Channel Shuffle Neural Architecture Search for Key Word Spotting
IEEE Signal Processing Letters(2023)
摘要
The evolution of Network Architecture (NA) allowed Key-Word Spotting (KWS) to exhibit high performance. Generally, NA for KWS is required to have low parameter and computation complexity maintaining high classification performance. Most of the attempts so far have been based on manual approaches, and often the architectures developed from such efforts dwell in the balance of the performance and the network complexity. Then, several KWS models based on Neural Architecture Search (NAS) technique have been proposed. However, these methods do not consider the number of parameters and FLOPs for NA in the search process and manually adjusted the complexity of NA by reducing the number of cells. It may not produce optimized NA with a balance between network complexity and performance. To develop effective network architecture for KWS, network complexity and performance must be considered. In this letter, we propose Channel Shuffle Neural Architecture Search (CSNAS) with channel weights. CSNAS selects whether each channel of the input feature is reflected in the computation or not in the search process and simultaneously controls the number of parameters, FLOPs, and performance. Experiment results show that CSNAS can generate NA that satisfies complexity and performance conditions, and NAs generated by CSNAS outperform state-of-the-art KWS methods.
更多查看译文
关键词
Keyword spotting,neural architecture search
AI 理解论文
溯源树
样例
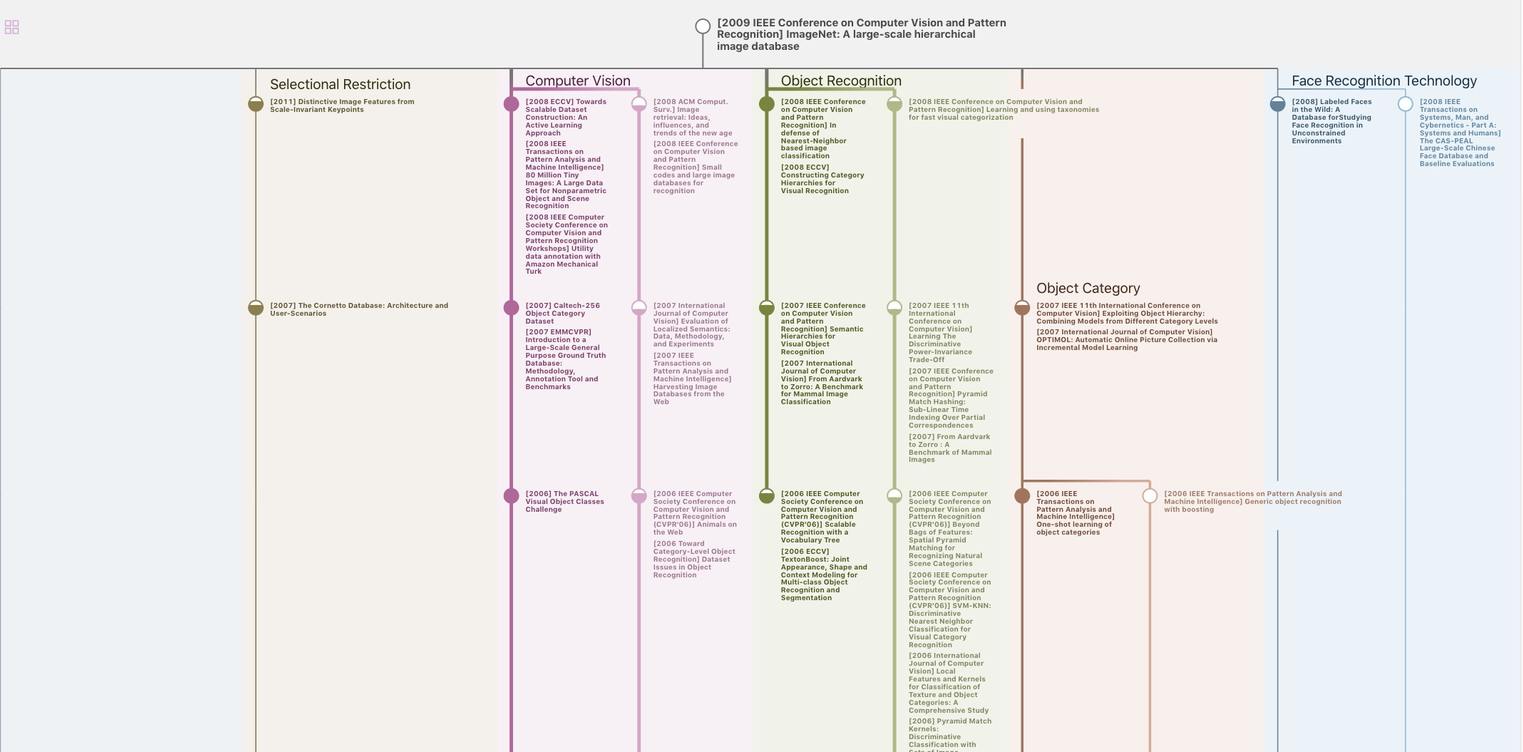
生成溯源树,研究论文发展脉络
Chat Paper
正在生成论文摘要