Beyond Fine-Tuning: Efficient and Effective Fed-Tuning for Mobile/Web Users
WWW 2023(2023)
摘要
Fine-tuning is a typical mechanism to achieve model adaptation for mobile/web users, where a model trained by the cloud is further retrained to fit the target user task. While traditional fine-tuning has been proved effective, it only utilizes local data to achieve adaptation, failing to take advantage of the valuable knowledge from other mobile/web users. In this paper, we attempt to extend the local-user fine-tuning to multi-user fed-tuning with the help of Federated Learning (FL). Following the new paradigm, we propose EEFT, a framework aiming to achieve Efficient and Effective Fed-Tuning for mobile/web users. The key idea is to introduce lightweight but effective adaptation modules to the pre-trained model, such that we can freeze the pre-trained model and just focus on optimizing the modules to achieve cost reduction and selective task cooperation. Extensive experiments on our constructed benchmark demonstrate the effectiveness and efficiency of the proposed framework.
更多查看译文
AI 理解论文
溯源树
样例
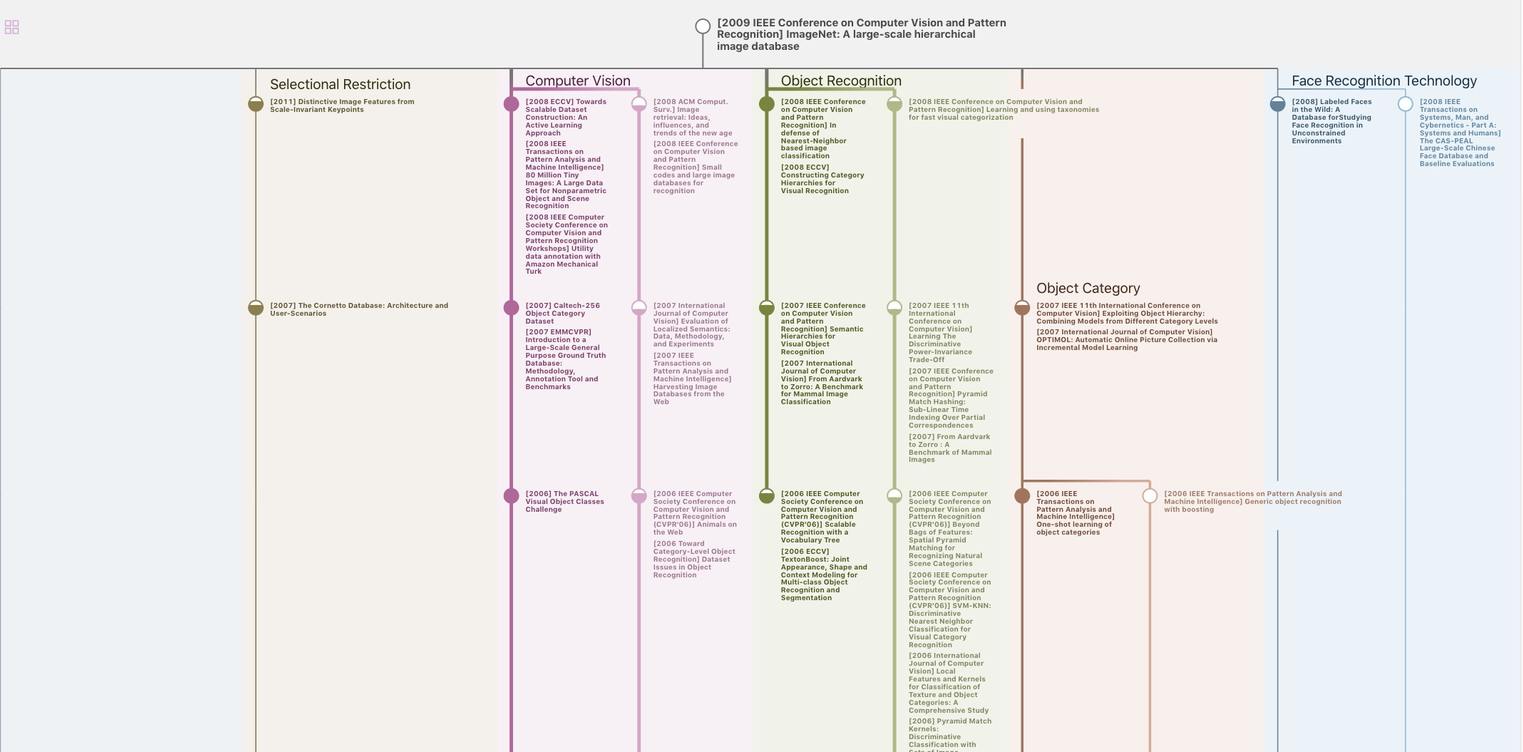
生成溯源树,研究论文发展脉络
Chat Paper
正在生成论文摘要