Examining Temporalities on Stance Detection Towards COVID-19 Vaccination
arXiv (Cornell University)(2023)
摘要
Previous studies have highlighted the importance of vaccination as an effective strategy to control the transmission of the COVID-19 virus. It is crucial for policymakers to have a comprehensive understanding of the public's stance towards vaccination on a large scale. However, attitudes towards COVID-19 vaccination, such as pro-vaccine or vaccine hesitancy, have evolved over time on social media. Thus, it is necessary to account for possible temporal shifts when analysing these stances. This study aims to examine the impact of temporal concept drift on stance detection towards COVID-19 vaccination on Twitter. To this end, we evaluate a range of transformer-based models using chronological and random splits of social media data. Our findings demonstrate significant discrepancies in model performance when comparing random and chronological splits across all monolingual and multilingual datasets. Chronological splits significantly reduce the accuracy of stance classification. Therefore, real-world stance detection approaches need to be further refined to incorporate temporal factors as a key consideration.
更多查看译文
关键词
stance detection,vaccination
AI 理解论文
溯源树
样例
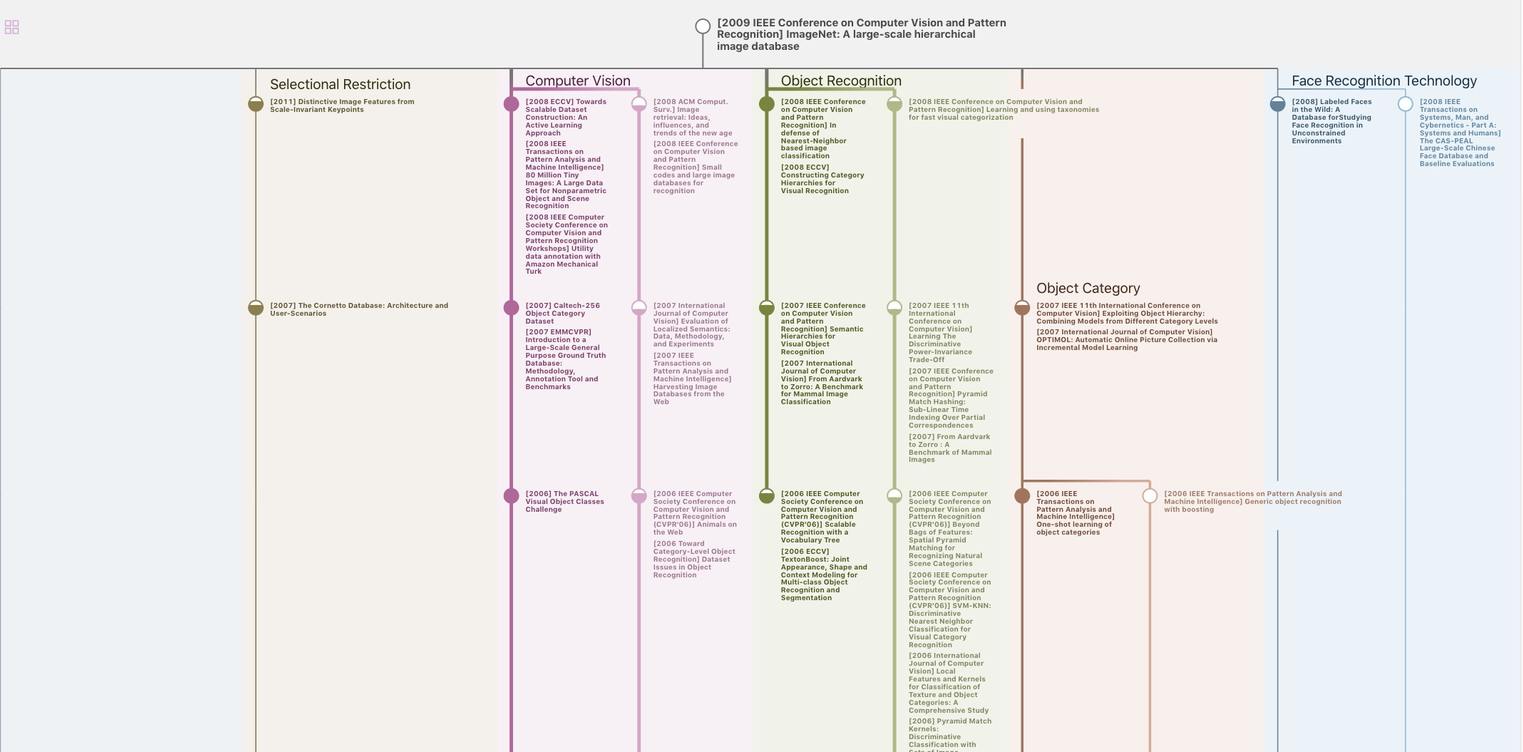
生成溯源树,研究论文发展脉络
Chat Paper
正在生成论文摘要