Prediction of Leakage Rate and Optimization of Structural Parameter of Blade Tip Labyrinth Seal
CHIANG MAI JOURNAL OF SCIENCE(2023)
摘要
To study the influence of the structural parameters of blade tip labyrinth seal (BTLS) on leakage flow characteristics, finite element method was used to calculate the relationship between blade tip leakage rate (BTLR) and four structural parameters such as tooth width, tooth height, tooth pitch and tooth number. With the finite element results as samples, support vector regression (SVR), back propagation (BP) neural network and extreme learning machine (ELM) were used to establish the prediction model of the relationship between BTLR and four structural parameters. The accuracy and applicability of three prediction models were compared and analyzed. The results showed that SVR algorithm has higher prediction accuracy and stability compared with other algorithms for the prediction of BTLR. The mean square error and determination coefficient of its test set are 0.00059637 and 0.99253 respectively. After that, SVR results were taken as samples of genetic algorithm to find the combination of structural parameters with the minimum BTLR. The obtained structural parameters were combined for simulation modeling calculation. Its results showed that the fluid velocity in the blade tip region is significantly reduced and the velocity transition is gentle. The difference between simulation and optimization was 0.01%. This method innovatively applies machine learning algorithm to the prediction of BTLR, and improves the problem of low speed and high cost when only using finite element method. It provides a new way to calculate BTLR. In addition, the structural parameters of BTLS are optimized to reduce BTLR. This idea expands the field of application of machine learning algorithms.
更多查看译文
关键词
blade tip leakage rate,prediction and optimization,support vector regression,back propagation neural network,extreme learning machine,genetic algorithm
AI 理解论文
溯源树
样例
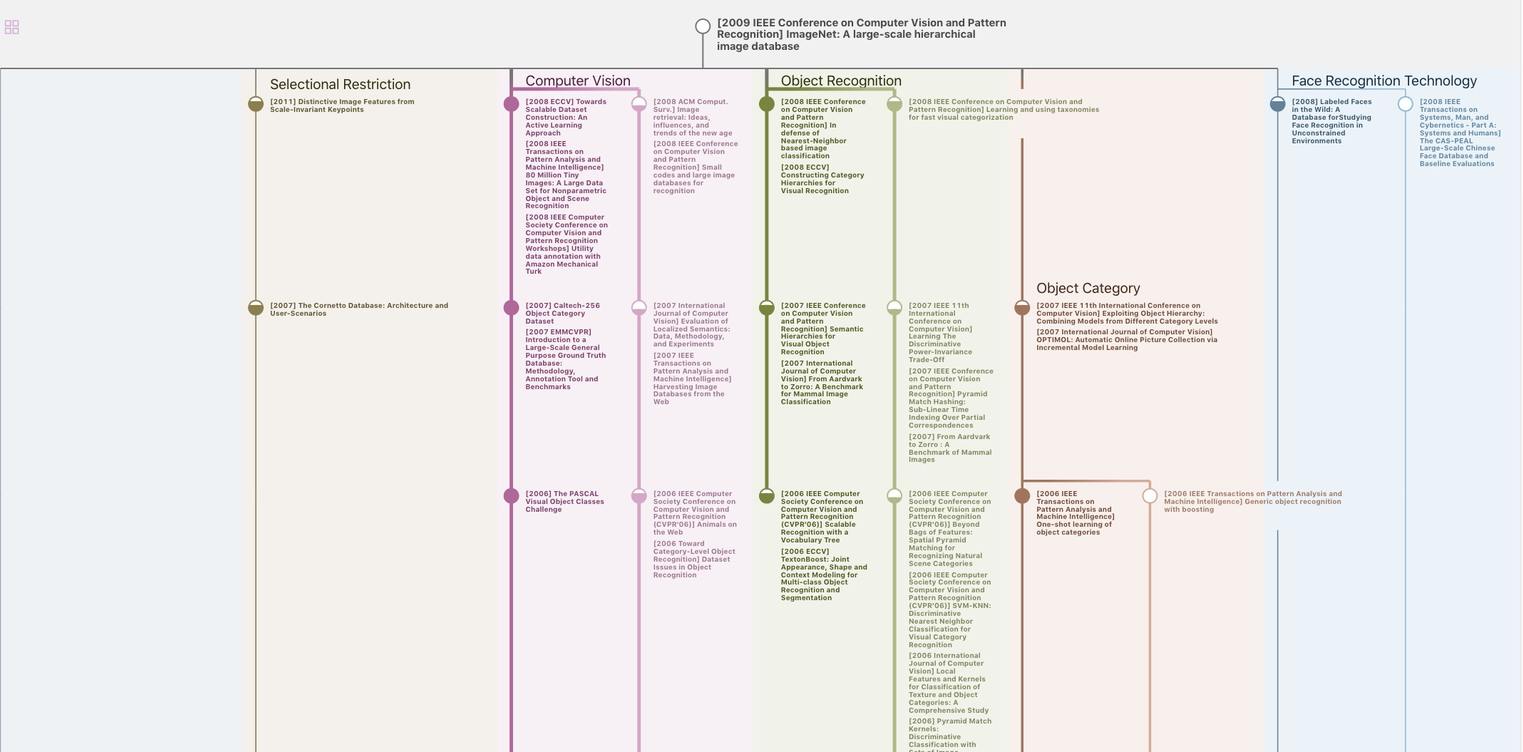
生成溯源树,研究论文发展脉络
Chat Paper
正在生成论文摘要