Bootstrapping Contrastive Learning Enhanced Music Cold-Start Matching
COMPANION OF THE WORLD WIDE WEB CONFERENCE, WWW 2023(2023)
摘要
We study a particular matching task we call Music Cold-Start Matching. In short, given a cold-start song request, we expect to retrieve songs with similar audiences and then fastly push the cold-start song to the audiences of the retrieved songs to warm up it. However, there are hardly any studies done on this task. Therefore, in this paper, we will formalize the problem of Music Cold-Start Matching detailedly and give a scheme. During the offline training, we attempt to learn high-quality song representations based on song content features. But, we find supervision signals typically follow power-law distribution causing skewed representation learning. To address this issue, we propose a novel contrastive learning paradigm named Bootstrapping Contrastive Learning (BCL) to enhance the quality of learned representations by exerting contrastive regularization. During the online serving, to locate the target audiences more accurately, we propose Clustering-based Audience Targeting (CAT) that clusters audience representations to acquire a few cluster centroids and then locate the target audiences by measuring the relevance between the audience representations and the cluster centroids. Extensive experiments on the offline dataset and online system demonstrate the effectiveness and efficiency of our method. Currently, we have deployed it on NetEase Cloud Music, affecting millions of users.
更多查看译文
关键词
Music Cold-Start Matching,Bootstrapping Contrastive Learning,Clustering-based Audience Targeting
AI 理解论文
溯源树
样例
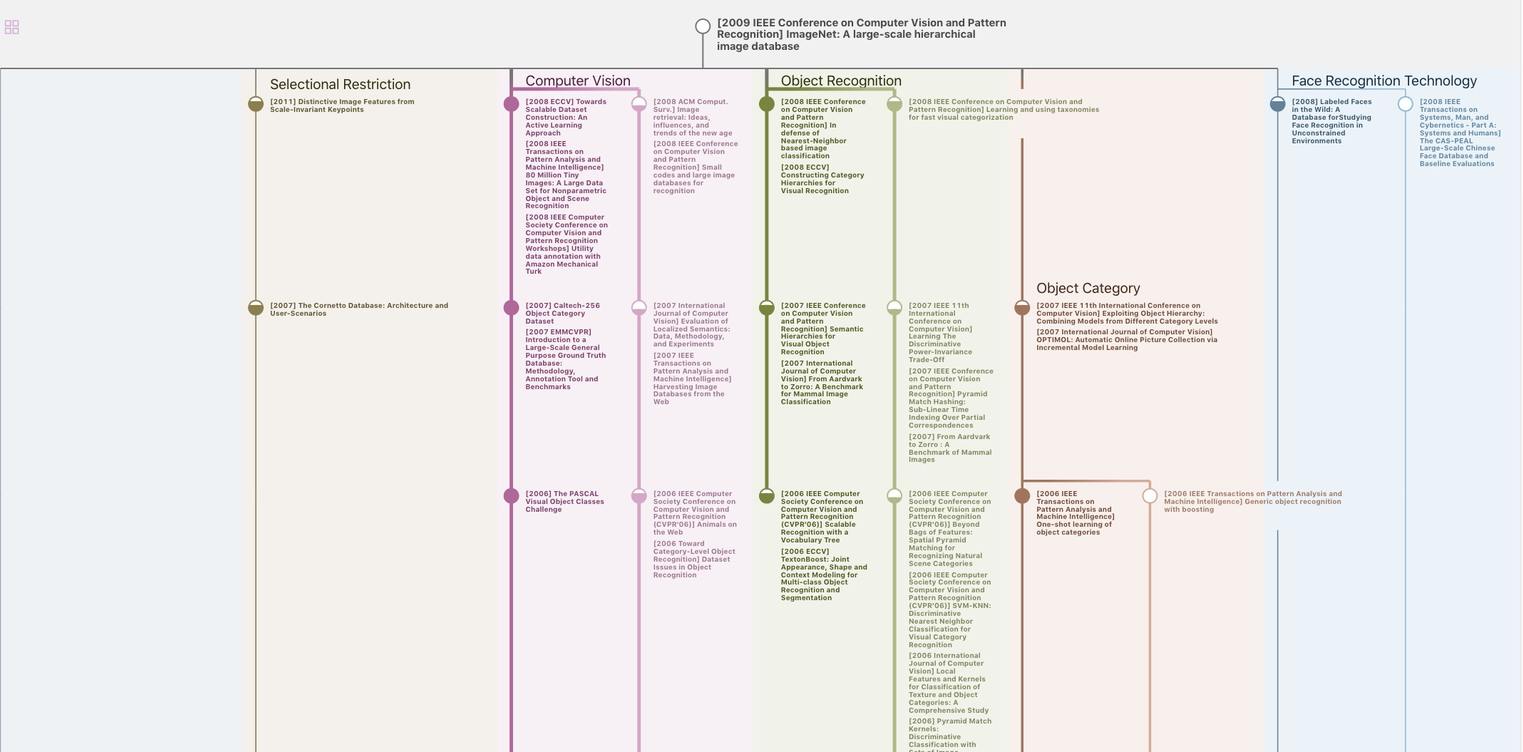
生成溯源树,研究论文发展脉络
Chat Paper
正在生成论文摘要