T2D: Spatiotemporal Feature Learning Based on Triple 2D Decomposition
ICLR 2023(2023)
摘要
In this paper, we propose triple 2D decomposition (T2D) of a 3D vision Transformer (ViT) for efficient spatiotemporal feature learning. The idea is to divide the input 3D video data into three 2D data planes and use three 2D filters, implemented by 2D ViT, to extract spatial and motion features. Such a design not only effectively reduces the computational complexity of a 3D ViT, but also guides the network to focus on learning correlations among more relevant tokens. Compared with other decomposition methods, the proposed T2D is shown to be more powerful at a similar computational complexity. The CLIP-initialized T2D-B model achieves state-of-the-art top-1 accuracy of 85.0% and 70.5% on Kinetics-400 and Something-Something-v2 datasets, respectively. It also outperforms other methods by a large margin on FineGym (+17.9%) and Diving-48 (+1.3%) datasets. Under the zero-shot setting, the T2D model obtains a 2.5% top-1 accuracy gain over X-CLIP on HMDB-51 dataset. In addition, T2D is a general decomposition method that can be plugged into any ViT structure of any model size. We demonstrate this by building a tiny size of T2D model based on a hierarchical ViT structure named DaViT. The resulting DaViT-T2D-T model achieves 82.0\% and 71.3\% top-1 accuracy with only 91 GFLOPs on Kinectics-400 and Something-Something-v2 datasets, respectively. Source code will be made publicly available.
更多查看译文
关键词
spatiotemporal feature learning,video recognition,action recognition,video Transformer
AI 理解论文
溯源树
样例
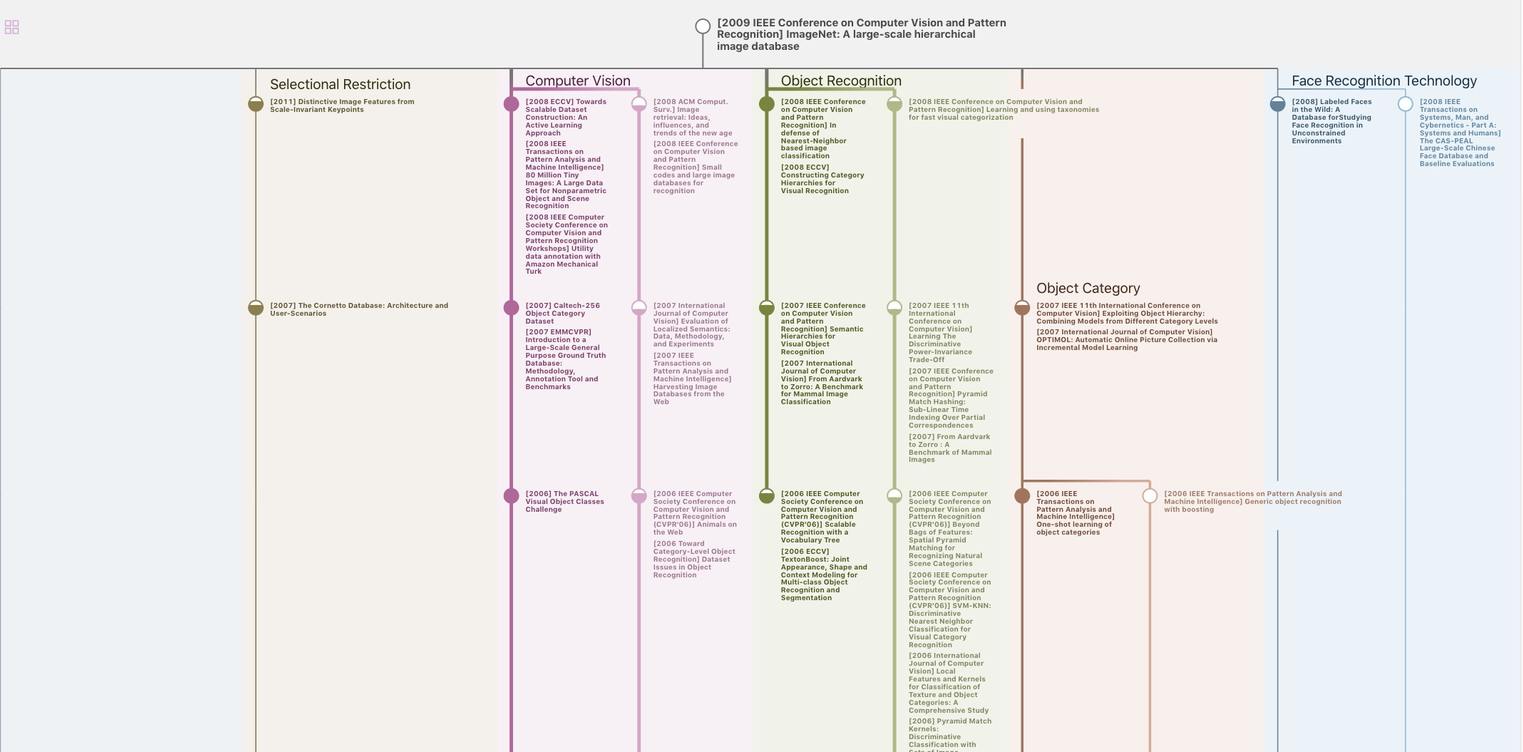
生成溯源树,研究论文发展脉络
Chat Paper
正在生成论文摘要