Ner4Opt: Named Entity Recognition for Optimization Modelling from Natural Language
Integration of Constraint Programming, Artificial Intelligence, and Operations Research(2023)
摘要
Solving combinatorial optimization problems involves a two-stage process that follows the model-and-run approach. First, a user is responsible for formulating the problem at hand as an optimization model, and then, given the model, a solver is responsible for finding the solution. While optimization technology has enjoyed tremendous theoretical and practical advances, the overall process has remained the same for decades. To date, transforming problem descriptions into optimization models remains a barrier to entry. To alleviate users from the cognitive task of modeling, we study named entity recognition to capture components of optimization models such as the objective, variables, and constraints from free-form natural language text, and coin this problem as Ner4Opt. We show how to solve Ner4Opt using classical techniques based on morphological and grammatical properties and modern methods leveraging pre-trained large language models and fine-tuning transformers architecture with optimization-specific corpora. For best performance, we present their hybridization combined with feature engineering and data augmentation to exploit the language of optimization problems. We improve over the state-of-the-art for annotated linear programming word problems, identify several next steps and discuss important open problems toward automated modeling.
更多查看译文
关键词
Optimization Modeling, Named Entity Recognition, Natural Language Processing
AI 理解论文
溯源树
样例
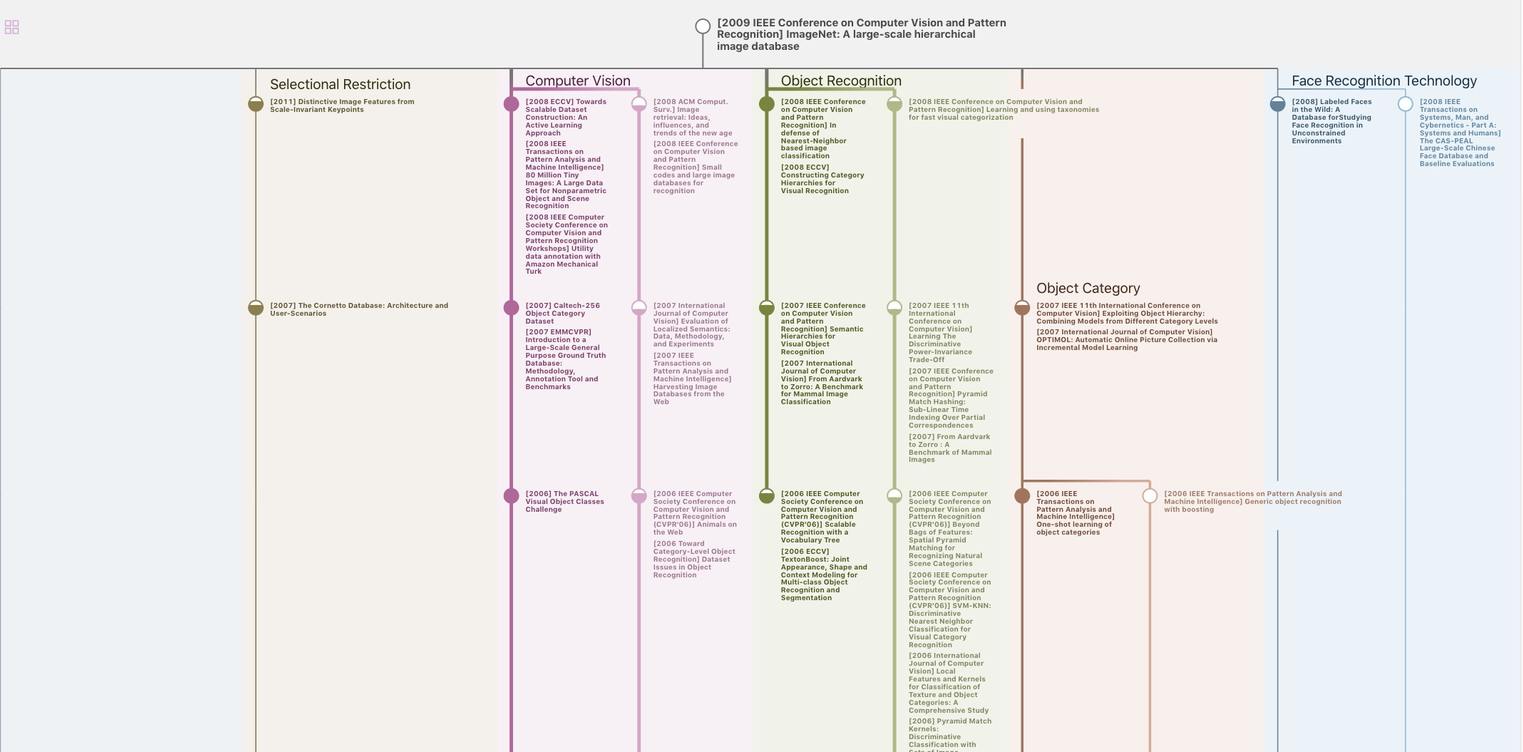
生成溯源树,研究论文发展脉络
Chat Paper
正在生成论文摘要