Self-Supervised Learning With Cluster-Aware-DINO for High-Performance Robust Speaker Verification
IEEE-ACM TRANSACTIONS ON AUDIO SPEECH AND LANGUAGE PROCESSING(2024)
摘要
The automatic speaker verification task has achieved great success using deep learning approaches with a large-scale, manually annotated dataset. However, collecting a significant amount of well-labeled data for system building is very difficult and expensive. Recently, self-supervised speaker verification has attracted a lot of interest due to its no dependency on labeled data. In this article, we propose a novel and advanced self-supervised learning framework based on our prior work, which can construct a powerful speaker verification system with high performance without using any labeled data. To avoid the impact of false negative pairs, we adopt the self-distillation with no labels (DINO) framework as the initial model, which can be trained without exploiting negative pairs. Then, we further introduce a cluster-aware training strategy for DINO to improve the diversity of data. In the iterative learning stage, due to a mass of unreliable labels from unsupervised clustering, the quality of pseudo labels is important for the system performance. This motivates us to propose dynamic loss-gate and label correction (DLG-LC) methods to alleviate the performance degradation caused by unreliable labels. Furthermore, we extend the DLG-LC from single-modality to multi-modality on the audio-visual dataset to further improve the performance. The experiments were conducted using the widely-used Voxceleb dataset. Compared to the best-known self-supervised speaker verification system, our proposed method achieve relative EER improvement of 22.17%, 27.94% and 25.56% on Vox-O, Vox-E and Vox-H test sets, even with fewer iterations, smaller models, and simpler clustering methods. Importantly, the newly proposed self-supervised learning system even achieves comparable results with the fully supervised system, but without using any human-labeled data.
更多查看译文
关键词
Self-supervised speaker verification,cluster-aware dino,dynamic loss-gate,label correction,multi-modality
AI 理解论文
溯源树
样例
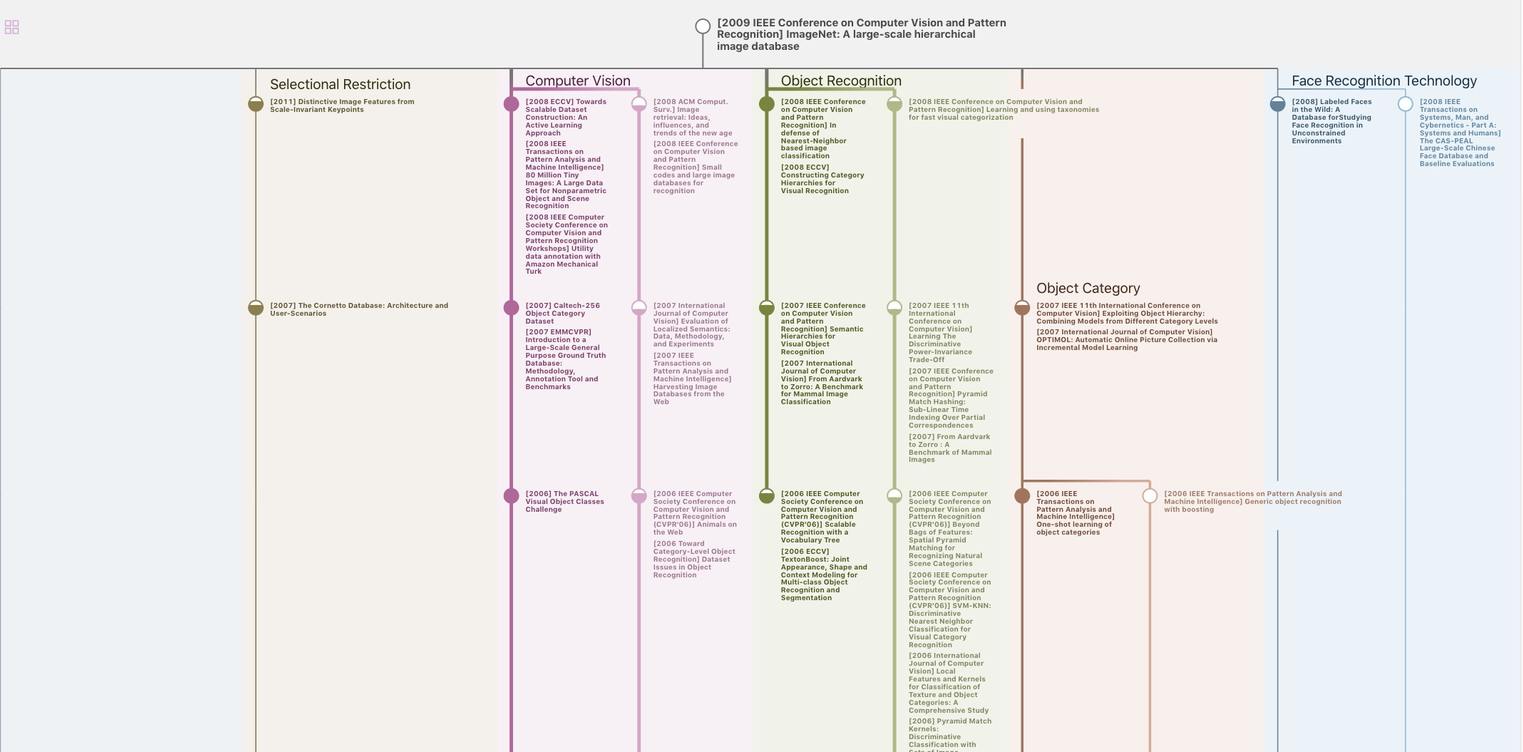
生成溯源树,研究论文发展脉络
Chat Paper
正在生成论文摘要