Intent-Aware Propensity Estimation via Click Pattern Stratification
COMPANION OF THE WORLD WIDE WEB CONFERENCE, WWW 2023(2023)
摘要
Counterfactual learning to rank via inverse propensity weighting is the most popular approach to train ranking models using biased implicit user feedback from logged search data. Standard click propensity estimation techniques rely on simple models of user browsing behavior that primarily account for the attributes of the presentation context that affect whether the relevance of an item to the search context is observed. Most notably, the inherent effect of the listwise presentation of the items on users' propensity for engagement is captured in the position of the presented items on the search result page. In this work, we enrich this position bias based click propensity model by proposing an observation model that further incorporates the underlying search intent, as reflected in the user's click pattern in the search context. Our approach does not require an intent prediction model based on the content of the search context. Instead, we rely on a simple, yet effective, non-causal estimate of the user's browsing intent from the number of click events in the search context. We empirically characterize the distinct rank decay patterns of the estimated click propensities in the characterized intent classes. In particular, we demonstrate a sharper decay of click propensities in top ranks for the intent class identified by sparse user clicks and the higher likelihood of observing clicks in lower ranks for the intent class identified by higher number of user clicks. We show that the proposed intent-aware propensity estimation technique helps with training ranking models with more effective personalization and generalization power through empirical results for a ranking task in a major e-commerce platform.
更多查看译文
关键词
learning to rank,unbiased learning,weak supervision
AI 理解论文
溯源树
样例
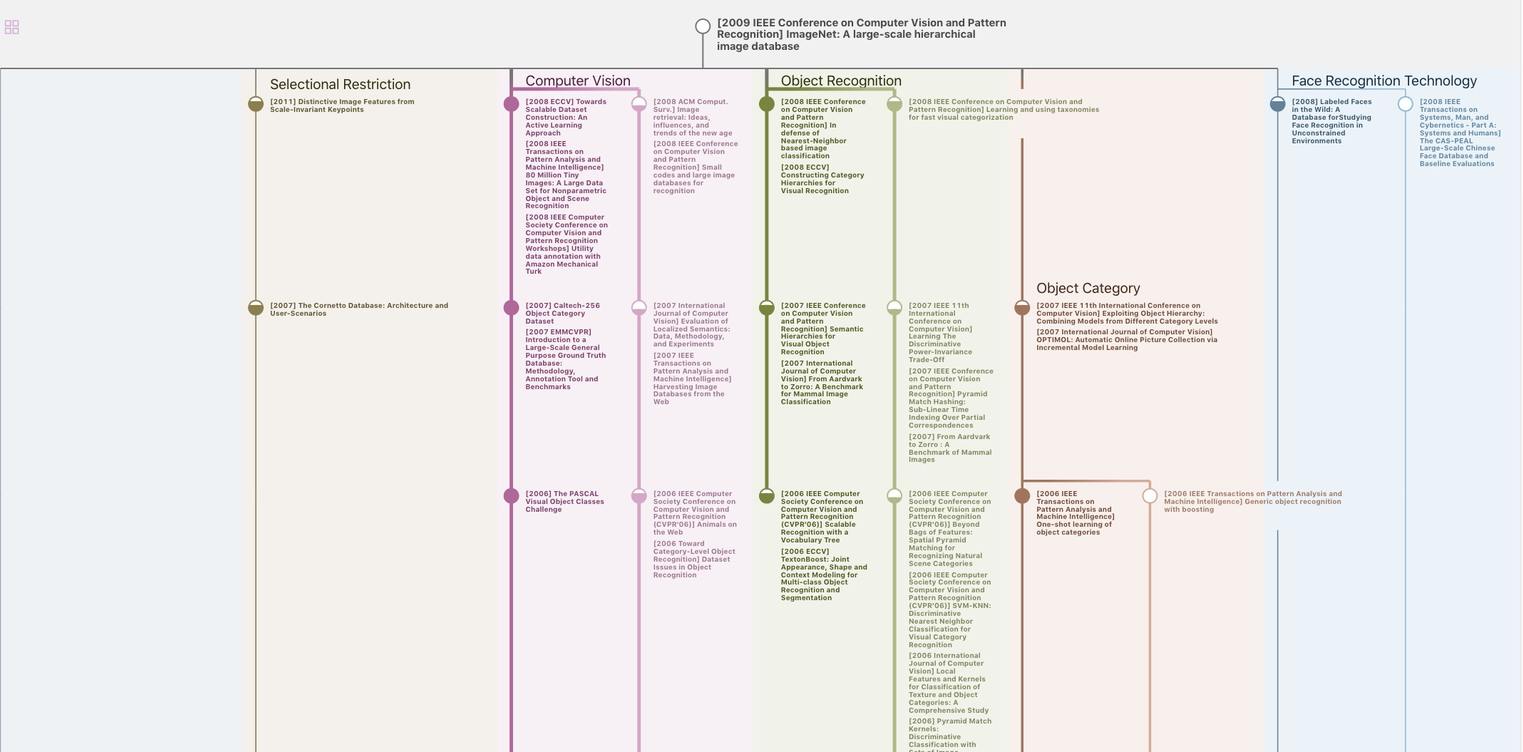
生成溯源树,研究论文发展脉络
Chat Paper
正在生成论文摘要