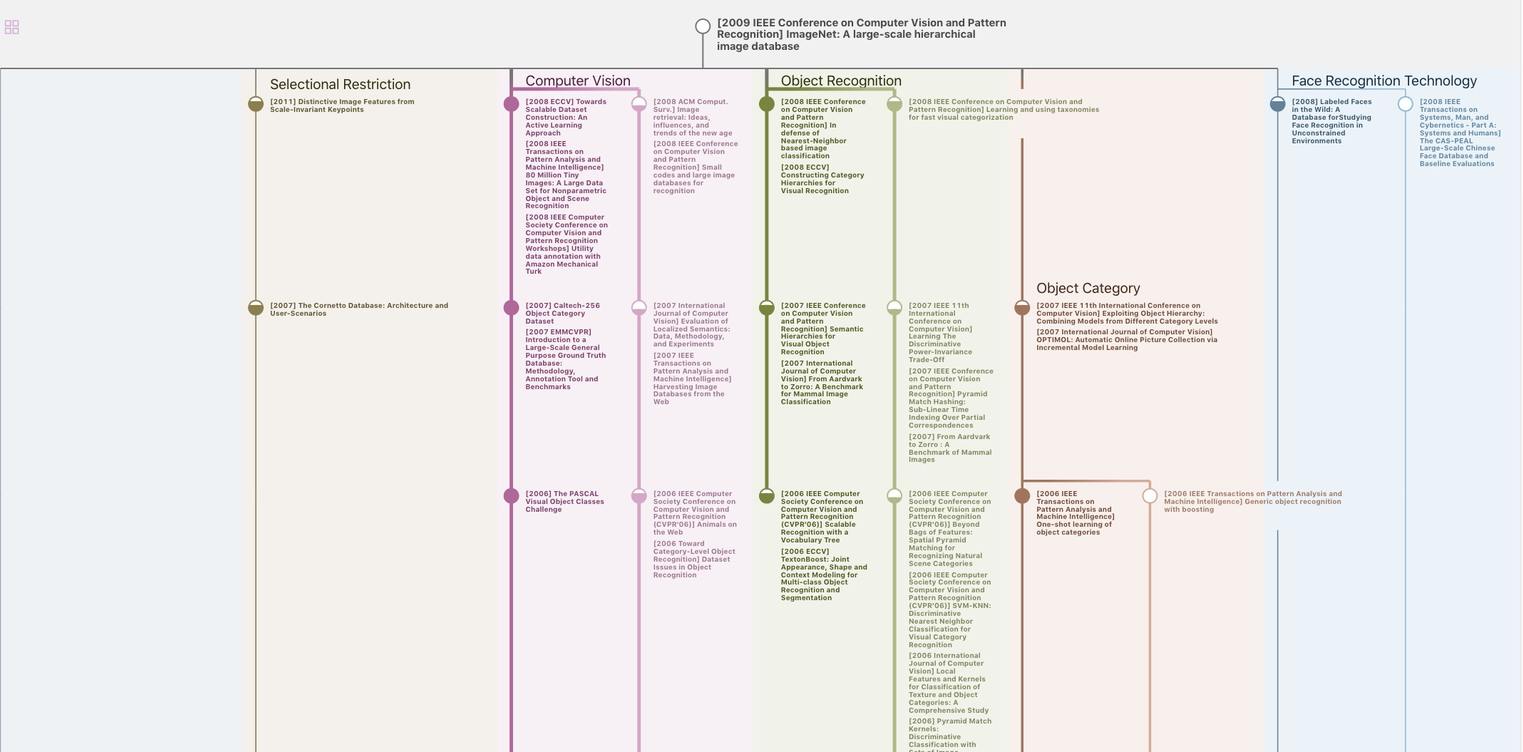
生成溯源树,研究论文发展脉络
Chat Paper
正在生成论文摘要