Enhancing DNN Training Efficiency Via Dynamic Asymmetric Architecture
IEEE Computer Architecture Letters(2023)
摘要
Deep neural networks (DNNs) require abundant multiply-and-accumulate (MAC) operations. Thanks to DNNs’ ability to accommodate noise, some of the computational burden is commonly mitigated by quantization–that is, by using lower precision floating-point operations. Layer granularity is the preferred method, as it is easily mapped to commodity hardware. In this paper, we propose Dynamic Asymmetric Architecture (DAA), in which the micro-architecture decides what the precision of each MAC operation should be during runtime. We demonstrate a DAA with two data streams and a value-based controller that decides which data stream deserves the higher precision resource. We evaluate this mechanism in terms of accuracy on a number of convolutional neural networks (CNNs) and demonstrate its feasibility on top of a systolic array. Our experimental analysis shows that DAA potentially achieves 2x throughput improvement for ResNet-18 while saving 35% of the energy with less than 0.5% degradation in accuracy.
更多查看译文
关键词
dnn training efficiency,dynamic
AI 理解论文
溯源树
样例
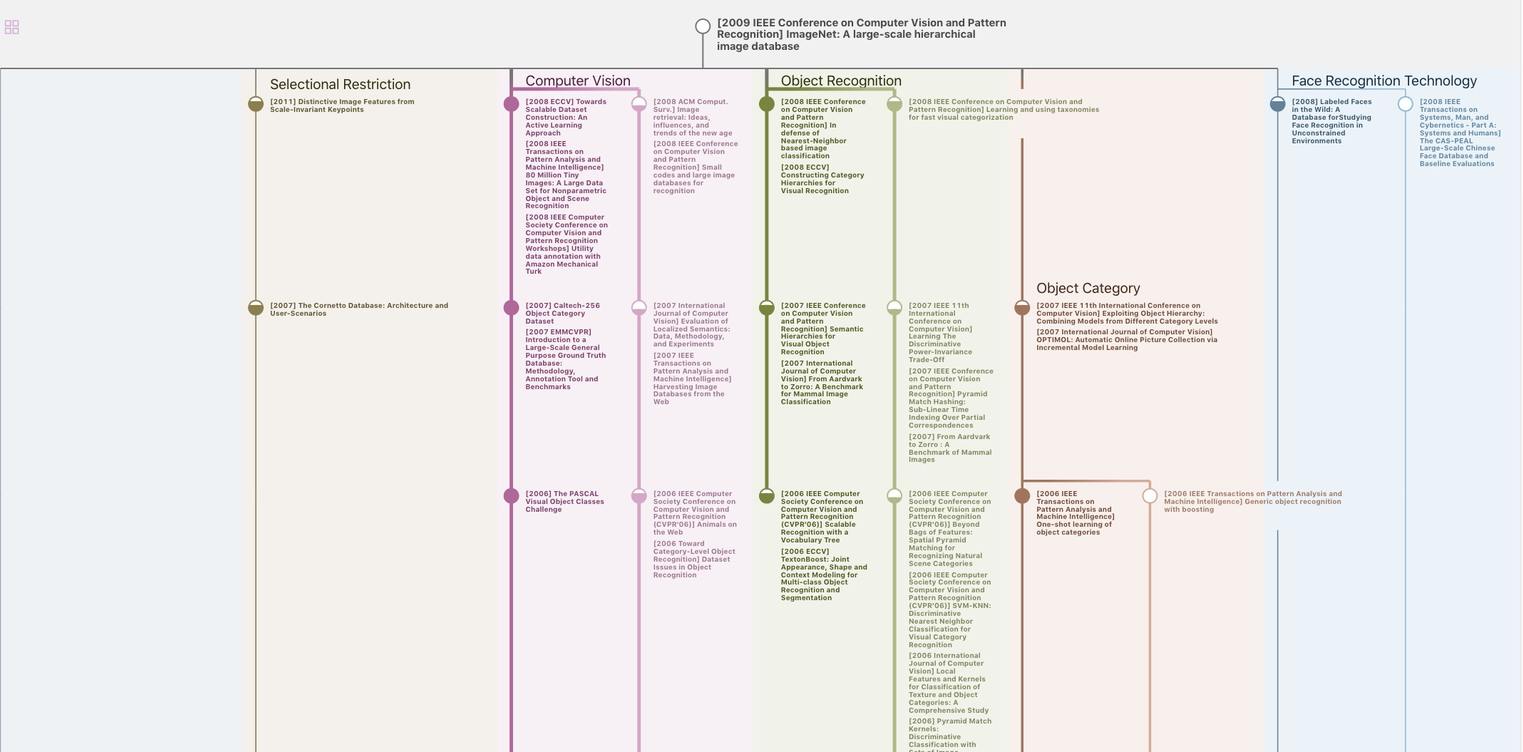
生成溯源树,研究论文发展脉络
Chat Paper
正在生成论文摘要