Multi Layer Peeling for Linear Arrangement and Hierarchical Clustering.
CoRR(2023)
摘要
We present a new multi-layer peeling technique to cluster points in a metric space. A well-known non-parametric objective is to embed the metric space into a simpler structured metric space such as a line (i.e., Linear Arrangement) or a binary tree (i.e., Hierarchical Clustering). Points which are close in the metric space should be mapped to close points/leaves in the line/tree; similarly, points which are far in the metric space should be far in the line or on the tree. In particular we consider the Maximum Linear Arrangement problem \cite{Approximation_algorithms_for_maximum_linear_arrangement} and the Maximum Hierarchical Clustering problem \cite{Hierarchical_Clustering:_Objective_Functions_and_Algorithms} applied to metrics. We design approximation schemes ($1 - \epsilon$ approximation for any constant $\epsilon > 0$) for these objectives. In particular this shows that by considering metrics one may significantly improve former approximations ($0.5$ for Max Linear Arrangement and $0.74$ for Max Hierarchical Clustering). Our main technique, which is called multi-layer peeling, consists of recursively peeling off points which are far from the "core" of the metric space. The recursion ends once the core becomes a sufficiently densely weighted metric space (i.e. the average distance is at least a constant times the diameter) or once it becomes negligible with respect to its inner contribution to the objective. Interestingly, the algorithm in the Linear Arrangement case is much more involved than that in the Hierarchical Clustering case, and uses a significantly more delicate peeling.
更多查看译文
关键词
clustering,linear arrangement,multi layer
AI 理解论文
溯源树
样例
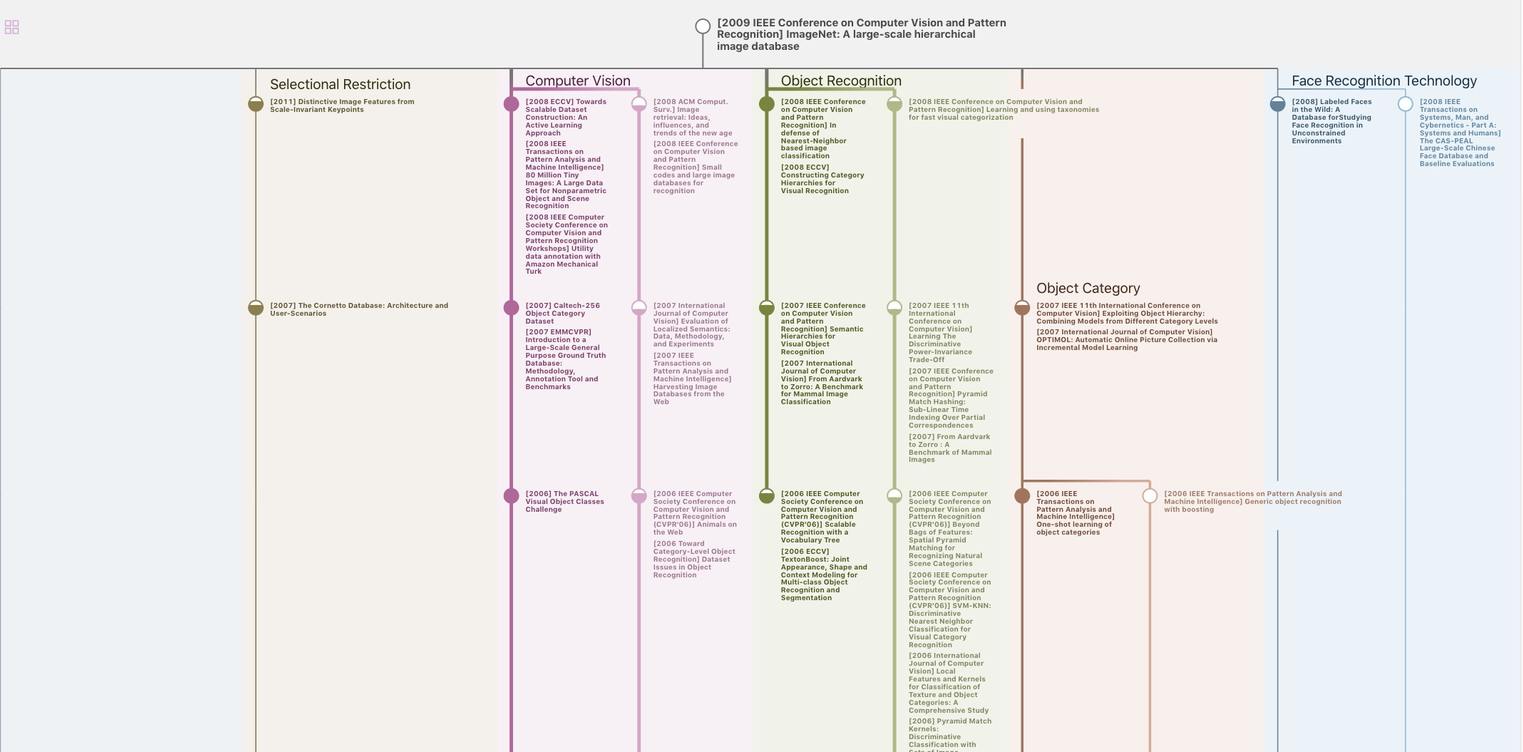
生成溯源树,研究论文发展脉络
Chat Paper
正在生成论文摘要