HGBER: Heterogeneous Graph Neural Network With Bidirectional Encoding Representation.
IEEE transactions on neural networks and learning systems(2023)
摘要
Heterogeneous graphs with multiple types of nodes and link relationships are ubiquitous in many real-world applications. Heterogeneous graph neural networks (HGNNs) as an efficient technique have shown superior capacity of dealing with heterogeneous graphs. Existing HGNNs usually define multiple meta-paths in a heterogeneous graph to capture the composite relations and guide neighbor selection. However, these models only consider the simple relationships (i.e., concatenation or linear superposition) between different meta-paths, ignoring more general or complex relationships. In this article, we propose a novel unsupervised framework termed Heterogeneous Graph neural network with bidirectional encoding representation (HGBER) to learn comprehensive node representations. Specifically, the contrastive forward encoding is firstly performed to extract node representations on a set of meta-specific graphs corresponding to meta-paths. We then introduce the reversed encoding for the degradation process from the final node representations to each single meta-specific node representations. Moreover, to learn structure-preserving node representations, we further utilize a self-training module to discover the optimal node distribution through iterative optimization. Extensive experiments on five open public datasets show that the proposed HGBER model outperforms the state-of-the-art HGNNs baselines by 0.8%-8.4% in terms of accuracy on most datasets in various downstream tasks.
更多查看译文
关键词
Bidirectional encoding representation,heterogeneous graph neural network,heterogeneous graph representation learning,multiple meta-paths
AI 理解论文
溯源树
样例
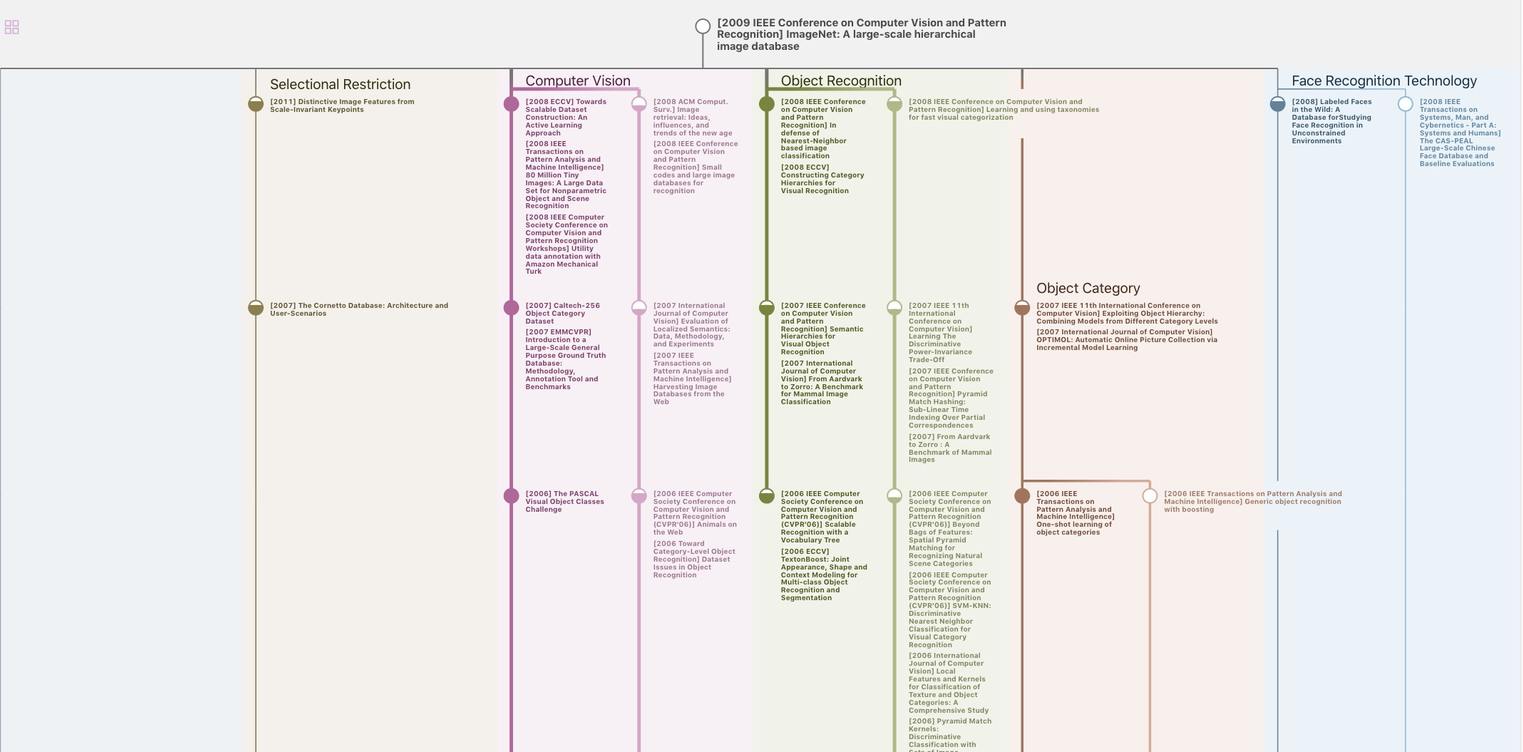
生成溯源树,研究论文发展脉络
Chat Paper
正在生成论文摘要