Human-Machine Comparison for Cross-Race Face Verification: Race Bias at the Upper Limits of Performance?
CoRR(2023)
摘要
Face recognition algorithms perform more accurately than humans in some cases, though humans and machines both show race-based accuracy differences. As algorithms continue to improve, it is important to continually assess their race bias relative to humans. We constructed a challenging test of 'cross-race' face verification and used it to compare humans and two state-of-the-art face recognition systems. Pairs of same- and different-identity faces of White and Black individuals were selected to be difficult for humans and an open-source implementation of the ArcFace face recognition algorithm from 2019 (5). Human participants (54 Black; 51 White) judged whether face pairs showed the same identity or different identities on a 7-point Likert-type scale. Two top-performing face recognition systems from the Face Recognition Vendor Test-ongoing performed the same test (7). By design, the test proved challenging for humans as a group, who performed above chance, but far less than perfect. Both state-of-the-art face recognition systems scored perfectly (no errors), consequently with equal accuracy for both races. We conclude that state-of-the-art systems for identity verification between two frontal face images of Black and White individuals can surpass the general population. Whether this result generalizes to challenging in-the-wild images is a pressing concern for deploying face recognition systems in unconstrained environments.
更多查看译文
关键词
cross-race bias,face,human-machine
AI 理解论文
溯源树
样例
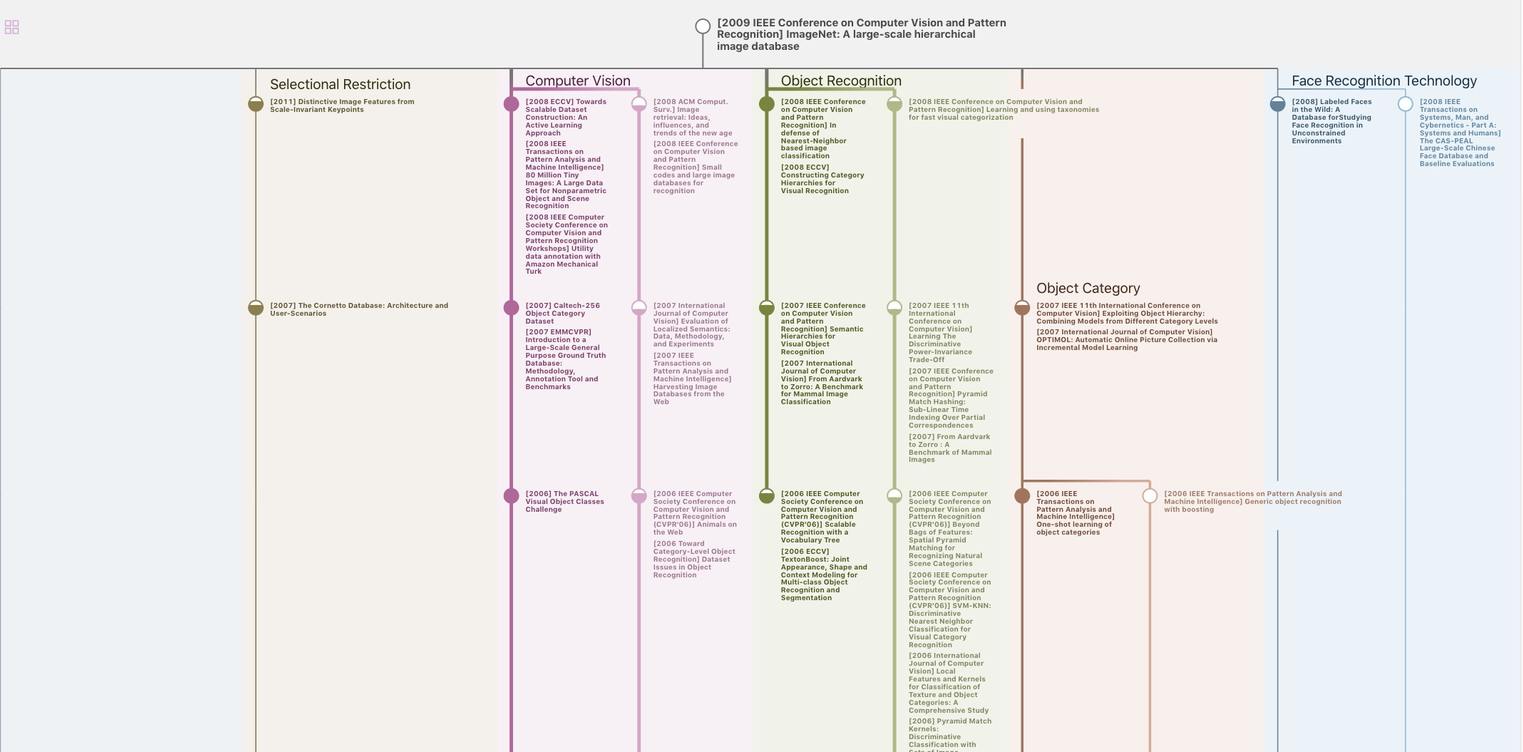
生成溯源树,研究论文发展脉络
Chat Paper
正在生成论文摘要