SHoP: A Deep Learning Framework for Solving High-Order Partial Differential Equations
AAAI 2024(2024)
摘要
Solving partial differential equations (PDEs) has been a fundamental problem in computational science and of wide applications for both scientific and engineering research. Due to its universal approximation property, neural network is widely used to approximate the solutions of PDEs. However, existing works are incapable of solving high-order PDEs due to insufficient calculation accuracy of higher-order derivatives, and the final network is a black box without explicit explanation. To address these issues, we propose a deep learning framework to solve high-order PDEs, named SHoP. Specifically, we derive the high-order derivative rule for neural network, to get the derivatives quickly and accurately; moreover, we expand the network into a Taylor series, providing an explicit solution for the PDEs. We conduct experimental validations four high-order PDEs with different dimensions, showing that we can solve high-order PDEs efficiently and accurately. The source code can be found at https://github.com/HarryPotterXTX/SHoP.git.
更多查看译文
关键词
ML: Deep Learning Theory,ML: Applications,ML: Deep Learning Algorithms,ML: Transparent, Interpretable, Explainable ML
AI 理解论文
溯源树
样例
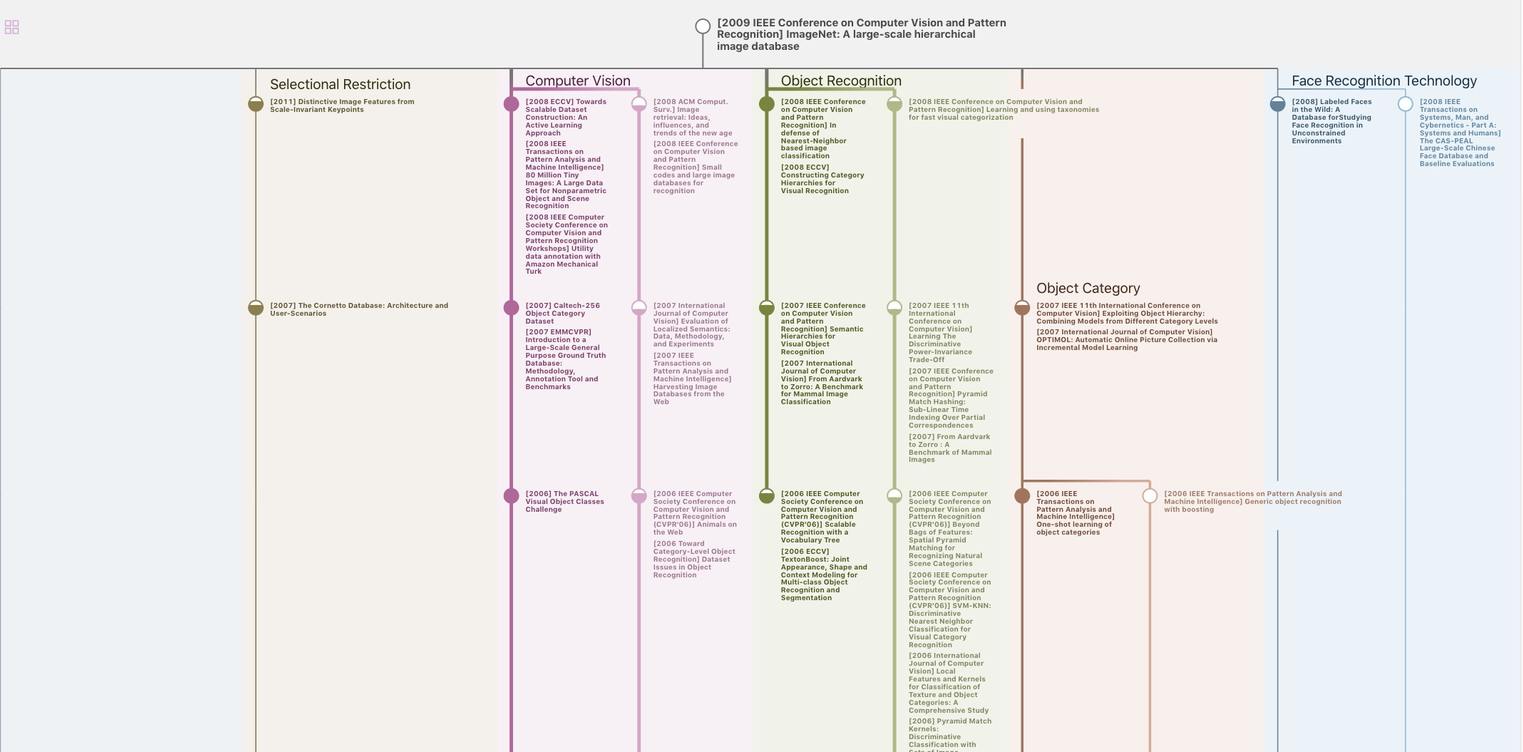
生成溯源树,研究论文发展脉络
Chat Paper
正在生成论文摘要