A Bayesian sparse factor model with adaptive posterior concentration
CoRR(2023)
摘要
In this paper, we propose a new Bayesian inference method for a high-dimensional sparse factor model that allows both the factor dimensionality and the sparse structure of the loading matrix to be inferred. The novelty is to introduce a certain dependence between the sparsity level and the factor dimensionality, which leads to adaptive posterior concentration while keeping computational tractability. We show that the posterior distribution asymptotically concentrates on the true factor dimensionality, and more importantly, this posterior consistency is adaptive to the sparsity level of the true loading matrix and the noise variance. We also prove that the proposed Bayesian model attains the optimal detection rate of the factor dimensionality in a more general situation than those found in the literature. Moreover, we obtain a near-optimal posterior concentration rate of the covariance matrix. Numerical studies are conducted and show the superiority of the proposed method compared with other competitors.
更多查看译文
关键词
bayesian sparse factor model,adaptive posterior concentration
AI 理解论文
溯源树
样例
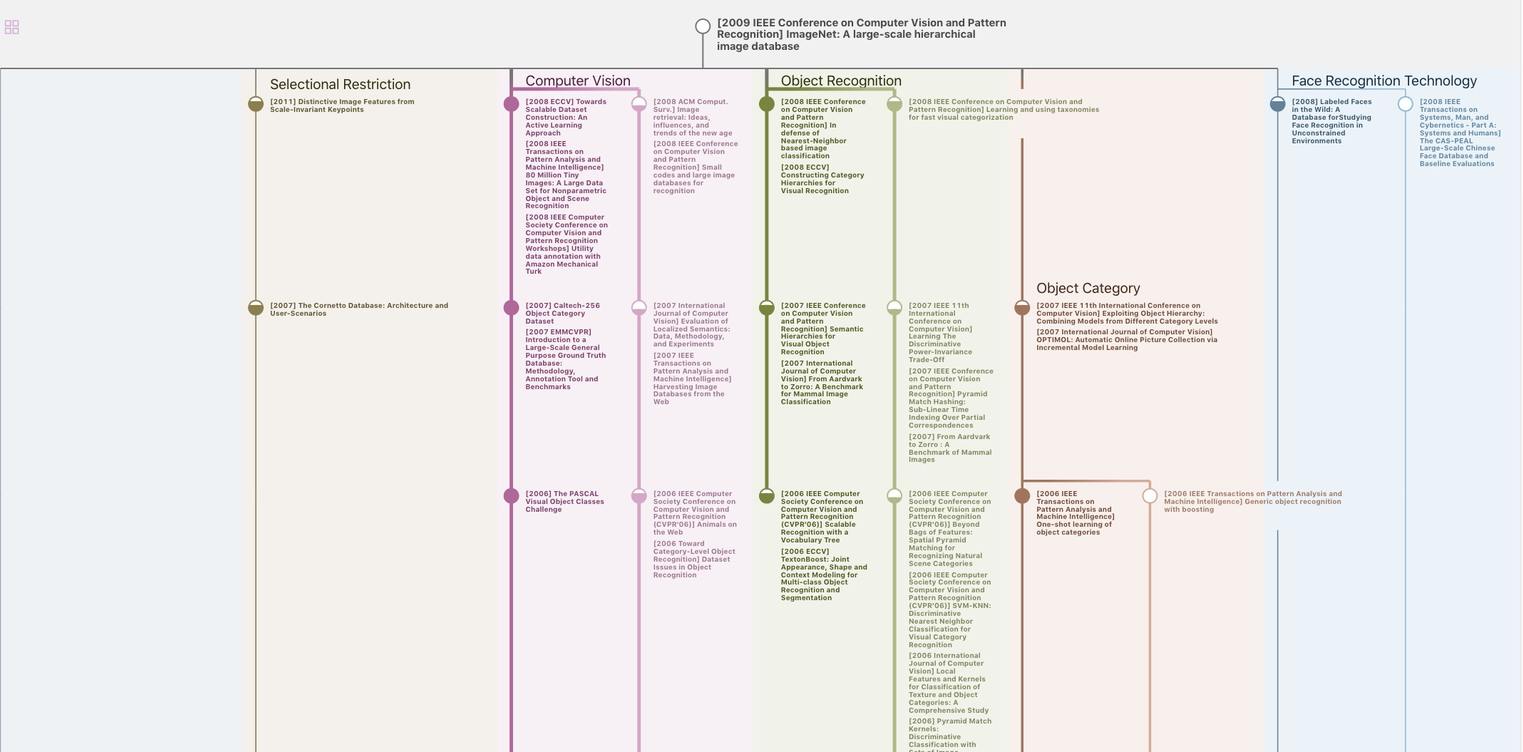
生成溯源树,研究论文发展脉络
Chat Paper
正在生成论文摘要