Training changes the EEG complexity and functional connectivity of precise timing prediction*
NER(2023)
摘要
Precise timing prediction is the ability to estimate time in millisecond timescale, it can speed up behavior, optimize perception, benefit adaptive behaviors. Behavioral studies have demonstrated training can improve the performance of precise timing prediction. However, neural evidence is still lacking in describing how training changes the neural characteristics of precise timing prediction. This study designed a cue-tapping (task1)/ timing-in-mind (task2) experiment, collected behavioral and electroencephalogram (EEG) data of 24 subjects in both before and after training period. Sample entropy (SampEn) and Lempel-Ziv complexity (LZC) were calculated to measure EEG complexity, functional connectivity based on phase locking value was also involved. Consequently, behavioral results showed that error time declined and accuracy rate increased as training progressed. SampEn was much smaller after training in almost all frequency-bands in task1, and reduced in task2 as well. LZC showed a decreased tendency after training, but no statistical significance was found. Moreover, after training, much stronger functional connectivity was found in low frequency-band in both tasks. The results can shed light on the modeling of training and precise predictive timing.
更多查看译文
关键词
Training, Timing prediction, Sample entropy, Lempel-Ziv complexity (LZC), Phase locking value(PLV)
AI 理解论文
溯源树
样例
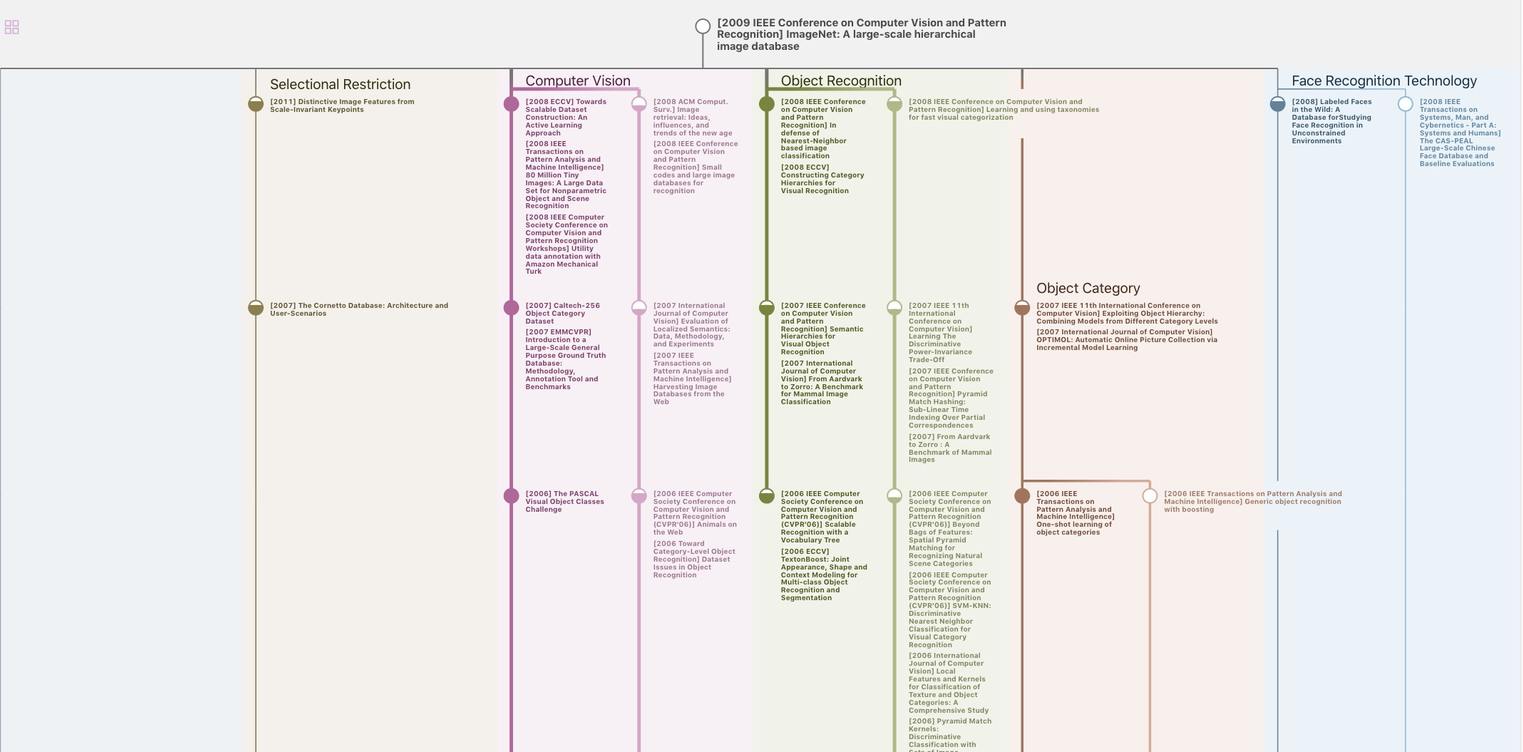
生成溯源树,研究论文发展脉络
Chat Paper
正在生成论文摘要