Learning Deep Operator Networks: The Benefits of Over-Parameterization
ICLR 2023(2023)
摘要
Neural Operators that directly learn mappings between function spaces have received considerable recent attention. Deep Operator Networks (DeepONets), a popular recent class of neural operators have shown promising preliminary results in approximating solution operators of parametric differential equations. Despite the universal approximation guarantees, there is yet no optimization convergence guarantee for DeepONets based on gradient descent (GD). In this paper, we establish such guarantees and show that over-parameterization based on wide layers provably helps. In particular, we present two types of optimization convergence analysis: first, for smooth activations, we bound the spectral norm of the Hessian of DeepONets and use the bound to show geometric convergence of GD based on restricted strong convexity (RSC); and second, for ReLU activations, we show the neural tangent kernel (NTK) of DeepONets at initialization is positive definite, which can be used with the standard NTK analysis to imply geometric convergence. Further, we present empirical results on three canonical operator learning problems: Antiderivative, Diffusion-Reaction equation, and Burger’s equation, and show that wider DeepONets lead to lower training loss on all the problems, thereby supporting the theoretical results
更多查看译文
关键词
Deep Operator Networks,Optimization,Neural Tangent Kernel
AI 理解论文
溯源树
样例
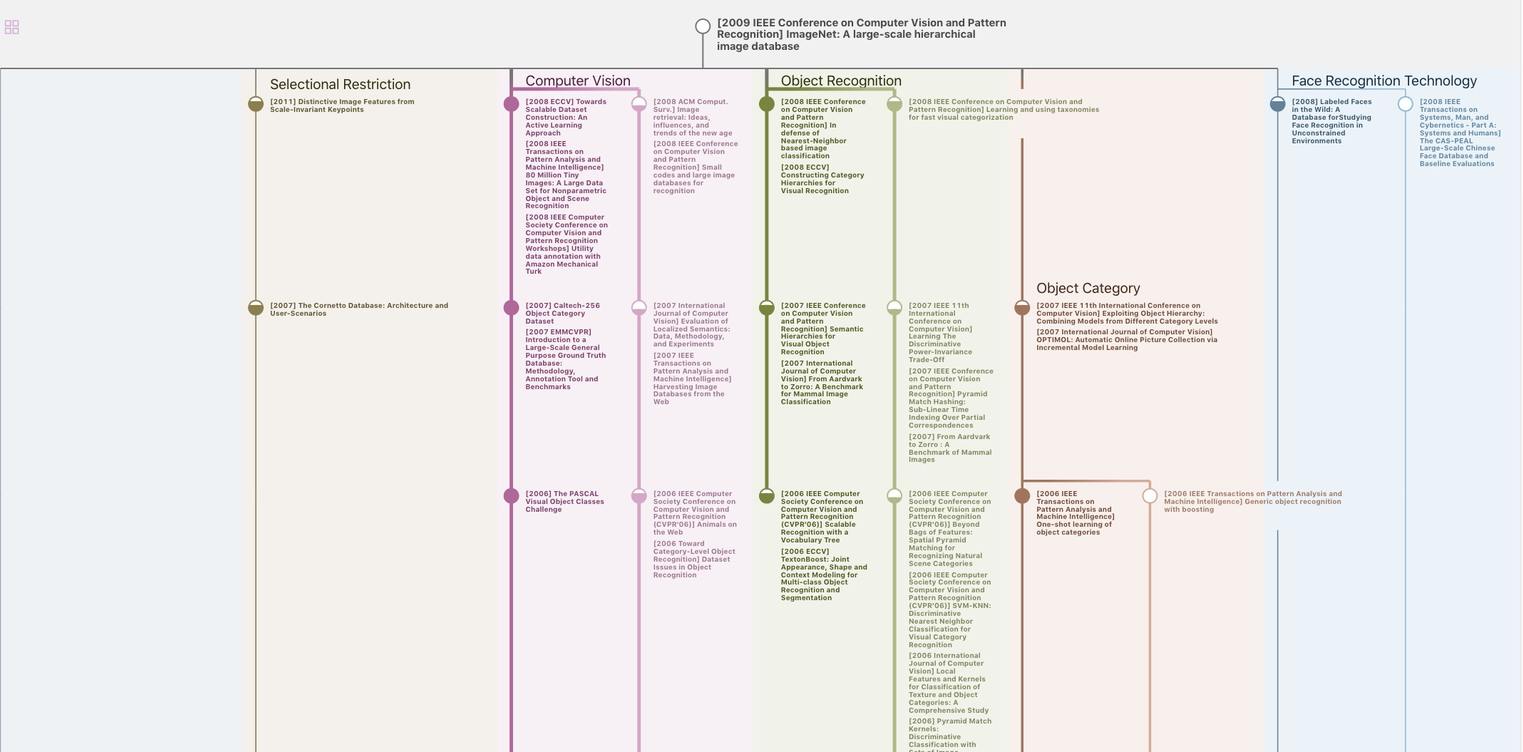
生成溯源树,研究论文发展脉络
Chat Paper
正在生成论文摘要