Efficient Network Representation Learning via Cluster Similarity
DASFAA (3)(2023)
摘要
Network representation learning is a de facto tool for graph analytics. The mainstream of the previous approaches is to factorize the proximity matrix between nodes. However, if n is the number of nodes, since the size of the proximity matrix is n × n , it needs O(n^3) time and O(n^2) space to perform network representation learning; they are significantly high for large-scale graphs. This paper introduces the novel idea of using similarities between clusters instead of proximities between nodes; the proposed approach computes the representations of the clusters from similarities between clusters and computes the representations of nodes by referring to them. If l is the number of clusters, since l ≪ n , we can efficiently obtain the representations of clusters from a small l × l similarity matrix. Furthermore, since nodes in each cluster share similar structural properties, we can effectively compute the representation vectors of nodes. Experiments show that our approach can perform network representation learning more efficiently and effectively than existing approaches.
更多查看译文
关键词
Efficient,Algorithm,Network representation learning,Graph clustering
AI 理解论文
溯源树
样例
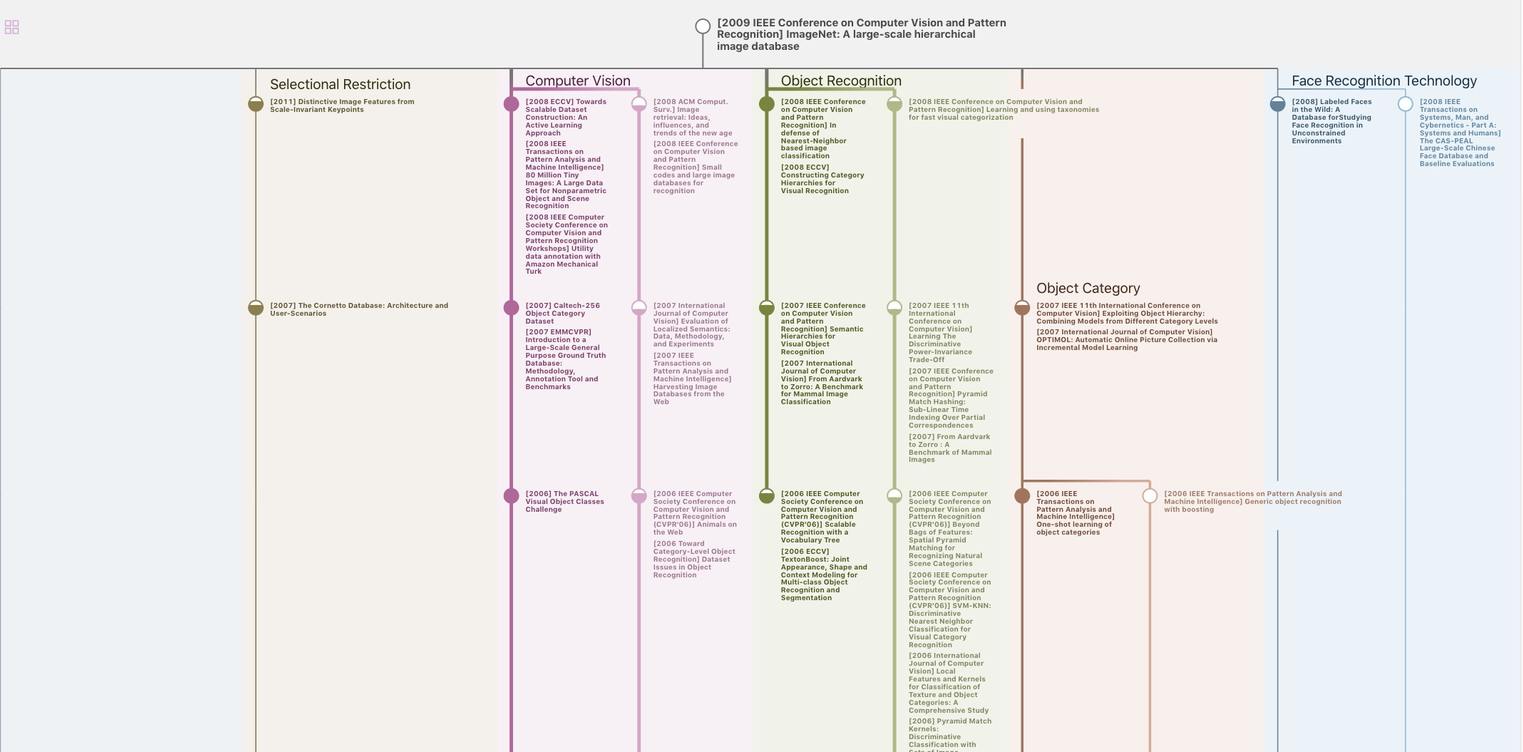
生成溯源树,研究论文发展脉络
Chat Paper
正在生成论文摘要