Beyond Single Path Integrated Gradients for Reliable Input Attribution via Randomized Path Sampling.
ICCV(2023)
摘要
Input attribution is a widely used explanation method for deep neural networks, especially in visual tasks. Among various attribution methods, Integrated Gradients (IG) [28] is frequently used because of its model-agnostic applicability and desirable axioms. However, previous work [24], [8], [9] has shown that such method often produces noisy and unreliable attributions during the integration of the gradients over the path defined in the input space. In this paper, we tackle this issue by estimating the distribution of the possible attributions according to the integrating path selection. We show that such noisy attribution can be reduced by aggregating attributions from the multiple paths instead of using a single path. Inspired by Stick-Breaking Process [20], we suggest a random process to generate rich and various sampling of the gradient integrating path. Using multiple input attributions obtained from randomized path, we propose a novel attribution measure using the distribution of attributions at each input features. We identify proposed method qualitatively show less-noisy and object-aligned attribution and its feasibility through the quantitative evaluations.
更多查看译文
AI 理解论文
溯源树
样例
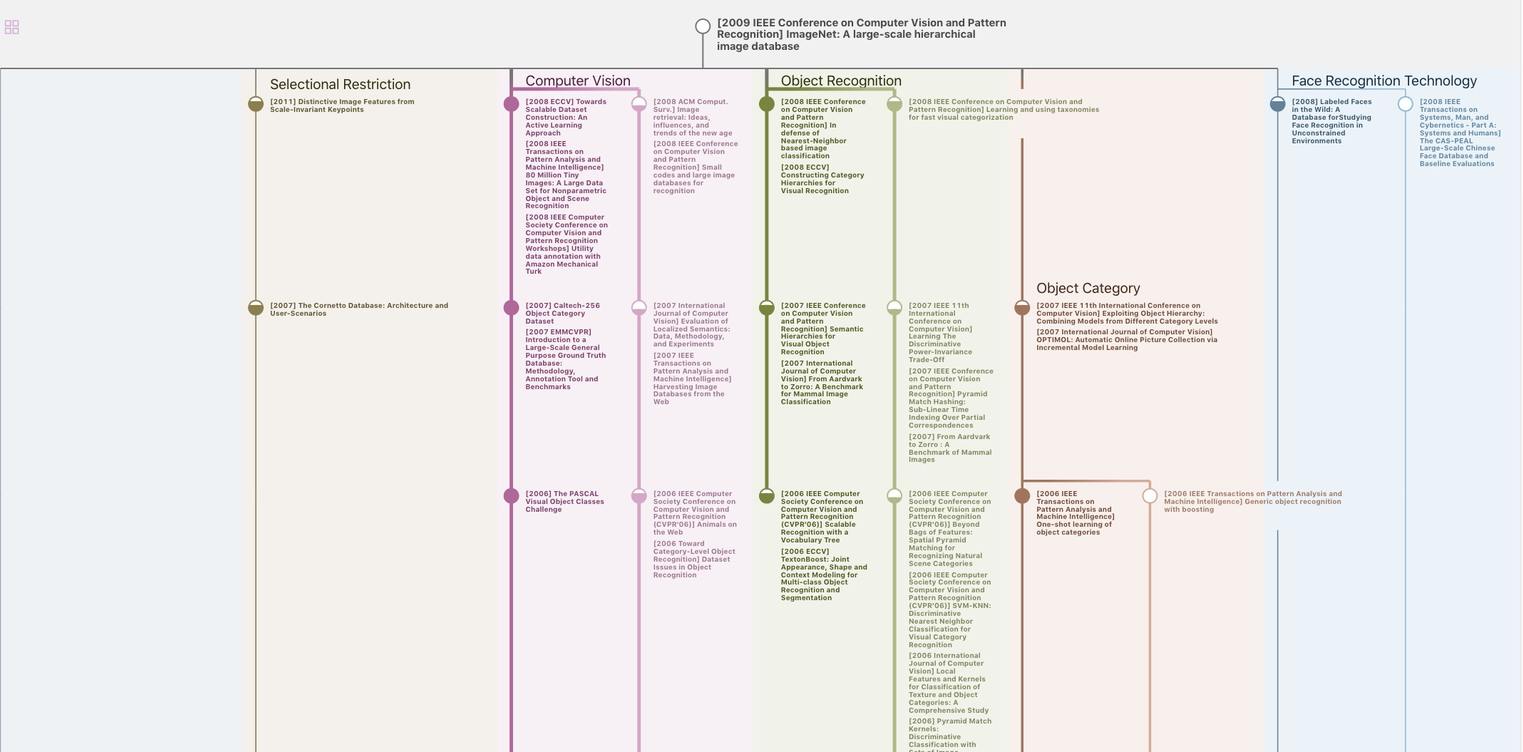
生成溯源树,研究论文发展脉络
Chat Paper
正在生成论文摘要