The StarCraft Multi-Agent Exploration Challenges: Learning Multi-Stage Tasks and Environmental Factors Without Precise Reward Functions.
IEEE Access(2023)
摘要
In this paper, we propose a novel benchmark called the StarCraft Multi-Agent Exploration Challenges(SMAC-Exp), where agents learn to perform multi-stage tasks and to use environmental factors without precise reward functions. The previous challenges (SMAC) recognized as a standard benchmark of Multi-Agent Reinforcement Learning are mainly concerned with ensuring that all agents cooperatively eliminate approaching adversaries only through fine manipulation with obvious reward functions. SMAC-Exp, on the other hand, is interested in the exploration capability of MARL algorithms to efficiently learn implicit multi-stage tasks and environmental factors as well as micro-control. This study covers both offensive and defensive scenarios. In the offensive scenarios, agents must learn to first find opponents and then eliminate them. The defensive scenarios require agents to use topographic features. For example, agents need to position themselves behind protective structures to make it harder for enemies to attack. We investigate a total of twelve MARL algorithms under both sequential and parallel episode settings of SMAC-Exp and observe that recent approaches perform well in similar settings to the previous challenge, but we discover that current multi-agent approaches place relatively less emphasis on exploration perspectives. To a limited extent, we observe that an enhanced exploration method has a positive effect on SMAC-Exp, however, there is still a gap that state-of-the-art algorithms cannot resolve the most challenging scenarios of SMAC-Exp. Consequently, we propose a new axis for future research into Multi-Agent Reinforcement Learning studies.
更多查看译文
关键词
Multi-agent reinforcement learning,exploration,benchmark,StarCraft multi-agent challenge,multi-stage task
AI 理解论文
溯源树
样例
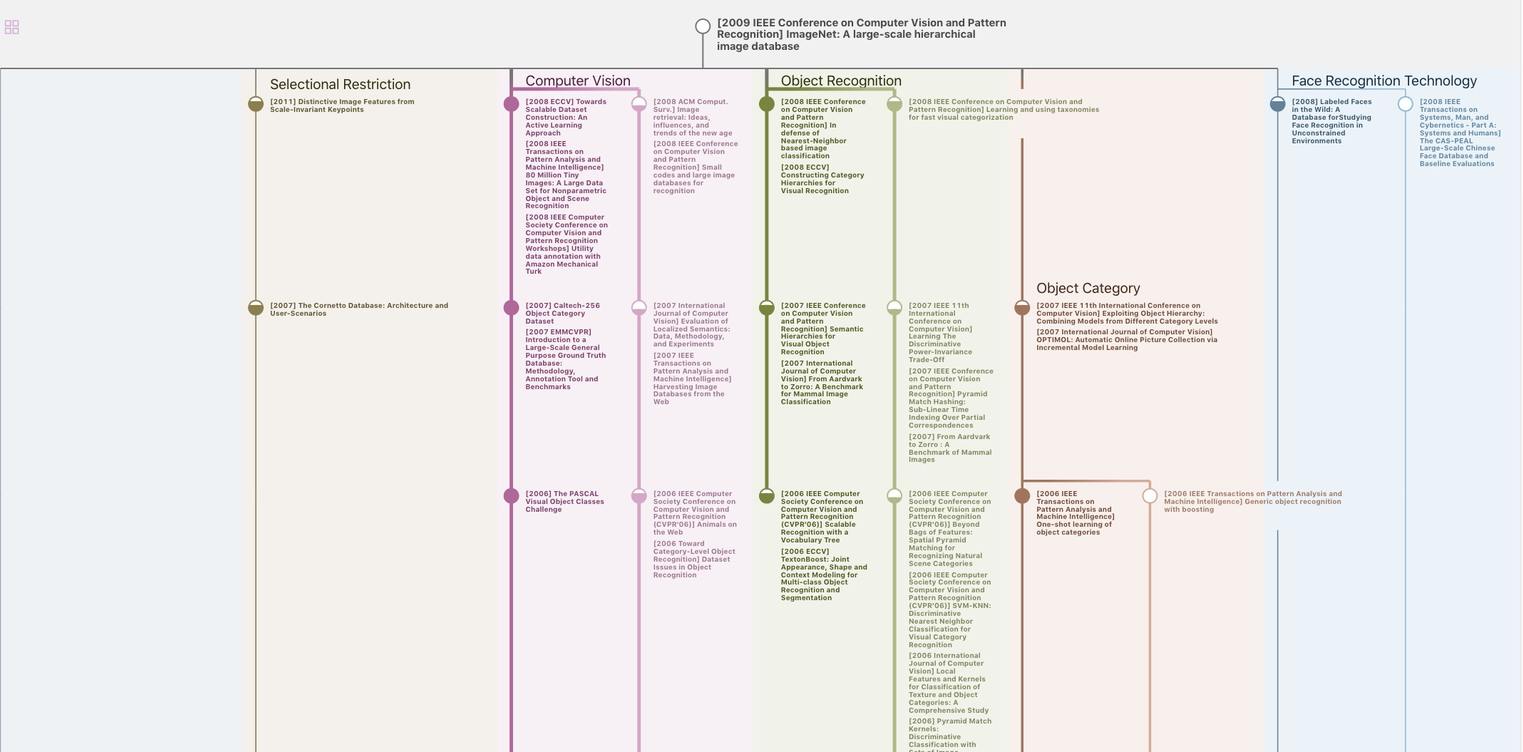
生成溯源树,研究论文发展脉络
Chat Paper
正在生成论文摘要