Progressive Learning of 3D Reconstruction Network From 2D GAN Data
IEEE TRANSACTIONS ON PATTERN ANALYSIS AND MACHINE INTELLIGENCE(2024)
摘要
This paper presents a method to reconstruct high-quality textured 3D models from single images. Current methods rely on datasets with expensive annotations; multi-view images and their camera parameters. Our method relies on GAN generated multi-view image datasets which have a negligible annotation cost. However, they are not strictly multi-view consistent and sometimes GANs output distorted images. This results in degraded reconstruction qualities. In this work, to overcome these limitations of generated datasets, we have two main contributions which lead us to achieve state-of-the-art results on challenging objects: 1) A robust multi-stage learning scheme that gradually relies more on the models own predictions when calculating losses and 2) A novel adversarial learning pipeline with online pseudo-ground truth generations to achieve fine details. Our work provides a bridge from 2D supervisions of GAN models to 3D reconstruction models and removes the expensive annotation efforts. We show significant improvements over previous methods whether they were trained on GAN generated multi-view images or on real images with expensive annotations.
更多查看译文
关键词
3D reconstruction,3D texture learning,generative adversarial networks,single-image inference
AI 理解论文
溯源树
样例
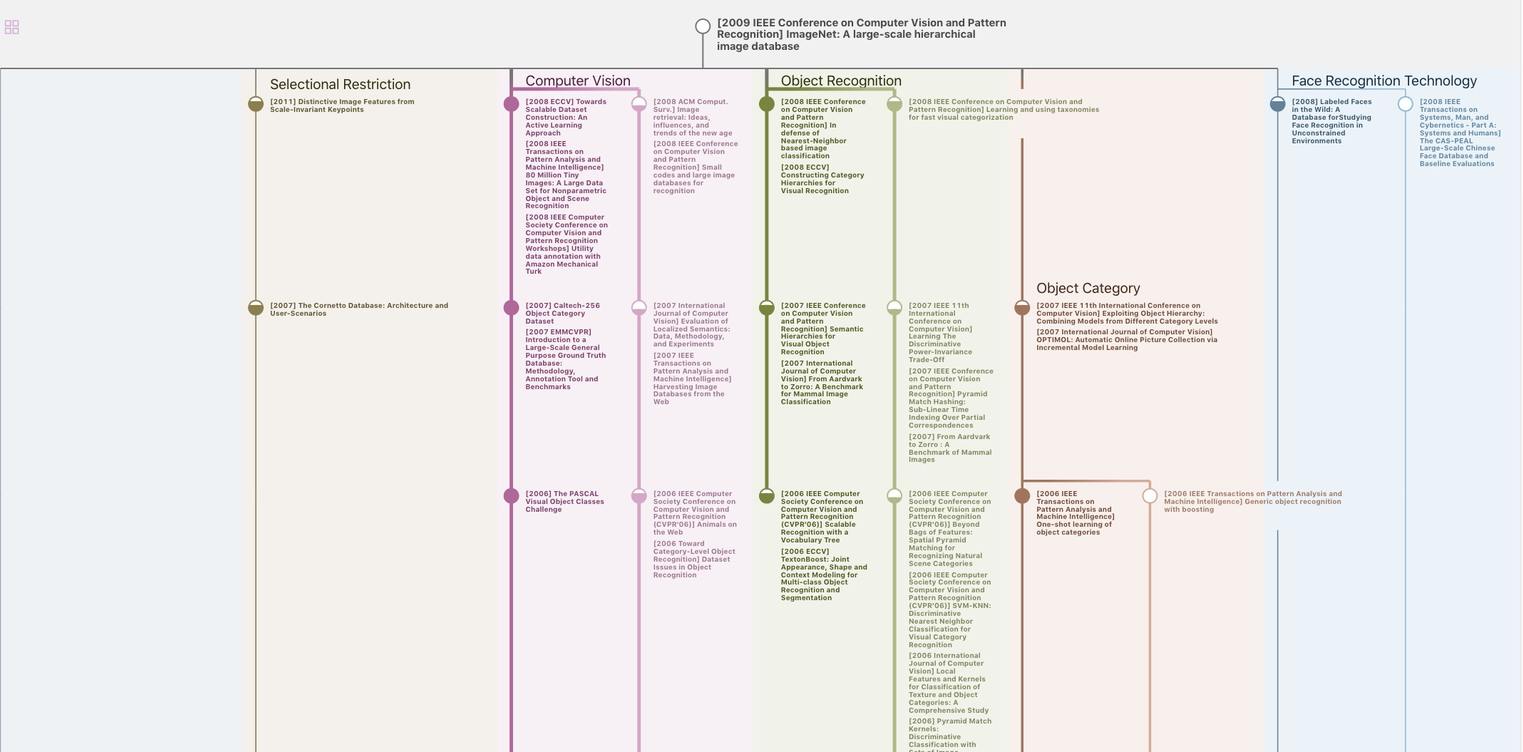
生成溯源树,研究论文发展脉络
Chat Paper
正在生成论文摘要