Improved risk minimization algorithms for technology-assisted review
Intelligent Systems with Applications(2023)
摘要
MINECORE is a recently proposed decision-theoretic algorithm for technology-assisted review that attempts to minimise the expected costs of review for responsiveness and privilege in e-discovery. In MINECORE, two probabilistic classifiers that classify documents by responsiveness and by privilege, respectively, generate posterior probabilities. These latter are fed to an algorithm that returns as output, after applying risk minimization, two ranked lists, which indicate exactly which documents the annotators should review for responsiveness and which documents they should review for privilege. In this paper we attempt to find out if the performance of MINECORE can be improved (a) by using, for the purpose of training the two classifiers, active learning (implemented either via relevance sampling, or via uncertainty sampling, or via a combination of them) instead of passive learning, and (b) by using the Saerens-Latinne-Decaestecker algorithm to improve the quality of the posterior probabilities that MINECORE receives as input. We address these two research questions by carrying out extensive experiments on the RCV1-v2 benchmark. We make publicly available the code and data for reproducing all our experiments.
更多查看译文
关键词
Technology-assisted review,E-discovery,Risk minimization,Dataset shift,MINECORE
AI 理解论文
溯源树
样例
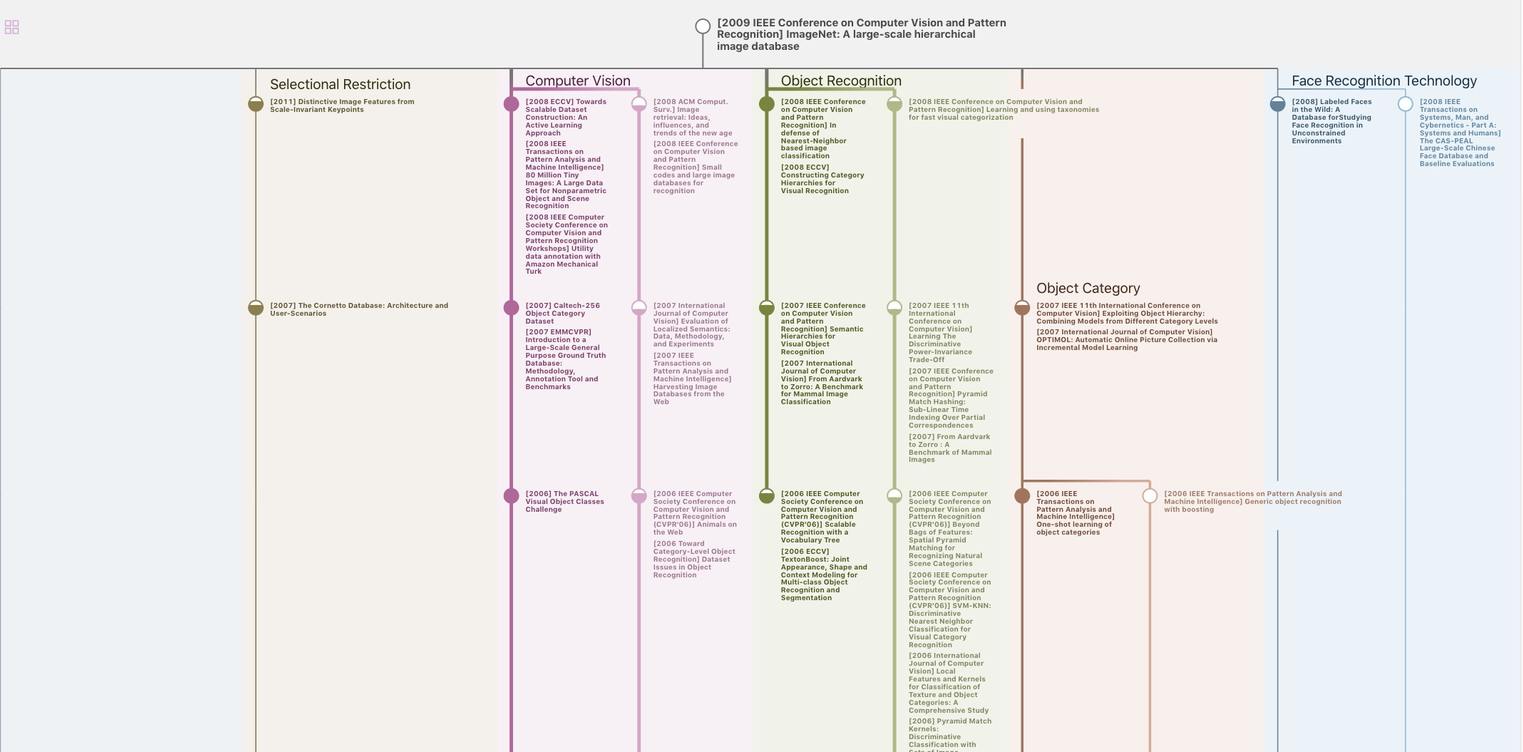
生成溯源树,研究论文发展脉络
Chat Paper
正在生成论文摘要