How (Un)Fair is Text Summarization?
ICLR 2023(2023)
摘要
Creating a good summary requires carefully choosing details from the original text to accurately represent it in a limited space. If a summary contains biased information about a group, it risks passing this bias off to readers as fact. These risks increase if we consider not just one biased summary, but rather a biased summarization algorithm. Despite this, little work has measured whether these summarizers demonstrate biased performance. Rather, most work in summarization focuses on improving performance, ignoring questions of bias. In this paper we demonstrate that automatic summarizers both amplify and introduce bias towards information about under-represented groups. Additionally, we show that summarizers are highly sensitive to document structure, making the summaries they generate unstable under changes that are semantically meaningless to humans, which poses a further fairness risk. Given these results, and the large scale potential for harm presented by biased summarization, we recommend that bias analysis be performed and reported on summarizers to ensure that new automatic summarization methods do not introduce bias to the summaries they generate.
更多查看译文
关键词
Natural language processing,Summarization,Fairness
AI 理解论文
溯源树
样例
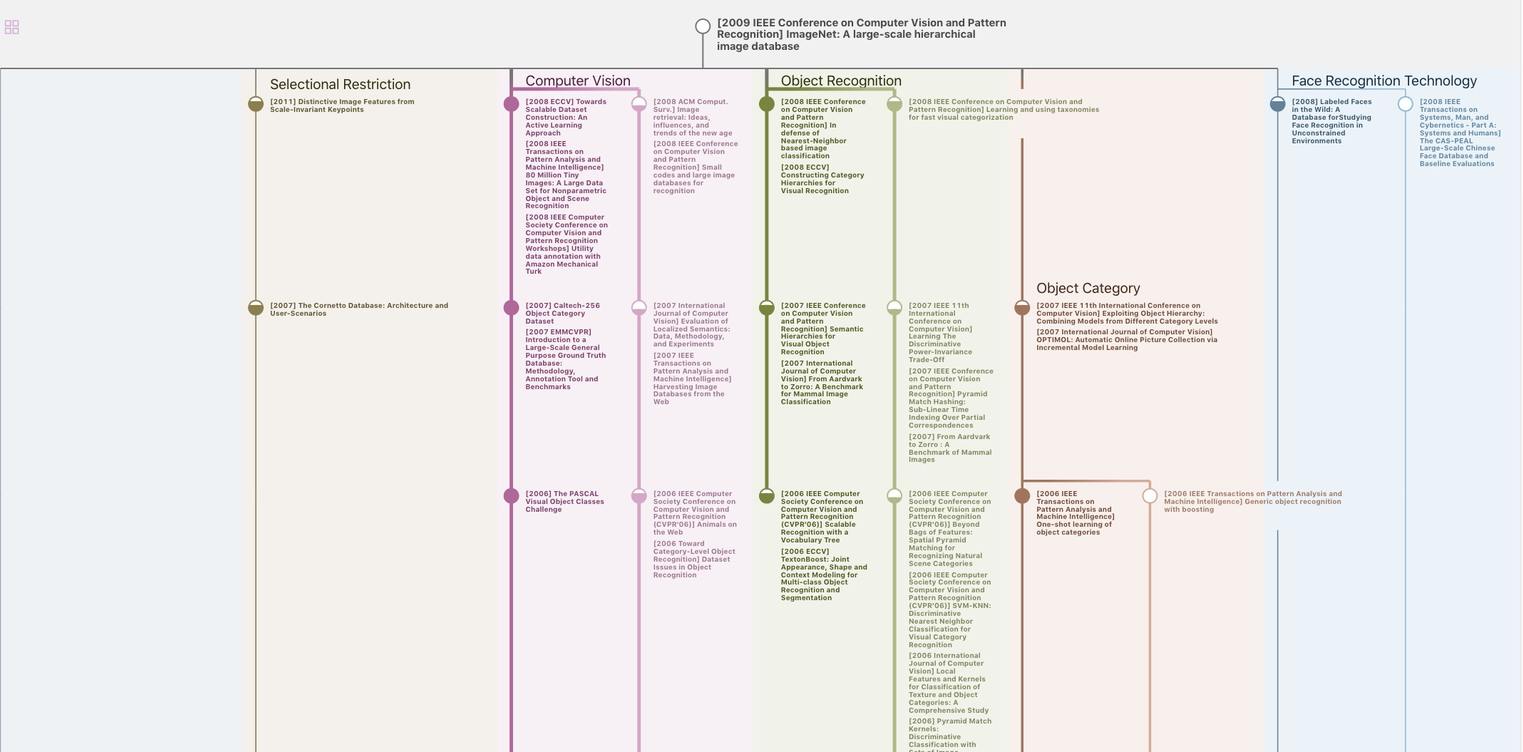
生成溯源树,研究论文发展脉络
Chat Paper
正在生成论文摘要