Moment Distributionally Robust Probabilistic Supervised Learning
ICLR 2023(2023)
摘要
Probabilistic supervised learning assumes the groundtruth itself is a distribution instead of a single label, as in classic settings. Common approaches learn with a proper composite loss and obtain probability estimates via an invertible link function. Typical links such as the softmax yield restrictive and problematic uncertainty certificates. In this paper, we propose to make direct prediction of conditional label distributions from first principles in distributionally robust optimization based on an ambiguity set defined by feature moment divergence. We derive its generalization bounds under mild assumptions. We illustrate how to manipulate penalties for underestimation and overestimation. Our method can be easily incorporated into neural networks for end-to-end representation learning. Experimental results on datasets with probabilistic labels illustrate the flexibility, effectiveness, and efficiency of this learning paradigm.
更多查看译文
关键词
probabilistic supervised learning,distributionally robust optimization,proper scoring rules
AI 理解论文
溯源树
样例
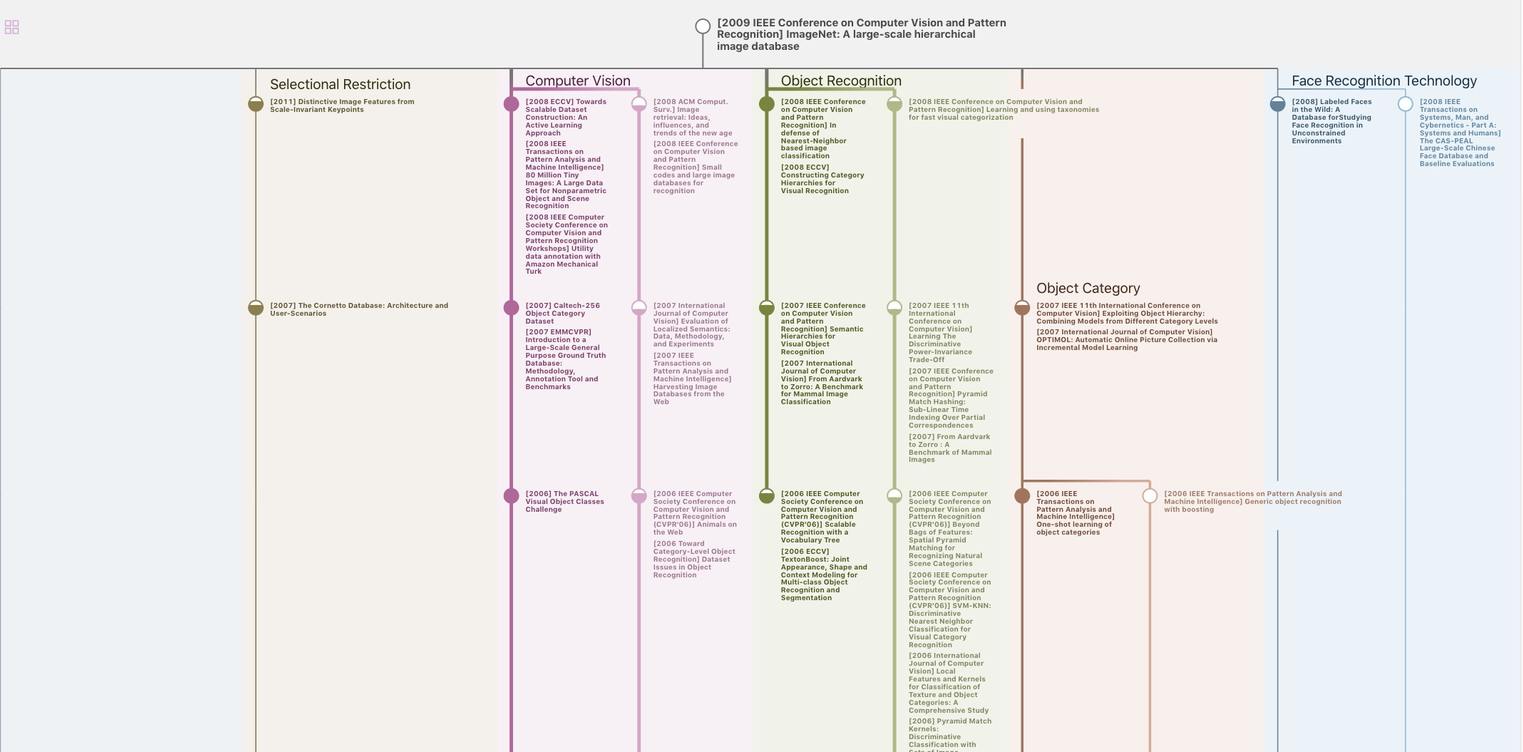
生成溯源树,研究论文发展脉络
Chat Paper
正在生成论文摘要