Pairwise learning for the partial label ranking problem
Pattern Recognit.(2023)
摘要
The partial label ranking problem is a particular preference learning scenario that focuses on learning pref-erence models from data, such that they predict a complete ranking with ties defined over the values of the class variable for a given input instance. This work proposes to transform the rankings into preference relations among pairs of class labels and to learn a standard classifier for each of them. This classifier is then used to estimate the probability of each event from the preference relation between the two com-pared class labels. Finally, the probabilities obtained for each preference comparison are used to compute a preference matrix utilized to solve the corresponding rank aggregation problem and so obtain the ranking among all the class labels. The experimental evaluation shows that the proposed method is ranked ahead of competing algorithms in accuracy while obtaining similar CPU time results.(c) 2023 The Authors. Published by Elsevier Ltd. This is an open access article under the CC BY-NC-ND license ( http://creativecommons.org/licenses/by-nc-nd/4.0/ )
更多查看译文
关键词
label,learning
AI 理解论文
溯源树
样例
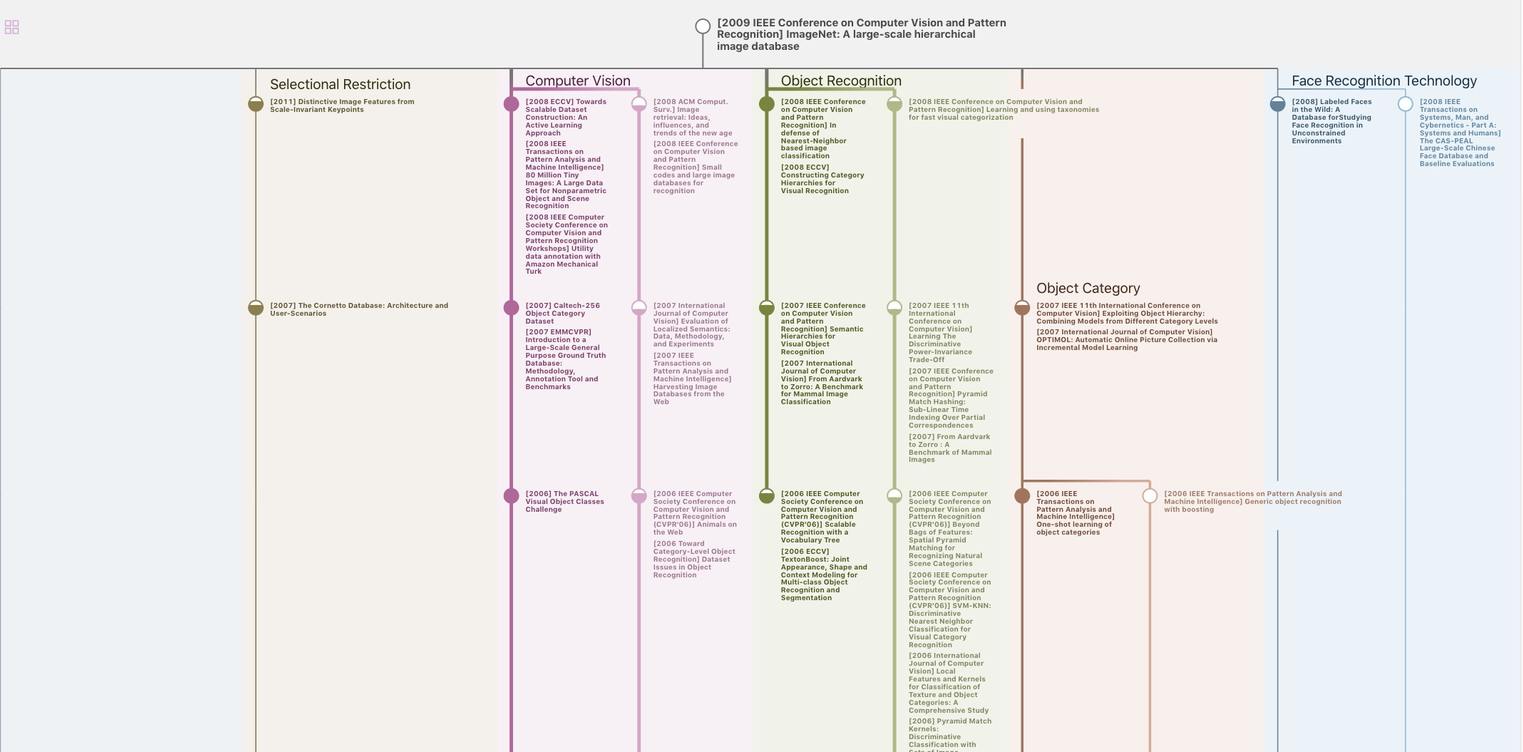
生成溯源树,研究论文发展脉络
Chat Paper
正在生成论文摘要