IEDR: A Context-aware Intrinsic and Extrinsic Disentangled Recommender System
ICLR 2023(2023)
摘要
Intrinsic and extrinsic factors jointly affect users' decisions in item selection (e.g., click, purchase). Intrinsic factors reveal users' real interests and are invariant in different contexts (e.g., time, weather), whereas extrinsic factors can change w.r.t. different contexts. Analyzing these two factors is an essential yet challenging task in recommender systems. However, in existing studies, factor analysis is either largely neglected, or designed for a specific context (e.g., the time context in sequential recommendation), which limits the applicability of such models. In this paper, we propose a generic model, IEDR, to learn intrinsic and extrinsic factors from various contexts for recommendation. IEDR contains two key components: a contrastive learning component, and a disentangling component. The two components collaboratively enable our model to learn context-invariant intrinsic factors and context-based extrinsic factors from all available contexts. Experimental results on real-world datasets demonstrate the effectiveness of our model in factor learning and impart a significant improvement in recommendation accuracy over the state-of-the-art methods.
更多查看译文
关键词
Recommender Systems,Intrinsic and Extrinsic Factors,Contrastive Learning,Disentangled Representation,Mutual Information
AI 理解论文
溯源树
样例
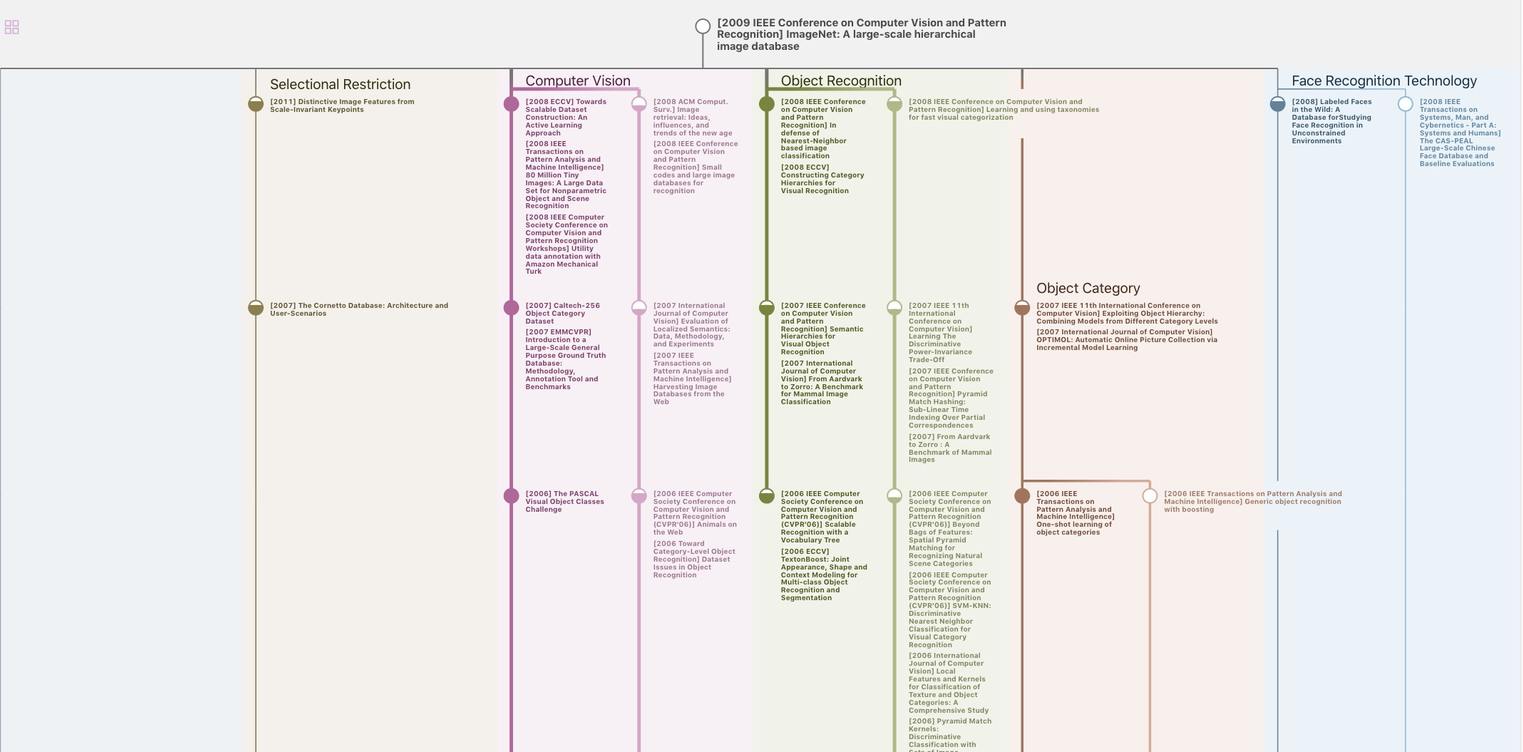
生成溯源树,研究论文发展脉络
Chat Paper
正在生成论文摘要