Taxonomy Expansion for Named Entity Recognition.
CoRR(2023)
摘要
Training a Named Entity Recognition (NER) model often involves fixing a taxonomy of entity types. However, requirements evolve and we might need the NER model to recognize additional entity types. A simple approach is to re-annotate entire dataset with both existing and additional entity types and then train the model on the re-annotated dataset. However, this is an extremely laborious task. To remedy this, we propose a novel approach called Partial Label Model (PLM) that uses only partially annotated datasets. We experiment with 6 diverse datasets and show that PLM consistently performs better than most other approaches (0.5 - 2.5 F1), including in novel settings for taxonomy expansion not considered in prior work. The gap between PLM and all other approaches is especially large in settings where there is limited data available for the additional entity types (as much as 11 F1), thus suggesting a more cost effective approaches to taxonomy expansion.
更多查看译文
关键词
taxonomy,expansion
AI 理解论文
溯源树
样例
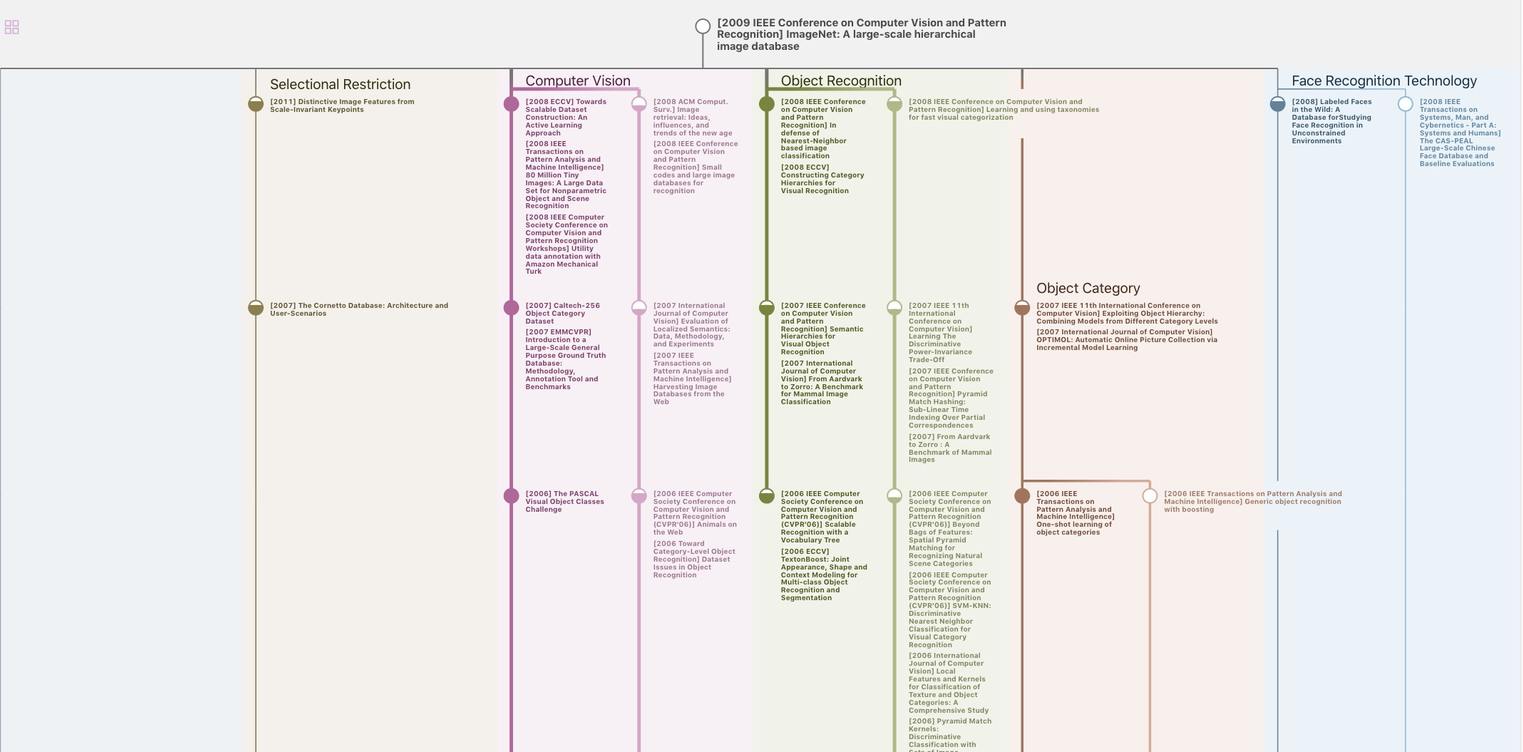
生成溯源树,研究论文发展脉络
Chat Paper
正在生成论文摘要