A Theory on Adam Instability in Large-Scale Machine Learning
CoRR(2023)
摘要
We present a theory for the previously unexplained divergent behavior noticed in the training of large language models. We argue that the phenomenon is an artifact of the dominant optimization algorithm used for training, called Adam. We observe that Adam can enter a state in which the parameter update vector has a relatively large norm and is essentially uncorrelated with the direction of descent on the training loss landscape, leading to divergence. This artifact is more likely to be observed in the training of a deep model with a large batch size, which is the typical setting of large-scale language model training. To argue the theory, we present observations from the training runs of the language models of different scales: 7 billion, 30 billion, 65 billion, and 546 billion parameters.
更多查看译文
关键词
adam instability,machine learning,large-scale
AI 理解论文
溯源树
样例
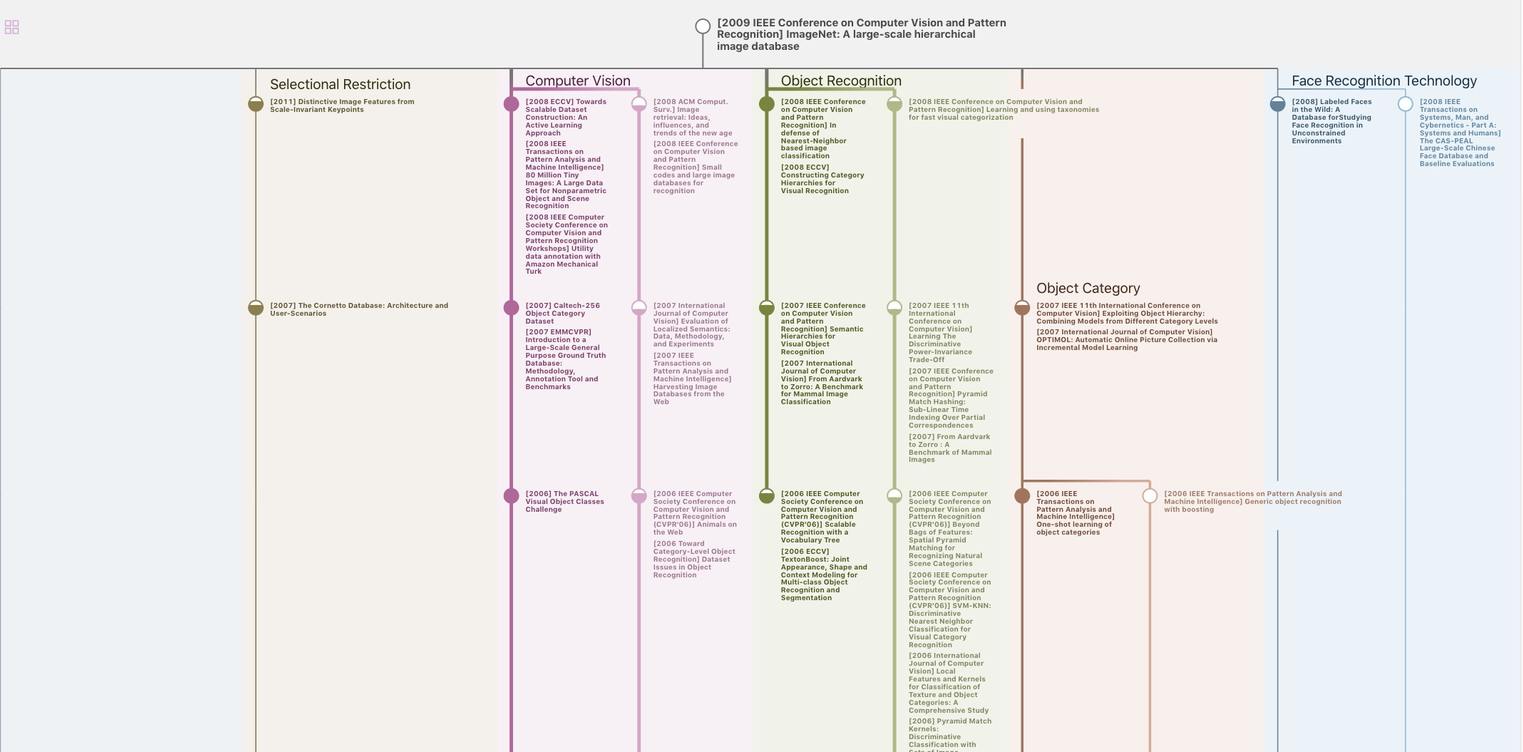
生成溯源树,研究论文发展脉络
Chat Paper
正在生成论文摘要