Sampling recovery in the uniform norm
CoRR(2023)
摘要
We study the recovery of functions in the uniform norm based on function evaluations. We obtain worst case error bounds for general classes of functions, also in $L_p$-norm, in terms of the best $L_2$-approximation from a given nested sequence of subspaces combined with bounds on the the Christoffel function of these subspaces. Our results imply that linear sampling algorithms are optimal (up to constants) among all algorithms using arbitrary linear information for many reproducing kernel Hilbert spaces; a result that has been observed independently in [Geng \& Wang, arXiv:2304.14748].
更多查看译文
AI 理解论文
溯源树
样例
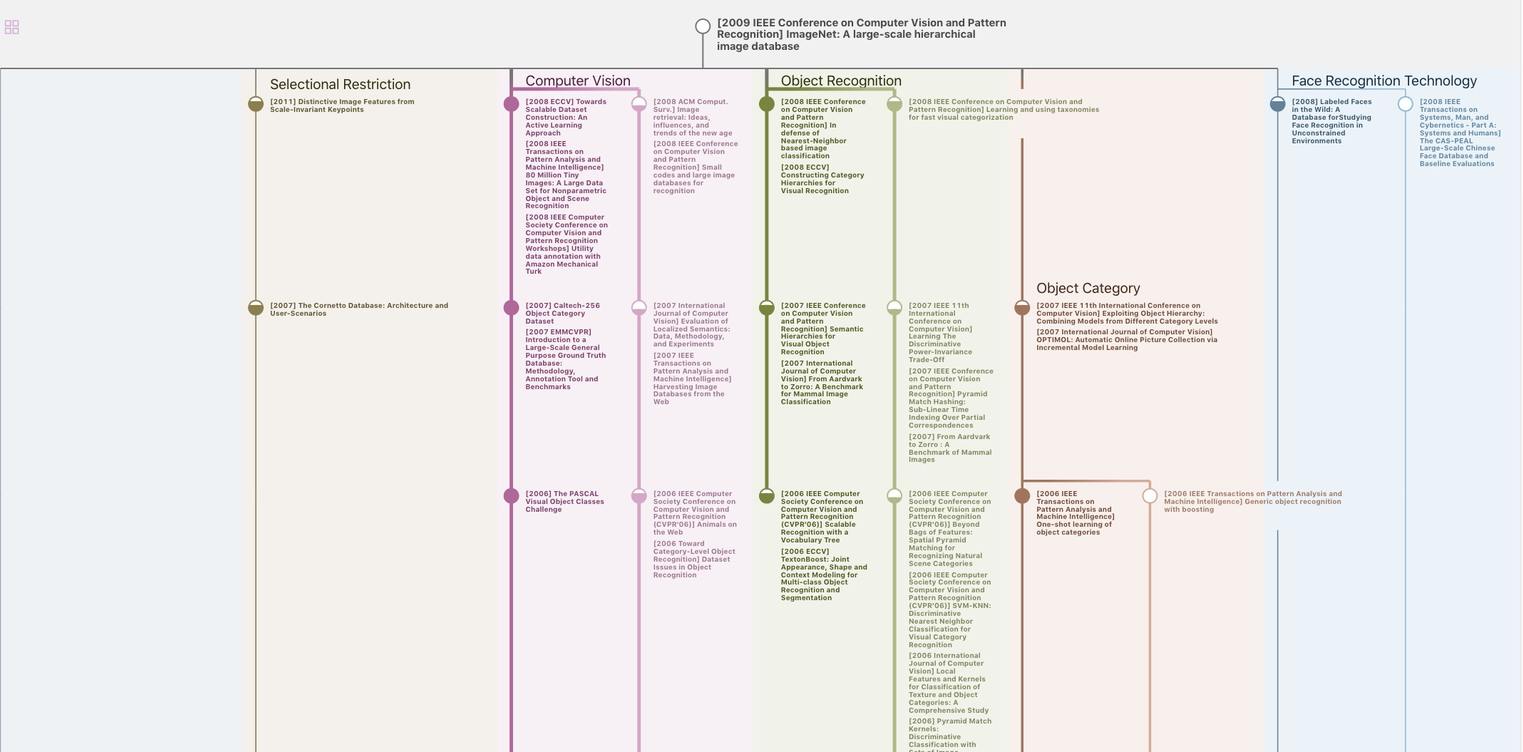
生成溯源树,研究论文发展脉络
Chat Paper
正在生成论文摘要