When Federated Recommendation Meets Cold-Start Problem: Separating Item Attributes and User Interactions
WWW 2024(2024)
摘要
Federated recommendation system usually trains a global model on the server
without direct access to users' private data on their own devices. However,
this separation of the recommendation model and users' private data poses a
challenge in providing quality service, particularly when it comes to new
items, namely cold-start recommendations in federated settings. This paper
introduces a novel method called Item-aligned Federated Aggregation (IFedRec)
to address this challenge. It is the first research work in federated
recommendation to specifically study the cold-start scenario. The proposed
method learns two sets of item representations by leveraging item attributes
and interaction records simultaneously. Additionally, an item representation
alignment mechanism is designed to align two item representations and learn the
meta attribute network at the server within a federated learning framework.
Experiments on four benchmark datasets demonstrate IFedRec's superior
performance for cold-start scenarios. Furthermore, we also verify IFedRec owns
good robustness when the system faces limited client participation and noise
injection, which brings promising practical application potential in
privacy-protection enhanced federated recommendation systems. The
implementation code is available
更多查看译文
AI 理解论文
溯源树
样例
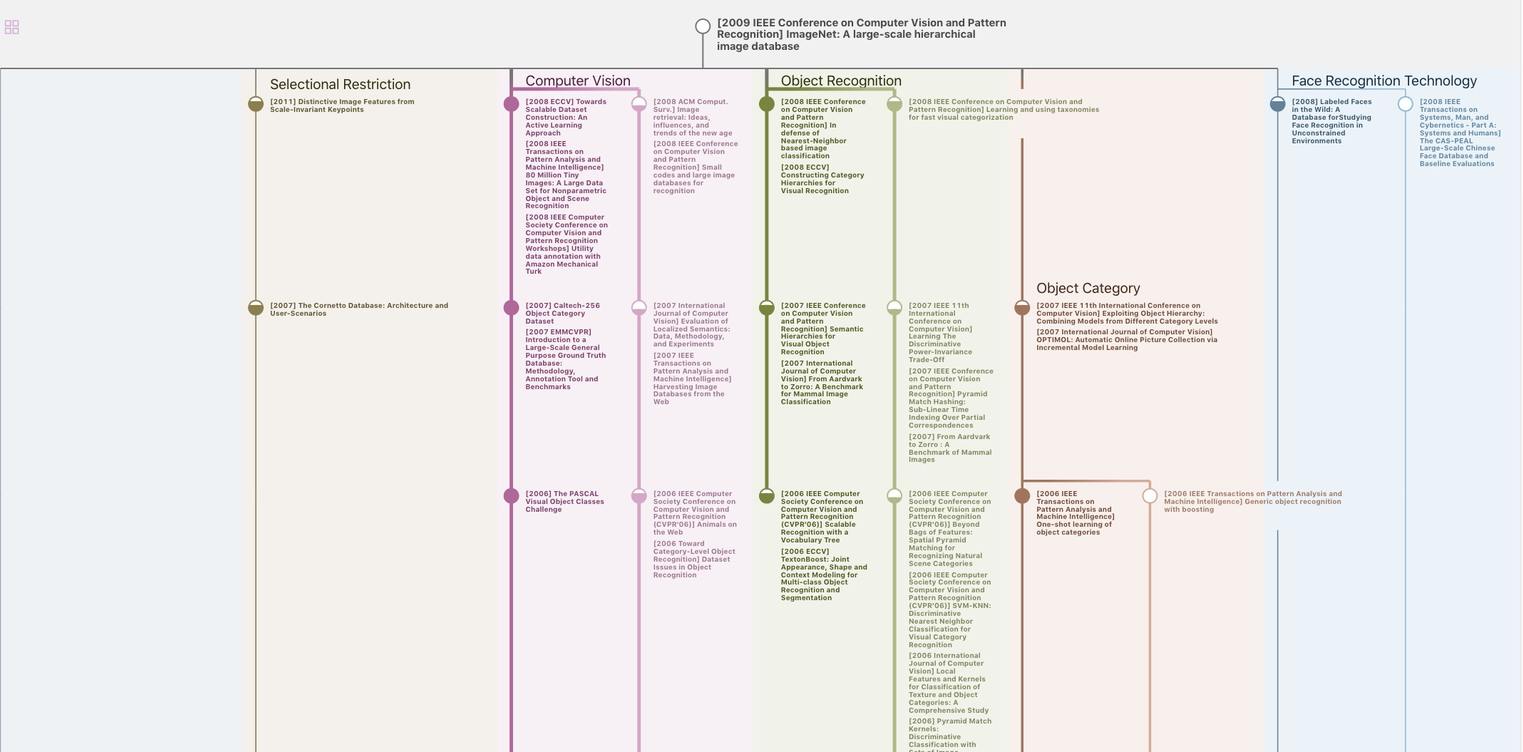
生成溯源树,研究论文发展脉络
Chat Paper
正在生成论文摘要