Analysing River Systems with Time Series Data Using Path Queries in Graph Databases
ISPRS INTERNATIONAL JOURNAL OF GEO-INFORMATION(2023)
摘要
Transportation networks are used in many application areas, like traffic control or river monitoring. For this purpose, sensors are placed in strategic points in the network and they send their data to a central location for storage, viewing and analysis. Recent work proposed graph databases to represent transportation networks, since these networks can change over time, a temporal graph data model is required to keep track of these changes. In this model, time-series data are represented as properties of nodes in the network, and nodes and edges are timestamped with their validity intervals. In this paper, we show that transportation networks can be represented and queried using temporal graph databases and temporal graph query languages. Many interesting situations can be captured by the temporal paths supported by this model. To achieve the above, we extend a recently introduced temporal graph data model and its high-level query language T-GQL to support time series in the nodes of the graph, redefine temporal paths and study and implement new kinds of paths, namely Flow paths and Backwards Flow paths. Further, we analyze a real-world case, using a portion of the Yser river in the Flanders' river system in Belgium, where some nodes are equipped with sensors while other ones are not. We model this river as a temporal graph, implement it using real data provided by the sensors, and discover interesting temporal paths based on the electric conductivity parameter, that can be used in a decision support environment, by experts for analyzing water quality across time.
更多查看译文
关键词
river systems,transportation networks,sensor networks,graph databases,temporal databases,temporal query languages
AI 理解论文
溯源树
样例
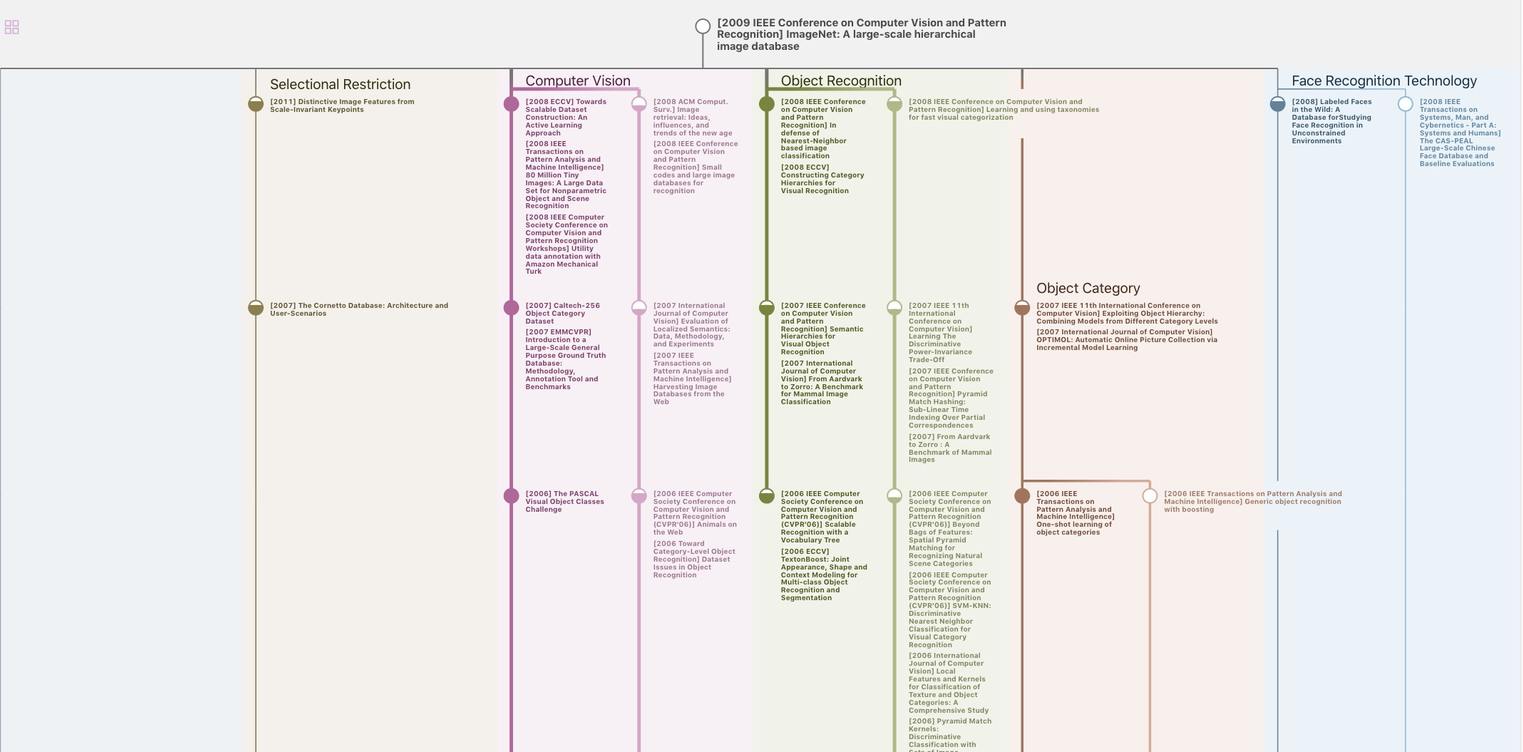
生成溯源树,研究论文发展脉络
Chat Paper
正在生成论文摘要